Top 10 image annotation platforms for AI / Computer Vision projects [2025]

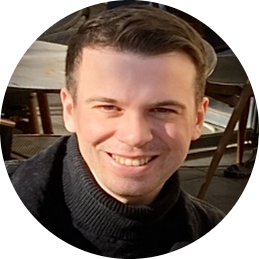
💡 In this article, discover the 10 best image annotation platforms for your IA projects.
Introduction: Understanding the importance of image annotation for Computer Vision projects
As we all know, AI developments rely on vast quantities of data. The foundations of the most innovative products, such as ChatGPT or the AI embedded in cutting-edge autonomous vehicles, rely on massive datasets to achieve exceptional (if still imperfect) levels of performance. These advances would not be possible without access to high-quality, large-scale data, which highlights the importance of advanced image annotation platforms, whose recent progress is enabling the industrialization of AI development practices.
In this article, we dive into the landscape of image annotation platforms to present you with the best options to meet your specific needs. Whether you're working on models 🔗 "Computer Vision" models, such as object detection models, or other Machine Learning / Deep Learning-related tasks, the principles of 🔗 data augmentationdata augmentation, 🔗 image segmentation or 🔗 annotation with Bounding Boxes are probably not unfamiliar to you.
As an independent player and user of this type of platform, we've listed our top 10 AI annotation platforms existing on the market to help you make informed decisions and produce high-quality data for your models. Below are the platforms we'll be covering in this article:
🔗 #1 - Encord: ideal for the most complex data and cases.
One of the newest and most powerful tools on the AI data processing market. Encord is a comprehensive platform designed to unleash the potential of data-driven AI. It enables the development, testing and deployment of large-scale predictive and generative AI systems. Encord offers tools for creating high-quality training data, using active learning pipelines, assessing model quality and much more. The platform is particularly effective for annotating various visual modalities, managing large-scale annotation teams with customizable workflows and integrated quality control tools.
What you'll love about Encord:
- Task management and automation: Encord simplifies and optimizes complex workflows, increasing efficiency and productivity. However, there are no dashboards to monitor the activities (work time, number of labels produced) of your team of Data Labelers.
- Well-documented API: Encord integration into existing pipelines is easy, and customer support is responsive and efficient
- Advanced annotation features and stability: Encord stands out for its remarkable speed and stability when processing videos, as well as its support for DICOM files, useful for radiological studies. Encord is particularly appreciated in the medical community.
- Workflow and team management: Encord's latest updates include functions for managing teams of data labelers working on the same dataset. It is also possible to extract data on the time spent on certain tasks or the number of labels produced.
- Collaboration and quality control: Encord facilitates collaboration between engineers and annotators, eliminating the need for additional communication platforms.
What you might not like:
- Performance issues (relatively minor): Users have reported occasional problems of latency and slowness, sometimes related to the location of annotation teams. These problems are offset by extremely responsive support.
- User interface sometimes confusing: Some users feel that the interface could be improved.
- Initial complexity: There is an initial learning curve, partly due to gaps in documentation.
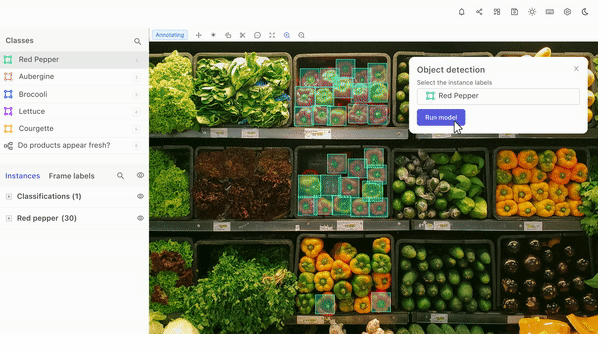
🔗 #2 - V7 Labs, a comprehensive annotation tool, one of the market leaders
V7 (Darwin) is an AI training data platform for annotating images, videos, documents and medical imaging files. It is designed to rapidly provide high-quality annotated data for training Computer Vision models. V7 features self-labeling, MLOps workflow management, and the ability to call on the services of professional annotators.
What you'll love about V7:
- Automatic annotation features: V7 integrates tools like SAM for auto-annotation, facilitating annotation of complex data and 🔗 pre-labeling for human-in-the-loop strategies.
- Intuitive user interface: The interface is intuitively organized and the tools are easy to use, even for the most complex tasks.
- Workflow and team management: The platform makes it easy to manage teams and workflows. The interface for tracking working hours and Data Labelers' productivity is one of the most comprehensive on the market.
- Data export options: V7 offers several options for exporting annotations, including integration with AWS S3 to automatically decompose videos into images.
What you might not like:
- Lack of flexibility for file manipulation: Unlike services like Google Drive, V7 doesn't allow you to manipulate files/folders on its platform (no Drag & Drop, for example).
- Filtering problems: It can be difficult to filter or sort documents in large datasets.
- Protecting your data and intellectual property: Some contractual clauses, particularly in the trial version, are rather vague about V7's ability to use your data once you have entered it into the platform.
- Occasional slowness: The platform can sometimes be slow when working with large datasets.
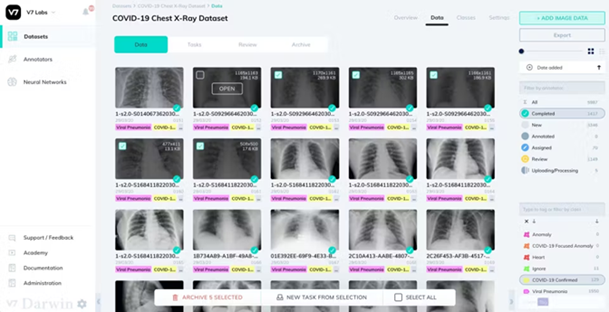
🔗 #3 - Labelbox: expert in collaborative annotation. A versatile solution for large-scale AI projects.
Labelbox is an annotation platform appreciated for its ease of use, efficiency and versatility. It stands out for its intuitive interface and fast learning curve.
What you'll love about Labelbox:
- Work time tracking: Users appreciate the ease of tracking work time and total number of labels per Data Labeler or shift.
- Simple interface: Labelbox has a straightforward, easy-to-understand interface for label designers and annotators alike.
- A "user-friendly" tool: The platform is considered very user-friendly and easy to use for beginners.
- Ergonomic shortcuts: Users appreciate the annotation tool's shortcuts, which are ergonomic and well-designed for practitioners.
- Python API support: The platform offers easy integration into pipelines via its Python API.
- Efficient workflows: Labelbox makes it easy to filter labels or tags, improving project efficiency.
What you might not like:
- Lack of customization: Some users lament the absence of customization options, such as changing the background.
- Multichannel image management: Labelbox has difficulties with multichannel images, common in scientific imaging but rare in computer vision.
- Performance problems: Bugs and sluggishness have been reported, particularly during updates or with high-resolution images.
- Segmentation tool limitations: Precise segmentation can be difficult, especially for small image portions.

🔗 #4 - Scale Rapid: fast, flexible, but not for every budget
Scale Rapid is a platform renowned for its speed, ease of implementation and efficiency in processing large datasets. It stands out for its ability to provide high-quality training data, and its capacity to deliver data (with an embedded crowdsourcing service) in a very short timeframe.
What you'll love about Scale Rapid:
- Easy to set up and use: Users appreciate the ease with which they can configure Scale Rapid in their environment and use it to produce image labels in a matter of hours.
- Numerous data formats supported: The platform supports various data formats such as CSV files, text files, images and videos.
- Fast labeling: Scale Rapid is renowned for its fast data processing, offering real-time feedback on labeling instructions.
- Flexible payment, "on-demand" model: The ability to pay as you go is particularly appreciated, especially in budget-constrained environments.
What you might not like:
- User interface: Many users feel that the user interface could be improved to be more user-friendly and interactive.
- Complexity of the pricing model: Scale Rapid's pricing structure is seen as complex and sometimes costly, which can be an obstacle for some users.
- Limitations in certain use cases: While the platform is effective for many projects, it doesn't always deliver the most accurate or efficient results for all types of project.
- Lack of precision: In some cases, annotated data are not as precise as expected, requiring further improvements and additional (chargeable) review cycles.
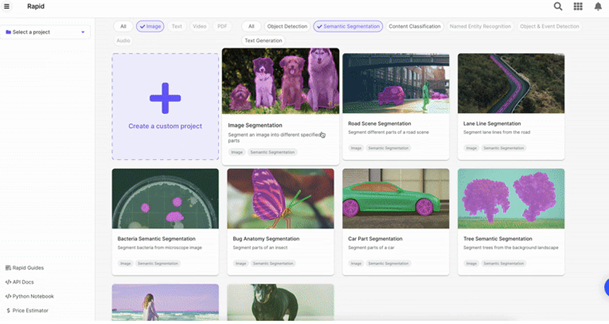
🔗 #5 - CVAT.ai: a pioneer, known and recognized by Data Scientists... moving upmarket
CVAT (Computer Vision Annotation Tool): is an annotation tool designed for professional teams, focusing on data labeling for Computer Vision algorithms. It is open-source, developed under MIT license and can be installed on-premise or in the Cloud. More recently, a web-based offering "CVAT.ai" has emerged, with a very simple pricing model, eliminating lengthy installation or maintenance processes.
What you'll like about CVAT.ai:
- Open-source and professional: CVAT is professional open-source code, making it easy to adapt and use on-premise or in the cloud. If you want to keep the data in your working environment, nothing could be simpler: just install CVAT on one of your servers.
- A web-based version: The tool is now available online, making it easy to access and use without downloading and maintenance. Its pricing model is very simple (transparent price per user).
- Versatility: CVAT.ai is adaptable and adjustable for a variety of annotation scenarios, making it useful in diverse contexts (sports, retail, automotive, etc.).
- Support for numerous data extraction formats: CVAT's main strength is that it supports more download formats than most of its competitors, offering unprecedented flexibility.
What you might not like:
- Performance problems: On the web version, if the CVAT.ai server goes down, there may be a loss of data, as annotators cannot save their work. For larger volumes of data, we recommend setting up an additional backup process. If you work with a team of more than 3 data labelers, managing concurrent access can also be a problem.
- Limited data import functionality: Data import functionalities are not very ergonomic, and performance problems have been reported by many users.
- Lack of functionality: Although the pricing model is straightforward, it lacks functionality for tracking hours worked, number of image tags produced, and so on.
- Outdated interface: The user interface is considered a little dated, and could benefit from an update to bring it into line with other labeling platforms on the market.
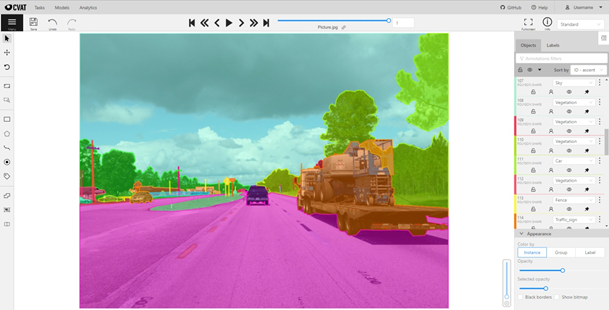
🔗 #6 - Label Studio: Open Source doesn't mean incomplete
Label Studio is a versatile data annotation tool, often described as a Swiss Army knife in this field. It is a professional tool for machine learning, essential for building successful AI-based products.
What you'll love about Label Studio:
- Open-source and professional: Label Studio is open-source code developed under an MIT license, guaranteeing high accessibility and adaptability. The tool is powerful and can be easily installed on your own environments, including Clouds (AWS, Google Cloud, etc.). The tool is regularly updated and covers a wide range of use cases. It is accessible via a web interface.
- Tailor-made labeling interfaces: Label Studio is flexible and can be configured quickly, with a high number of templates and a configurable interface using XML code. Label Studio can be used for different types of data, including audio, video and text.
- Machine Learning integration: The tool is designed to integrate easily with a multitude of frameworks on the market.
- Accessibility: Although there is a "Pro" package available for a fee, the vast majority of Label Studio's functions are free of charge. So you can install Label Studio on your servers and start working, without worrying about license fees, number of users, etc.
What you might not like:
- Maintainability: You need to install Label Studio on your environments. Practical from a data protection point of view, this means you need to take into account the costs associated with the use and maintenance of your servers (on-premise or Cloud).
- Limited collaboration features: Label Studio features user access management. However, the platform could benefit from improved tracking of working hours and the number of labels produced by teams. In addition, collaborative working sometimes poses performance problems: with poorly managed concurrent access, your annotators have difficulty working on common data sets. The solution is to duplicate your initial dataset into a multitude of sub-datasets, which implies considerable curation work prior to labeling.
- Data import and export: While Label Studio has made progress in this area over the past two years, data import and export functionalities are still rather unstable and limited, and can sometimes generate errors. There are well-documented APIs for integrating Label Studio with your ML setup, but some users have reported performance and instability problems when using these APIs.

🔗 #7 - Kili: a platform full of promise
Kili Technology is a comprehensive annotation tool designed to quickly label training data, identify and correct problems in datasets, and simplify annotation operations. The software dramatically speeds up the construction of reliable AIs, and offers customizable interfaces for a variety of data types, including images, videos, text, PDF documents, satellite imagery and conversations.
What you'll love about Kili:
- Stability: Kili offers the stability essential for working with large volumes of data and tight deadlines. It is easily adaptable to a variety of use cases, including Computer Vision and NLP.
- Collaborative features: The platform enables effective collaboration between team members and offers detailed analytical tracking, necessary when working with a large number of collaborators.
- Workflow: Kili lets you control the labeling queue and set up review processes to ensure annotation consistency and quality.
- Automatic annotation features: The platform offers automation tools such as interactive segmentation and tracking to speed up labeling without compromising quality.
What you might not like:
- Limited functionalityfor video annotation: Although improvements are planned, the current functionalities for 🔗 video annotation are limited. Kili is not suitable for video use cases, particularly when processing medical videos. Many problems note instability issues for video processing.
- Lack of advanced analytics: Some users would like more advanced analytics to monitor their team's productivity.
- Complexity of the API: For those without technical knowledge, using the API can be complex.
- Environment setup time: The initial set-up of projects on the platform can take time, especially for new users getting used to the interface.
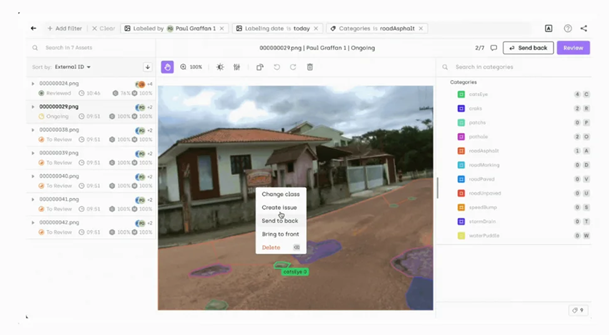
🔗 #8 - SuperAnnotate: a complete, user-friendly solution
SuperAnnotate is a data labeling platform renowned for its efficiency and versatility in managing image and video annotation tasks. It is widely recognized for its ease of use and efficiency in accelerating the annotation process.
What you'll love about SuperAnnotate:
- Ease of use: Users find the platform extremely user-friendly, even for beginners, with a concise and intuitive user interface.
- Time-saving features: The platform is equipped with automatic labeling functions such as "Magic Select", considerably reducing the time and effort required for the most time-consuming tasks.
- Efficient data management: SuperAnnotate offers excellent Data Management services, making it easy to manage different projects simultaneously.
- Excellent customer support: The platform is known for its excellent, responsive and helpful customer service, particularly beneficial for small businesses or new platform users.
- Collaboration and accessibility: SuperAnnotate enables collaborative working without requiring in-depth programming knowledge, making it accessible to a wider range of users.
What you might not like:
- Low accuracy of auto-segmentation functionality: Some users have experienced problems with the accuracy of auto-segmentation, particularly with low-resolution images.
- Limitations of the selection tool: The inability of the Magic Select function to concatenate different parts of selections into a single object can be a limitation for some specific tasks.
- Financial commitment: The platform prefers upfront financial commitments, which may not be feasible for all users, especially when the number of labels required is uncertain.
- Loading time : Users have noted delays in loading the next image when passing an image, which can slow down workflow.
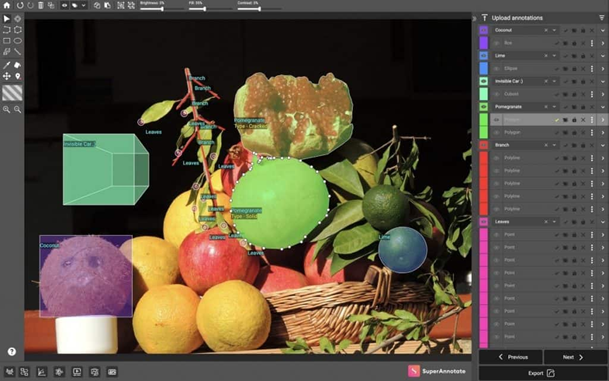
🔗 #9 - Dataloop, a Data Management platform for AI featuring a data annotation module
Dataloop is a complete platform covering the entire AI lifecycle, from development to production. It includes a data management and annotation platform to facilitate data generation for supervised learning, as well as automation pipelines to accelerate Computer Vision projects.
What you'll love about Dataloop:
- Easy-to-use interface: Dataloop offers an intuitive interface, making the tool easy to use, even for complex research projects such as text-to-image generation.
- Reactive, personalized customer support: Dataloop's support team is responsive and works closely with users to adapt the tool to their specific needs.
- Intuitive Python SDK: The Python SDK is easy to use, enabling rapid integration into projects.
What you might not like:
- Limited functionality for certain use cases: For certain atypical projects, such as image annotation with varied questions and a broad taxonomy, Dataloop may lack specific functionality, although the support team can often find solutions to remedy this.
- API for Human-in-the-Loop annotation: Dataloop's API for manual annotation may not meet all needs.
- Platform complexity: Dataloop is a comprehensive platform but can be complex to master, with some learning time required to use it fully.
- Notifications are sometimes late: Delays in notifying pipeline problems can lead to delays in production.
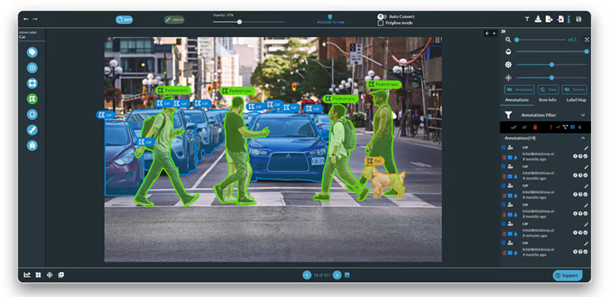
🔗 #10 - Playment, a market veteran and a reliable solution
Playment is a veteran in the (young) AI solutions market. Playment's GT Studio is a no-code data annotation platform designed to help ML teams create high-quality, diverse reference datasets efficiently. GT Studio is a web-based labeling platform that eliminates inefficiencies for the annotator and project manager through ML-assisted annotation tools and easy-to-use workflow management software.
What you'll love about Playment:
- Flexible and used in many fields: Playment can produce training data for multiple sectors, such as e-commerce, banking, document management, etc.
- Ease of use: The application is simple but powerful, ergonomic, with fairly few slowness compared to many platforms on the market.
What you might not like:
- High price: Although Playment offers quality services, cost can be seen as a brake, linked in particular to a per-annotated-object pricing model.
- Lack of functionality to produce customized reports: The Playment application's reports are not fully customizable and may lack some of the fields required to effectively drive its labeling activities.

Bonus: Labellerr, the perfect choice for agile, collaborative annotation management
🔗 Labellerr is a modern, intuitive platform designed to meet AI data management needs with a collaborative, agile approach. This solution enables AI systems to be developed, tested and deployed quickly and efficiently. Labellerr offers tools for automated data annotation, team and project management, and quality control. It supports diverse data types such as images, videos, text, audio and PDF files, making it extremely versatile for Machine Learning teams.
What you'll love about Labellerr:
- Task managementand automation: Labellerr enables automated data annotation and facilitates exploratory data analysis (EDA). This significantly increases team efficiency and productivity. The ability to manage multiple projects and users simplifies collaborative working.
- Intuitive user interface: Labellerr's user-friendly interface makes navigation through its functionalities quick and easy. Users can concentrate more on their annotation tasks without worrying about the complexity of the tool.
- Precision and efficiency: Labellerr's advanced algorithms guarantee high accuracy in data annotation. Smart Labelling functionality and the integration of AI generation models save precious time.
- Responsive customer support: Labellerr's support team is always on hand to offer technical assistance and advice, greatly enhancing the user experience and project quality.
- Flexibility and diversity of data formats: Labellerr supports a variety of data formats, making it suitable for a multitude of use cases, whether for image, text or audio annotations.
What you might not like:
- Minor performance issues: Some users have reported latency problems when handling large volumes of data. However, the auto-backup feature minimizes the impact of these slowdowns.
- Customize shortcuts: Customizing keyboard shortcuts could improve annotation efficiency, although this feature is not yet available.
- Limited support for 3D models: Although Labellerr excels at annotating 2D data, support for 3D models is still under development, which could limit its use in specific fields.
- Integration complexity : Although Labellerr offers integration capabilities with popular Machine Learning frameworks, this may require additional development efforts to integrate the tool into existing workflows.
Conclusion: choosing the right tool for your use case has a direct impact on your AI pipeline.
Here we are: thank you for reading our list of the 10 best free and paid image annotation tools. Whether you're labeling small datasets or building complex AI models, we're confident that the right tool for your specific use case will have a direct impact on both the annotation process and your results.
Make sure you consider all your options carefully to avoid wasting time and money on a tool that doesn't meet all your needs.
Not sure where to start? Innovatiana is an independent player that can help you choose the image annotation platform best suited to your needs. We offer personalized advice to help you select the ideal tool, taking into account the specifics of your projects, your budget and your objectives. If you've already made your choice, we can also put our team of specialized and expert Data Labelers at your disposal, ready to take on your annotation projects with efficiency and precision. 🔗 We look forward to hearing from you!