Data Labeling is a profession, not a menial job
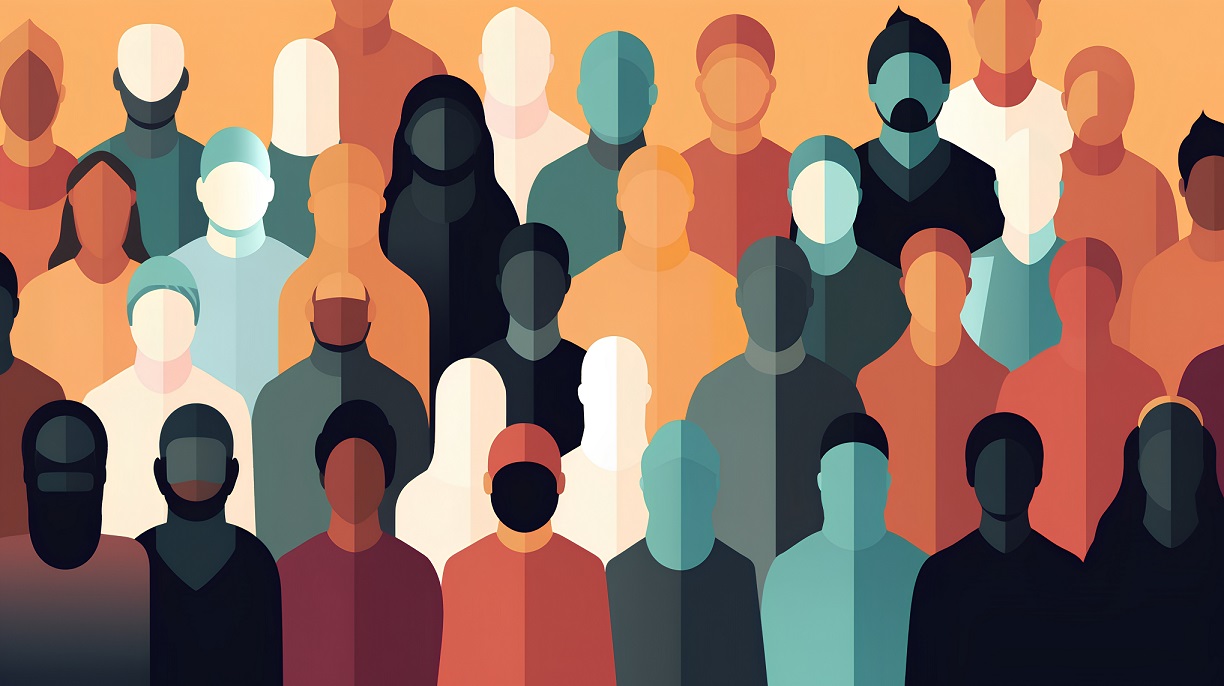
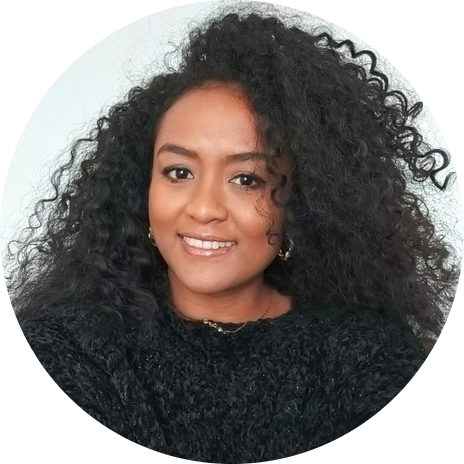
[Source on which our article is based: Deep Learning AI - The Batch - Issue 204 - https://www.deeplearning.ai/the-batch/issue-204/]
β
In a data-centric approach to AI, the development of high-performance AI products depends on accurately annotated data
β
However, the demanding nature of Data Labeling work and the costs associated with large-scale data annotation are encouraging companies to look for solutions to automate annotation work, or resort to low-paid freelance providers. These Data Labelers, often sourced via platforms such as Amazon Mechanical Turk or Upwork, are in high demand and sometimes tend to botch the job to meet the strict deadlines imposed on them, or give up. Yet everyone would benefit from considering data annotation less as an occasional job or "odd-job", and more as a profession in its own right.
β
β
How does the data annotation industry work?
Companies providing annotation services (or Data Labelers), such as Centaur Labs, Surge AI or Remotasks (part of Scale AI) and many other major players in the sector, use automated or manual crowdsourcing systems to manage freelance workers from all over the world. Freelance data labelers have to pass qualification exams, undergo training and be regularly assessed to perform tasks such as plotting "Bounding Boxes".Bounding Box"on images or videosThey are required to pass qualification exams, undergo training and undergo regular assessment to perform tasks such as tracing "Bounding Boxes" on images or videos, classifying sentiments expressed in publications on social networks, evaluating video clips of a sexual nature in certain cases, sorting bank transactions or evaluating chatbot responses.
β
β
Challenges related to job and salary stability for freelance Data Labelers
The salary scale for Data Labelers varies considerably depending on the location of the workers and the task assigned to them, ranging from $1 an hour in Kenya to $25 an hour or more in the USA. Some tasks requiring functional or specialized knowledge, sound judgment and/or a significant amount of work can be paid up to $300 per micro-task.
β
If a Data Labeler is absent for a day to go to the doctor, or suffers a power cut or Internet connection failure, he or she is immediately replaced by the crowdsourcing system. What's more, this system has no tolerance for moments of fatigue or temporary performance problems: a few mistakes too many, and that's the end of the contract for the Data Labeler!
β
Considering data-labeling to be a simple task accessible to all, companies are looking to cut costs drastically, even to the point of negotiating indecent hourly rates. But make no mistake: it is not possible to obtain both a quality service and respect for fundamental human rights at less than EUR 5 per hour (which is already very low!) for a Data Labeler, whether he or she is located in India, the Philippines or Madagascar.
β
Unfortunately, today's system is too impersonal: in order to protect their customers' trade secrets, companies assign tasks without revealing to the Data Labelers the identity of their customer, the application or the function concerned. Data Labelers do not know the purpose of the annotations they produce, and undertake not to talk about their work. The result is a loss of meaning, and data sets of mediocre to poor quality... not ideal for training models!
β
β
Challenges related to the instructions given to Data Labelers and their training
Instructions for labeling tasks are often poorly documented and ambiguous. For example, these tasks may call for the annotation of clothes worn by human beings, which excludes clothes on a photo of a doll or cartoon character. But what about images of clothing reflected in a mirror? Does a suit of armor count as clothing? What about diving masks? As data scientists and developers iterate on their models, the rules governing data annotation become increasingly complex, forcing annotators to take into account a growing variety of exceptions and special cases. At the first mistake or oversight, data labelers risk losing their jobs! Very often, their customers have not made the effort to accurately document any special or atypical cases, exceptions or potential data quality problems in the initial set. In many cases, no discussion is possible between the customer and the freelance Data Labeler, who finds himself in difficulty and ends up abandoning his work, even if it means not being paid for the work already carried out on the crowdsourcing platform. This is an aberration!
β
β
βChallenges related to working conditions, schedules and the uncertainty of data annotation micro-tasks.
In the world of Data Labeling, work schedules are often sporadic and unpredictable. Workers don't know when the next task will arrive, how long it will last, whether it will be interesting or overwhelming, or whether it will be well or poorly paid. This uncertainty, combined with the discrepancy between their hourly wages and their employers' earnings as reported in the press, can demoralize workers.
β
Many annotators deal with stress by clandestinely grouping together on WhatsApp to share information and ask for advice on how to find interesting tasks and avoid work they deem undesirable. They learn tricks, such as using existing artificial intelligence models to do the work for them for the simplest tasks, connecting via proxy servers to mask their location and creating multiple accounts to protect themselves from suspension if they break the rules set by the companies offering them work.
β
β
The importance of the Data Labeler profession and quality data annotation
The development of high-performance AI systems depends on accurately annotated data. However, the strict financial constraints of large-scale annotation encourage companies to use the cheapest solutions on the market, choosing the lowest hourly rate, without consideration for the quality of the data produced, the ethics of Supply Chain AI or the volume of hours that will be imposed on Data Labelers. Yet everyone would benefit from considering data annotation less as an occasional job and more as a profession in its own right.
β
The value of skilled Data Labelers (or annotators) becomes even more apparent as AI professionals adopt data-centric development practices that enable efficient systems to be built with relatively few examples. With far fewer examples, the proper selection and annotation of those examples is absolutely essential.
β
β
Manual data labeling is an expensive and laborious process, but it's the best way to create quality data sets for training AI models. With Innovatiana, we offer expertise, skilled labor and automated controls to handle data needs at scale. Talent is everywhere. Opportunities are not. We want to contribute, at our level, to redressing this injustice by creating jobs in Madagascar, with fair wages and ethical working conditions.
β
AΓ―cha CAMILLE JO, CEO of Innovatiana.