What role does LiDAR annotation play in 3D mapping?
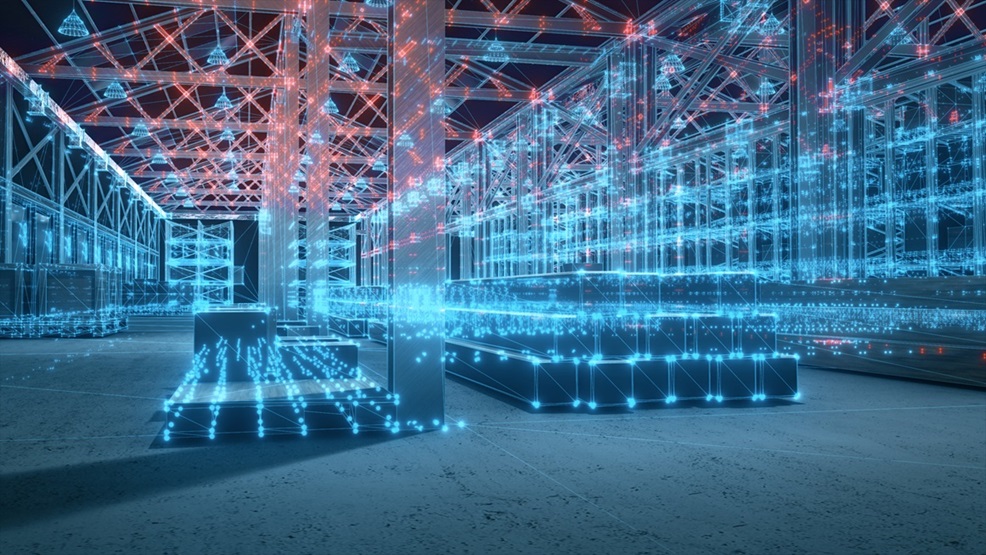
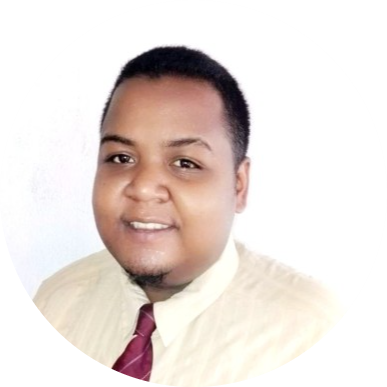
Have you ever wondered how autonomous vehicles navigate and understand their environment? The answer lies in 3D mapping and localization: LiDAR annotation plays a key role in making this possible. LiDAR, for "Light Detection and Ranging", is a remote sensing technology that uses laser beams to generate high-resolution 3D maps of the environment.
How does LiDAR annotation fit into this context? That's what we aim to explore in this article, in which we present the role of LiDAR annotation in 3D mapping, localization, training data training data preparation and related AI processes.
From understanding the basics of LiDAR technology to annotation mechanisms and the importance of accurate annotation in preparing your data, we'll cover the main principles of this technology. Whether you're a beginner or an expert in the field, this article will provide the most comprehensive overview possible of the world of LiDAR annotation and its impact on the development of technologies such as autonomous vehicles, robotics and many others.
Let's discover the potential of LiDAR annotation for 3D mapping and localization. Let's get started!
You have to start somewhere: what is LiDAR annotation?
LiDAR annotation is a process that transforms raw LiDAR data into a format that machines can understand and learn from. Basically, this involves labeling or tagging various objects in the data collected by LiDAR sensors.
What is a LiDAR sensor? It's a device that emits light waves that bounce off objects and return, creating detailed 3D maps of the sensor environment (i.e. what surrounds the sensors). The process transforms this 3D point cloud point cloud data into valuable information for applications such as autonomous vehicles, identifying and classifying different objects such as trees, cars, pedestrians and road signs.
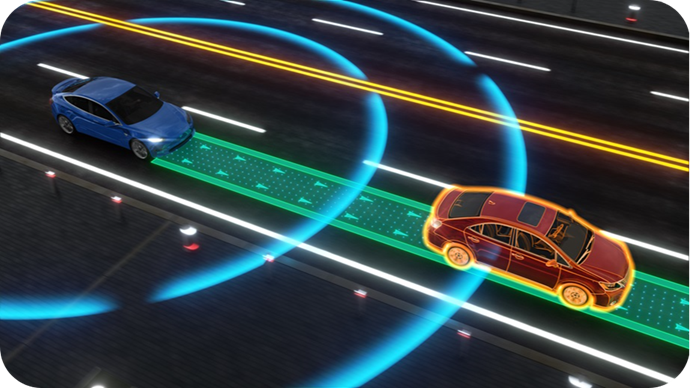
In short, LiDAR is a bit like a photographer capturing every tiny detail of a scene with pinpoint accuracy. LiDAR data enables machine learning models to learn and improve their ability to detect and interact precisely with the elements around them.
How does LiDAR work?
LiDAR is based on 4 essential components:
1. A laser
It emits pulses of light, often in the ultraviolet or near-infrared range, towards surrounding objects.
2. A scanner
This mechanism adjusts the speed at which the laser scans targets and determines the maximum range of laser pulses.
3. A sensor
It detects light pulses reflected from surfaces. The system evaluates the distance between the surface and the LiDAR by measuring the round-trip time of the light pulse.
4. A GPS
It precisely locates the LiDAR system, which is crucial to the accuracy of distance measurements.
Thanks to technological advances, modern LiDAR systems are extremely efficient at collecting detailed spatial data. Once collected, this data is compiled into a point cloud. This cloud represents a set of spatial coordinates of the detected objects, enabling the creation of a precise three-dimensional representation of the environment surrounding the LiDAR system.
Although LiDAR systems are capable of collecting a massive amount of spatial data, it must be borne in mind that this raw data is not directly exploitable by artificial intelligence in its initial form. Indeed, before being used effectively in AI applications, this data must undergo a pre-processing. This process generally involves steps such as noise filtering, normalization and segmentation, to transform the raw data into a more structured, usable format.
Segmentation, for example, helps to separate different elements of the point cloud, such as buildings, vehicles and vegetation, into distinct categories. This pre-processing is necessary for the performance of AI algorithms, as it reduces complexity and improves model accuracy when processing and interpreting LiDAR data in the training process. Pre-processing transforms raw data into a rich, structured set, suitable for advanced computer vision and spatial modeling applications.
In short, annotating LiDAR data requires the use oflabeling tools which enable annotators to create 3D bounding boxes around objects, apply semantic segmentation to classify materials and surfaces, and label each point in the point cloud with attributes such as height, length and depth.
Expertise in LiDAR annotation requires in-depth knowledge of the technology and its applications, as well as proficiency in the use of specialized specialized annotation tools. Annotators also need a sharp eye for accurately labeling objects and understanding their spatial relationships. As the demand for autonomous vehicles continues to grow, so does the need forLiDAR annotators annotators capable of producing high-quality annotations that enable machines to learn to navigate complex environments.
How does LiDAR annotation help with 3D mapping?
With advanced 3D capture technology (or a 3D super camera) like LiDAR, every feature such as trees, buildings, vehicles and roads in the 3D point cloud data is identified and classified with great precision. This attention to detail is very important for autonomous vehicles, for example, as it enables them to perceive and understand their environment with great clarity. Let's take an example (yes, we're going to talk about cars again!): thanks to LiDAR, an autonomous car can distinguish a pedestrian from a traffic sign, enabling it to make more informed and safer driving decisions, and above all avoid an accident!
LiDAR annotation mechanisms use advanced tools and software to guarantee the accuracy of annotations. These tools enable annotators to efficiently labelhuge amounts of point cloud data, ensuring that each data point contributes to creating a complete 3D map. This level of detail feeds the machine learning models used in autonomous vehicles, enhancing their performance by improving their perception of depth and spatial relationships in the real world.
What's more, the accuracy of LiDAR annotation is decisive in a variety of other applications beyond autonomous driving. For example, LiDAR is used in urban planning to create detailed city models, in forest management to monitor forest health, and in archaeology to discover and document ancient structures without physical excavation. Each application benefits from the precise 3D mapping provided by LiDAR annotation, enabling professionals in these fields to make informed decisions based on high-quality data.
In short, LiDAR annotation is fundamental to converting the point cloud or data into usable information. A meticulous annotation process ensures that every object and surface in the 3D point cloud is accurately labeled, enabling practical applications such as autonomous vehicles.
How important is LiDAR annotation for localization?
Localization goes beyond simple object detection. It involves understanding the vehicle's position in relation to its environment in real time. With LiDAR annotation, 3D bounding boxes and semantic segmentation provide detailed images of spatial relationships, enabling vehicles to "see" the 3D world in layers and attributes.
This depth annotation, supported by advanced LiDAR annotation tools, enriches point cloud datasets with information needed for accurate localization. Moreover, accurate localization has a direct impact on the performance and safety of autonomous vehicles. Incorrect localization can lead to misjudgments and potentially dangerous situations.
Consequently, accurate annotation of LiDAR data, facilitated by LiDAR annotation services skilled in handling the large volumes of data needed to train artificial intelligence models, plays a critical role in improving the safety features of these vehicles. It enables the correct identification of target objects and points on the ground, ensuring safe navigation through complex environments.
Let's discover the main advantages of LiDAR annotation
Precision and detail
LiDAR annotation provides highly accurate and detailed 3D maps of the environment. Every tree, building and road is precisely identified and categorized. This level of detail is crucial for autonomous vehicles and other high-precision applications.
The "engine" of autonomous vehicles
By creating clear, navigable maps through the annotation of point cloud data, LiDAR helps autonomous vehicles understand their environment. It improves their ability to detect objects and surfaces, ensuring safer navigation in complex environments.
Enhanced machine learning models
Annotating LiDAR data feeds machine learning models with detailed information. By understanding the 3D world in layers and attributes thanks to annotated data, AI systems improve in performance and reliability.
Semantic segmentation
This process divides point cloud data into categories, making it easier for machines to understand different elements of the 3D world. This is essential for analyzing and reacting accurately to the environment in applications such as automatic driving and urban planning.
User interface and experience
The accuracy of LiDAR annotation contributes to the development of more intuitive and ergonomic interfaces in technological products, particularly in augmented reality (AR) and virtual reality (VR) applications.
Navigation safety
For autonomous cars, the detailed environmental mapping provided by LiDAR annotation helps to avoid obstacles and make informed decisions, significantly reducing risks and improving safety features.
Advanced annotation tools
The use of sophisticated tools and software in LiDAR annotation guarantees efficiency and accuracy in the labeling process. These tools enable fast and efficient annotation of large datasets, supporting the development of AI and machine learning.
In summary, LiDAR annotation has become a prerequisite in many industries, as it is required as part of the development of certain artificial intelligence models. Its advantages in terms of accuracy and detailed mapping make it an indispensable method for data automation and analysis.
What about LiDAR annotation services?
Whatever your industry, obtaining quality data in large volumes is never an easy task. LiDAR annotation LiDARannotation solutions and services offer products for the most demanding engineering teams, providing data on demand. These players provide Data Labelers which label features in the point cloud data from LiDAR sensors, making the data useful for machines which can then interpret it. We have already mentioned on several occasions that these services are used, in particular, for on-board AI in autonomous vehicles. Several studies show that object detection accuracy in autonomous driving improves by over 25% with the help of advanced LiDAR annotation.
Using an outsourced provider to deliver quality annotations is becoming a necessity for AI teams. Growing market demand indicates that LiDAR annotation services are not just a gas pedal, they are an essential tool shaping the future of AI.
Main applications of LiDAR annotation in everyday life
If you've followed us this far (thank you!), you'll have understood that LiDAR annotation is an important process in the analysis and use of LiDAR data. You'll also have understood that one of the main applications of this technology is related to autonomous vehicles. As a reminder, LiDAR data annotation involves the labeling of data points in 3D point clouds, helping machines to understand and interact with the physical world. Labeled data can then be used to train an artificial intelligence model, which in turn automatically understands and interprets LiDAR data in real time.
LiDAR annotation has many applications in a wide range of industries. Here are a few of the most significant uses.
Autonomous vehicles (as if we hadn't insisted enough!)
One of the most revolutionary applications of LiDAR annotation is, of course, the relatively recent development of autonomous vehicles. LiDAR sensors mounted on autonomous cars collect data points from the surrounding environment, creating a 3D point cloud.
Through processes such as semantic segmentation and object detection, AI models can identify objects, pedestrians and other vehicles around them, ensuring safe navigation. This technology relies heavily on precisely annotated LiDAR data to understand depth, the relationship between objects and to make real-time decisions.
Urban planning and infrastructure development
LiDAR point cloud annotation services also greatly benefit urban planners and civil engineers. Using 3D LiDAR point cloud data, these professionals can generate accurate models of cities, landscapes and infrastructure projects.
This enables precise planning and simulation of construction projects, environmental analysis and preservation of historic sites. The point cloud data annotation tool helps create detailed, stratified 3D maps that can be used for land use assessment, flood risk assessment and urban planning, for example.
Robotics and drones
In robotics, LiDAR is used for navigation and obstacle avoidance. LiDAR-equipped drones are often used to monitor or inspect hard-to-reach infrastructures. LiDAR enables robots and drones to move and operate autonomously in complex environments, providing essential data on their immediate surroundings.
Agriculture
In agriculture, LiDAR is used to assess and monitor crop health, or to obtain real data for crop prediction. Data obtained from drones flying over crops can be used to optimize irrigation, fertilization and pest control strategies. In addition, LiDAR helps identify areas suffering from water stress or nutrient deficiencies, enabling farmers to intervene in a targeted manner and improve overall crop productivity. This technology plays a key role in modern agriculture, helping to maximize resource efficiency and minimize environmental impact, while increasing crop production.
Environmental management and forestry
In environmental management and forestry, LiDAR annotation helps create detailed topographic maps and assess vegetation health.
By analyzing point cloud data, researchers can detect ground points and measure forest biomass, helping to manage forest resources. This application of LiDAR data helps to monitor and preserve ecosystems, manage natural resources and plan sustainable development practices.
Archaeology and cultural heritage
Perhaps one of the least obvious applications of AI. We're not afraid to say it: LiDAR annotation is (positively) transforming archaeology and cultural heritage preservation. 3D LiDAR scanning enables archaeologists to discover and document ancient structures and landscapes beneath vegetation, without disturbing the site.
Annotated LiDAR data provides precise details of object boundaries and features, enabling the creation of accurate 3D reconstructions of archaeological sites. This not only aids research, but also the preservation and sharing of historical treasures with the world.
Security and defense
LiDAR is used in a variety of security and defense applications, including border surveillance, area mapping and target detection. Thanks to its ability to provide precise, detailed images of terrain and infrastructure, LiDAR is particularly useful for drawing up response plans in the event of natural disasters or security incidents. In the military field, LiDAR is used for terrain reconnaissance and strategic surveillance, offering advanced detection capability even in conditions of reduced visibility, such as at night or in difficult weather conditions.
Whether annotating bounding boxes for autonomous vehicles, segmentation for urban planning, or object detection in environmental analysis, LiDAR annotation accurately describes the 3D world to help build tomorrow's advanced technological products.
Last point
In short, LiDAR annotation is a powerful tool that helps machines understand and interpret the world around them. It involves the labeling of 3D point cloud data, making it easier for computers to recognize objects such as cars, trees and buildings in three-dimensional space. This process is essential for the development of autonomous vehicles and other advanced technologies that rely heavily on the perception capabilities of machines. The LiDAR annotation process can be complex, but its importance cannot be underestimated as it paves the way for the construction of safer, more efficient automated systems.
Now that you've read this guide, let us know if you think you have a better understanding of LiDAR technology, LiDAR annotation, and its importance in the world of technology? Don't hesitate to tell us about your use cases using LiDAR data. !