Semantic segmentation: how does it transform our view of the world?
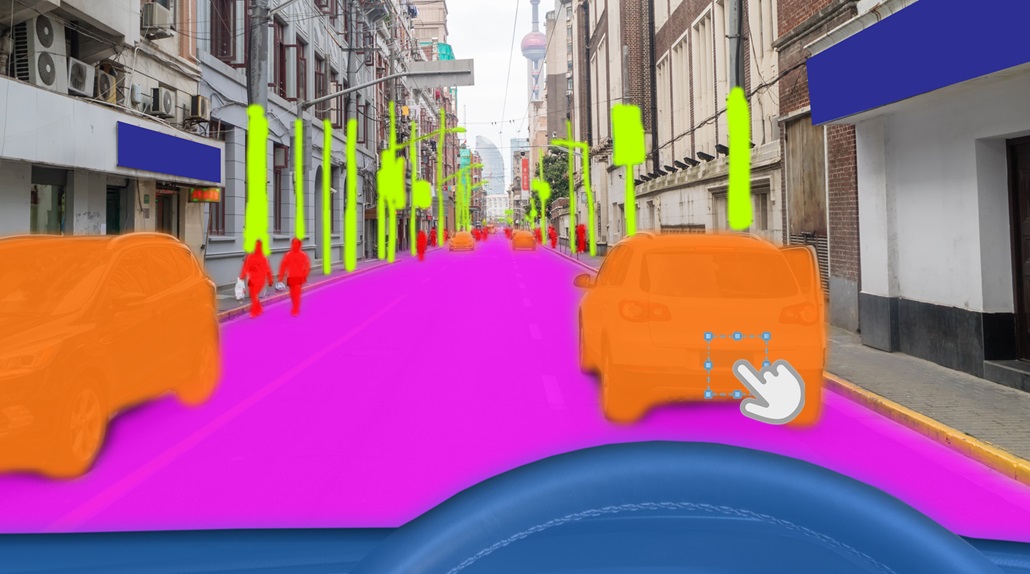
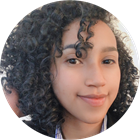
The π semantic segmentation is at the heart of advances in computer vision and artificial intelligence. It represents one of the most relevant image processing methods for understanding and interpreting visual scenes.
β
By segmenting an image into different regions and assigning each pixel a label corresponding to its semantic class, this technique enables π precise analysis of visual content. Semantic segmentation also identifies different parts of the image, including the background, improving the accuracy of the analysis.
β
Since its first uses in the 2000s, semantic segmentation has seen significant development, driven by advances in machine learning algorithms and neural network and deep neural network architectures. Neural networks, in particular π CNN, π FCN, π U-Net, π DeepLab and π PSPNetplay an essential role in training and structuring semantic segmentation models.
β
This development has opened up new perspectives in fields as varied as autonomous driving, medicine, cartography and augmented reality. Find out more in this article!
β
β
What is semantic segmentation and how does it work?
β
Semantic segmentation is a π image processing technique which involves dividing an image into different regions and assigning each pixel a label corresponding to its semantic class. This technique enables pixels to be classified into different classes, making it easier to understand the image. To improve segmentation accuracy, it is often useful to use a set of predefined classes or a specific data set.
β
In other words, it enables us to understand what each part of the image represents. To achieve this, semantic segmentation uses machine learning algorithms, in particular deep neural networks.
β
These are trained on large amounts of data to recognize and classify different visual elements. They are able to learn to identify specific features in an image, such as contours, textures and colors. In addition, neural networks identify different parts of an image, including the background, by analyzing the spatial and contextual relationships between pixels. This enables them to segment the image according to its semantic content.
β
β
β
β
β
β
β
What are the main areas of application for semantic segmentation?
β
Semantic segmentation has a variety of applications in a number of different fields, including :
- It is commonly used in computer vision for π object recognition and π image classification. For example, in the medical field, it is used to segment radiological images to identify anomalies. In the automotive industry, it is essential for the development of AI used by autonomous vehicles, helping to detect and classify objects on the road.
- In addition, semantic segmentation often uses predefined datasets or class sets to improve the accuracy and efficiency of the algorithms.
β
Computer vision and recognition of objects in an image
Semantic segmentation plays an important role in computer vision, enabling the precise detection and classification of objects in images. By segmenting an image into semantically significant regions, this technique enables computer vision algorithms to understand the composition of the scene and identify each object present.
β
It also distinguishes objects from the background by using segmentation masks to isolate regions such as the ground, sky or other elements from the main object. Deep learning plays a key role in this process, enabling semantic segmentation models to efficiently identify different parts of an image, including the background.
β
This is particularly important for applications such as video surveillance. In this field, fast and accurate object detection can be critical to safety. The same applies to autonomous cars, where semantic segmentation is used to detect and identify pedestrians, vehicles and obstacles on the road.
β
Cartography and navigation
In cartography, semantic segmentation is used to create accurate, detailed maps by automatically identifying the various elements of a scene, such as roads, buildings, trees and pedestrian areas.
β
This precise segmentation is essential for the creation of digital maps used in GPS navigation, urban planning and natural resource management.
Β
In the field of navigation, semantic segmentation is also used to help robots and autonomous vehicles interpret their environment, identifying obstacles and planning safe trajectories.
β
Medicine and medical imaging
In medical imaging, semantic segmentation is used to automatically segment and identify the different anatomical structures in medical images, such as organs, tumors or blood vessels.
β
This precise segmentation is essential for diagnosing diseases, planning treatments and monitoring the evolution of pathologies, as part of the development of medical AI.
β
For example, in magnetic resonance imaging (MRI) and the AI models developed around this technology, semantic segmentation is used to identify and measure the shape and size of brain tumors, helping doctors to assess disease progression and plan treatments.
β
Satellite image analysis and terrain reconnaissance
Semantic segmentation is widely used to analyze satellite images, automatically identifying different types of terrain, such as forests, waterways, urban areas and farmland.
β
This precise segmentation is useful for environmental mapping, natural resource monitoring, land management and urban planning. For example, in environmental monitoring, semantic segmentation is used to detect changes in land cover.
β
It can detect deforestation, urbanization and erosion of materials and soils. This enables researchers (and sometimes political decision-makers) to effectively monitor and manage fragile ecosystems.
β
Virtual and augmented reality
In virtual and augmented reality, semantic segmentation is used to recognize and segment objects and surfaces in the real world. This enables augmented reality applications to incorporate virtual objects realistically into their environment.
β
For example, in augmented reality video games, semantic segmentation is used to detect flat surfaces, such as tables and floors. A virtual object can then be realistically placed on these surfaces. This guarantees an immersive experience for players.
β
Similarly, in virtual reality applications, semantic segmentation is used to detect obstacles and objects in the virtual environment, enabling users to interact realistically with their virtual environment.
β
β
Semantic segmentation: a bridge between human perception and artificial intelligence?
β
Semantic segmentation plays an essential role in bringing artificial intelligence closer to understanding and interpreting visual scenes. This opens up new perspectives in fields such as computer vision, robotics and augmented reality.
β
Similar understanding of the environment
Semantic segmentation enables AI to understand visual scenes in a way similar to human perception. It can divide an image into different regions and assign each pixel a semantic meaning. In this way, it enables algorithms to recognize and categorize objects and scene elements in the same way as a human being would.
β
Contextual interpretation
Just as humans interpret a scene by taking into account the context and relationships between different elements, semantic segmentation also enables AI to analyze images contextually. By identifying the spatial and semantic relationships between objects, it enables algorithms to understand the overall meaning of the scene and act accordingly.
β
More natural interaction
By understanding visual scenes in a way similar to human perception, semantic segmentation makes interaction between humans and machines more natural and intuitive.
β
For example, in augmented reality applications, segmentation at the semantic level enables algorithms to detect flat surfaces and obstacles. As previously mentioned, this enables them to place virtual objects more realistically, making the user experience more immersive and satisfying.
β
Semantic segmentation: what prospects for the future of technology?
β
Semantic segmentation has the potential to shape the future of technology on many levels.
β
Improved machine perception
Semantic segmentation will continue to improve the ability of machines to perceive and understand their environment in a similar way to human perception. This will pave the way for significant advances in fields such as robotics, autonomous driving and augmented reality. This technique could enable machines to interact more intelligently and intuitively with the world around them.
β
Development of new applications
Semantic segmentation will pave the way for innovative new dimensions and applications in fields such as health, education, agriculture, urban planning and the environment. For example, it could be used to monitor the condition of agricultural crops, analyze medical images to diagnose disease, or assess the impact of climate change on the environment.
β
Integration with other emerging technologies
Semantic segmentation will be increasingly integrated with other emerging technologies such as the Internet of Things (IoT), virtual reality (VR) and blockchain. This technological convergence will open up new opportunities for innovation and value creation in fields such as logistics, security, entertainment and e-commerce.
β
β
What are the ethical implications of using semantic segmentation?
β
The use of semantic segmentation raises complex ethical issues that require careful thought and appropriate regulation to ensure its responsible and ethical use in society.
β
Privacy and personal data
Semantic segmentation can be used to extract sensitive information from an image, such as facial recognition or mass surveillance. This raises concerns about privacy and the risk of intrusive surveillance.
β
Strict policies and regulations are essential to ensure that personal data is not misused.
β
Bias and discrimination
Like any machine learning algorithm, semantic segmentation models can be subject to bias, reflecting the biases present in the training data.
β
This can lead to discriminatory or unfair results, favoring some groups or marginalizing others. It is crucial to implement bias mitigation techniques and ensure transparency and fairness in the design and use of these models.
β
Responsibility and automated decision-making
In some fields, such as autonomous driving or medicine, semantic segmentation is used to make critical decisions that can have a direct impact on people's lives.
β
This raises questions of liability in the event of error or system failure. We need to clarify the legal and ethical responsibilities of the developers, manufacturers and users of these automated systems.
β
Impact on jobs and professions
The increasing automation of tasks thanks to technologies such as semantic segmentation can lead to economic and social disruption, changing job requirements and replacing certain jobs.
β
It is crucial to put in place retraining and social protection policies to mitigate the negative effects on affected workers.
β
Conclusion
β
Semantic segmentation is essential in the field of computer vision and deep learning, offering significant advances in the understanding and interpretation of visual scenes. Its diverse applications, from autonomous driving to medicine, open up new technological and societal perspectives.
β
However, while semantic segmentation offers many exciting prospects, it also raises technical, ethical and social challenges. It will be crucial to develop more advanced techniques to overcome the current limitations of semantic segmentation, such as accurate segmentation in low-light conditions or in complex environments.
β
In addition, it will be essential to meet the ethical challenges of privacy, transparency and fairness in the use of this technology.