Video annotation projects: what are the most common mistakes?
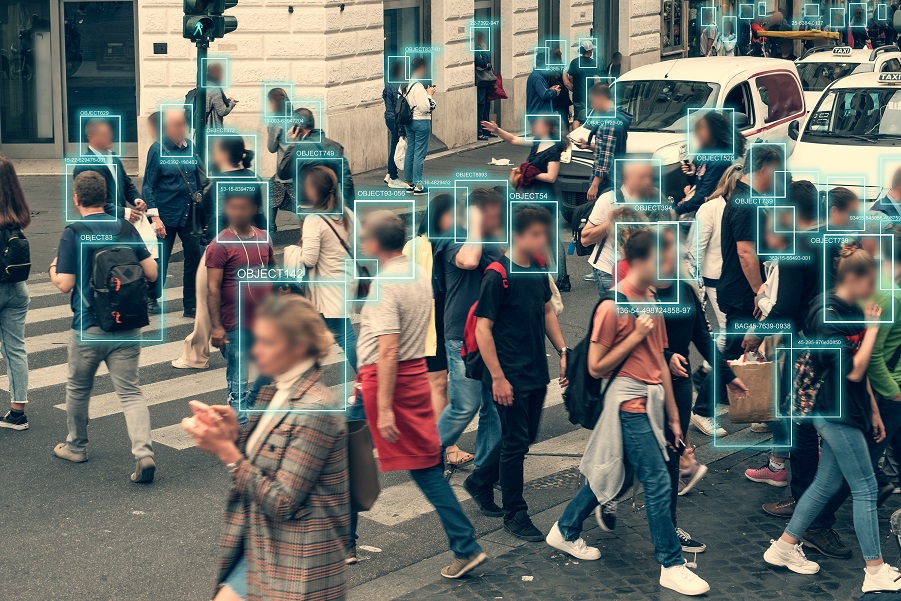
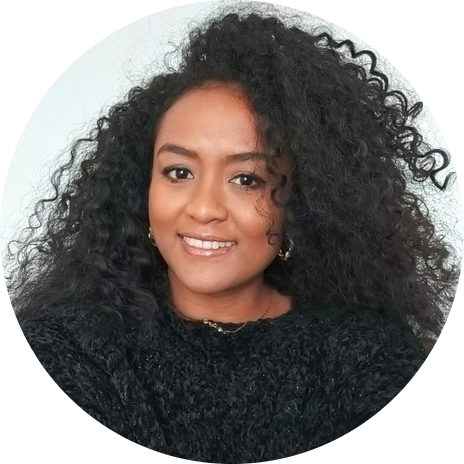
Video annotation projects: learn from common mistakes to train high-performance models!
β
Getting into data annotation for AI is one thing when it's just images. It's simple enough - a little concentration, patience, and anyone can claim to be an image annotator. Now, move on tovideo annotation, and you're playing in a totally different league.
β
Let's say you have a one-minute video clip. If the framerate is 24 frames per second, which is pretty standard in video, you need to manage 1,440 frames. Imagine that - 1,440 distinct moments to label, each representing a "piece" of the action. This greatly increases the complexity of your annotation task. And we're talking about optimized videos... I haven't even mentioned the case of π medical videos for example, the π noise or low resolution, which are all factors of complexity in video annotation.
β
Mastering video annotation is more than patience and attention to detail. You need real know-how and a strategy for analyzing and π content moderation strategy. Fortunately, we're here to help! With a few expert tips, skilled annotators and tricks from our experience, you'll be mastering video editing and annotation for your IA needs in no time.
β
β

β
β
Introduction to video annotation in artificial intelligence (AI)
β
Data annotation (including video annotation) is an essential element in the process of training algorithms to understand and accurately interpret visual information. Annotation is the language of data. Video annotation involves thelabeling of objects, actions and events in video images, enabling AI models to recognize and react appropriately to these elements. In this article, we explore the field of video annotation to understand its importance in the process of building high-performance AI products, as well as the most common pitfalls associated with video labeling.
β
The field of data annotation (including video annotation) corresponds to data labeling and video annotation classifying data to teach computers to recognize and differentiate between various objects or scenarios. Let's take a simple example: a dataset filled with images of cats and dogs. By annotating each image with the right labels, we pave the way for a computer vision model to accurately identify whether a given image or video contains a cat or a dog, or several cats or dogs.
β
Data annotation is a critical task when it comes to creating AI solutions that work in the real world. It is the cornerstone of AI models, the language of data in supervised learning models, where AI learns from examples. Adding labels or masks to video content can be done by hand (with manual annotations, made in-house, by your team, or by a service provider) or, to some extent, via automated systems. Labels can be as simple as marking objects in a frame, or as nuanced as identifying complex actions and emotions.
β
β
The applications of AI video tagging are diverse. Here are 4 applications:
β
1. Object detection
β
This involves training AI to spot specific items in video footage. For example, identifying and tracking objects, detecting vehicles, spotting road damage or identifying animals in a video.
β
2. Object tracking
β
Here, AI is trained to track objects through video footage, predicting their next movements. This capability is critical for tasks such as monitoring pedestrians or tracking vehicle movements for safety reasons.
β
3. Locating objects
β
AI is trained to locate objects in video footage and provide their coordinates. This is a useful feature for managing aspects such as a vehicle's ability to park autonomously.
β
4. Categorization / Segmentation
β
This involves categorizing objects in the video. For example, an AI model could be trained to differentiate between humans and vehicles in a video, categorizing them accordingly.
β
β
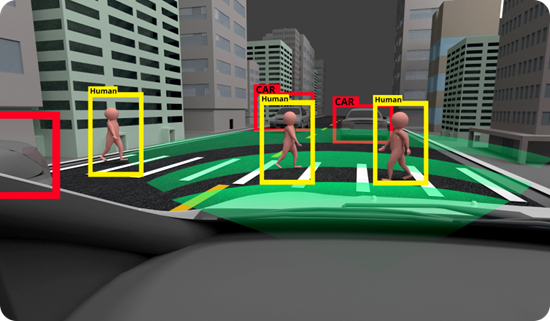
β
By annotating data, we essentially teach our AI models to focus on what matters. The ultimate goal is to create training data and have the models automatically recognize patterns, enabling it toautomatically label new data after a learning curve.
β
β
β
β
β
β
β
Methods used in video annotation for AI: a brief overview
β
AI and data specialists, such as data annotators, use several video annotation methods. The right method depends on the type of video being annotated and the use of the labeled data. Each video annotation tool and method addresses specific types of video training data and project requirements, offering unique benefits and challenges.
β
Here's a list of various annotation methods for videos:
β
β
Bounding boxes: at the heart of object recognition
Bounding boxes are a fundamental video annotation method where annotators draw rectangles around objects. This technique is simple and particularly effective for simple, well-defined objects, and is widely used because of its simplicity and cost-effectiveness.
β
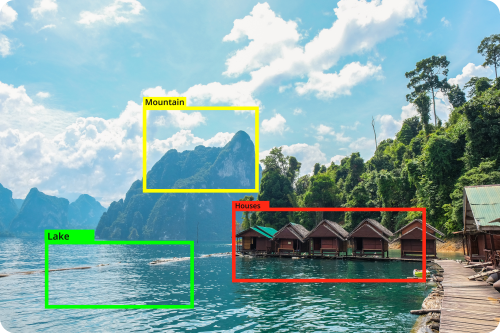
β
β
Semantic segmentation: revealing details with masks
The π semantic segmentation breaks objects down into their individual components. This detailed method involves labeling each part of an object separately, enabling AI systems to distinguish and analyze components of the same object in complex scenes.
β

β
β
Annotation of key points: highlighting specific aspects
Inkey point annotation, specific points or landmarks on an object are marked. This technique is particularly useful in applications such as facial recognition or motion analysis, where precise locations are essential for identifying objects.
β

β
β
3D cuboid annotation: adding an extra dimension
3D cuboid annotation adds a depth dimension, essential for understanding objects in a spatial context. This method is instrumental in applications involving spatial awareness, such as autonomous vehicle navigation and robotics.
β

β
β
Polygonal annotation: capture complex contours
For irregularly shaped objects,polygonal annotation offers a more precise solution than bounding boxes. By drawing polygons (multi-sided shapes) around objects, this method provides a precise outline, essential for complex shapes.
β

β
β
Landmark annotation: precision in detail
Similar to key point annotation,landmark annotation focuses on labeling specific points to identify specific objects in video frames. It's very effective for detailed analyses, such as facial recognition or biometric identification of objects too.
β
Object tracking annotation: follow the movement
Object tracking involves annotating objects across consecutive video frames together, helping machine learning models to track movement and predict future positions. This method of training machine learning models on image data is vital in surveillance, object detection and monitoring applications.
β
Interpolation annotation: smoothing transitions
L'π interpolation annotation uses keyframes to predict and fill annotations in intermediate frames. This is particularly useful in videos where objects move predictably, reducing the workload of frame-by-frame annotation.
β
Video classification: categorizing entire clips
β
In video classification, entire video files or clips are labeled according to their content or general theme. This high-level annotation helps AI systems to understand and categorize videos, such as distinguishing between genres in films or video archives.
β
β
β
What's the advantage of annotating videos rather than images?
β
Videos are made up of sequences of frames, but annotating them as complete videos rather than isolated frames offers a wealth of contextual information that greatly benefits AI models. What's more, many recent video annotation tools and services are equipped with additional features that improve the efficiency of working with large video datasets and make the work of data labeling teams much easier.
β
Annotating video sequences has many advantages, particularly for improving the training of AI models and expanding real-world applications. Here are some key benefits:
β
Efficient annotation with interpolation
Some AI annotation tools simplify the process by enabling interpolation. Instead of annotating each video frame yourself, you can simply mark the beginning and end of a sequence. The software then automatically fills in the intervening frames, saving time and effort.
β
Capturing temporal dynamics
Videos, unlike static images, encapsulate movement and change over time. By annotating videos, you provide rich data that helps AI models understand object movements and temporal variations, an aspect that static images cannot convey.
β
Richer data for AI training
Videos naturally contain more information than single images. This abundance of data, when annotated, provides a comprehensive learning resource for AI systems. Such detailed data input can dramatically improve the accuracy and performance of AI models.
β
Profitability and time efficiency
Video annotation can be more economical than image annotation. A single video can provide many data points, and focusing on key images reduces the overall time spent on the annotation process.
β
Wider real-world applications
Annotated videos offer a more accurate representation of real-world scenarios. This increased realism is crucial in training AI models for diverse applications, ranging from sports analysis and medical diagnosis to agricultural monitoring.
β
β
It's important to recognize that video annotation is always a complex and time-consuming task. Effective use of video annotation requires mastery of specific tools and workflows . Despite these challenges, the benefits of video annotation in advancing AI capabilities and applications are undeniable.
β
β
What is the role of data annotators (or video annotators)?
β
The role of a video annotator is essential in the development of sophisticated artificial intelligence systems. By meticulously adding labels and tags to various segments and frames of video footage, these professionals annotate videos to provide the critical data needed to train AI models. This process, commonly known as annotation, is essential for teaching AI systems to accurately identify objects, and understand various objects, actions or patterns in video content.
β
Beyond simple recognition, annotated videos can also teach AI about the context, movement and interaction of different elements within a scene. This is particularly important in fields such as the development of autonomous vehicles, where understanding dynamic environments is crucial, or in healthcare, where AI helps interpret complex medical images.
β
What's more, the quality and accuracy of video annotation directly impacts the effectiveness of AI training. Data annotators need to provide accurate video annotations to ensure that AI models learn correctly, reducing errors in real-world applications. This requires them to have a keen eye for detail and a good understanding of the subject. As with any other job, this requires training and experience.
β
What's more, video annotation is evolving with the advent of new technologies. Advanced tools, such as automated video annotation software and its specialized machine learning algorithms for annotation, are increasingly used to streamline the process, making it faster and more efficient. However, human supervision remains essential to guarantee the reliability and accuracy of the data supplied to AI systems.
β
β
Video annotators play a pivotal role for Computer Vision algorithms by bridging the gap between raw video footage and intelligent AI applications, enabling the creation of smarter, more intuitive technology solutions across a variety of industries.
β
β
β
β
β
β
β
The video annotation process for Computer Vision models
β
Let's now take a closer look at the video annotation process, which is fundamental to the development of Computer Vision technologies. A streamlined AI annotation pipeline can help solve the video annotation task efficiently. The following methodology can be applied for successful and rapid delivery of labeled video data:
β
Selecting and training annotators
The process begins by assembling a team of qualified annotators. They must receive comprehensive training to align them with the project's objectives. Their expertise, particularly in understanding the nuances of the project, is essential. Integrating their skills with AI-assisted tools (for example, a recent, collaborative data annotation platform) can greatly speed up the manual annotation process while guaranteeing accuracy.
β
Selecting tools for enhanced annotation
Choosing the right video annotation tools is crucial. Select tools offering advanced video management capabilities, a user-friendly interface and features such as event-based classification, dynamic object tracking and efficient interpolation. The tool must also support efficient project and team management, ensuring a smooth workflow.
β
Definition of project objectives and data preparation
Setting clear project objectives from the outset establishes a focused direction for the annotation process. Depending on the objectives, such as differentiating between animal species or categorizing objects by color, the type and volume of data required will vary. Collecting a diverse dataset covering various scenarios and objects is crucial to developing a robust AI model.
β
Implementing annotation strategies
With objectives and tools in place, develop a consistent annotation strategy. This involves creating guidelines that ensure consistency across all annotated data, and annotating videos correctly. It's important to adapt these strategies to the needs of the project, aligning them with the expected results of the AI model.
β
Iterative revision and refinement
Continually reviewing and refining annotations is key. Regular quality checks and updates not only improve annotations but also correct inaccuracies. Modify annotations according to feedback on AI model performance, ensuring continuous improvement and relevance.
β
Feedback loop for continuous improvement
Establish a feedback loop that uses AI model performance information to refine both the annotation process and annotator training. This ensures that the project evolves in parallel with the model, leading to more accurate and efficient annotations over time.
β
By adopting this methodology, the video annotation process can be made more efficient, facilitating the development of advanced AI models in Computer Vision.
β
β
Some video annotation techniques
β
The following is a list of methods and best practices for video annotation, which can contribute to the success of your projects. This list, although not exhaustive, can be shared with annotators as part of their training:
β
Understanding the task and choosing the right annotation method
It's important to first understand the specific requirements of the dataset and task, and in particular the business. Just as Data Scientists can't work in silos, Data Labelers need to have a solid grounding and ideally knowledge of your use case sector. For example, specialized Data Labelers have expertise in medicine, and are therefore able to annotate medical videos.
β
An annotation strategy is also necessary for any project, and must take into account preferred techniques and methods, to be communicated to the team of annotators, and configured in the labeling platform. For example, estimating human posture in video data requires the key point method to label human joints. On the other hand, bounding box annotation alone may not provide sufficiently detailed information. The choice of annotation method must correspond to the project's objectives to guarantee effective training of the AI model.
β
Single image annotation method
This traditional approach involves breaking down the video into individual frames or images, which are then labeled separately. Suitable for videos with minimal object movement or smaller datasets, this method can become time-consuming and costly for larger video datasets due to the huge number of frames requiring annotation.
β
Multiframe or stream annotation method
In this method, objects are labeled in video streams. Annotators track objects and their coordinates frame by frame as the video plays. Faster and more efficient, this approach is better for larger datasets, guaranteeing accuracy and consistency in object detection and labeling, and gaining in popularity with advances in video annotation tools.
β
Automatic annotation tools for continuous frames
Recent advances in video annotation include automatic annotation features that simplify the process. Machine learning algorithms follow objects across frames, maintaining continuity. These algorithms analyze pixel movements between frames to accurately track objects that may appear intermittently throughout the video. Automation lightens the annotation workload and improves data consistency.
β
Adapt to changing annotation needs
As AI and machine learning technologies advance, video annotation methods must also evolve. Annotators and project managers need to keep abreast of the latest tools and techniques to ensure that video annotation services and processes remain efficient, accurate and up-to-date with developments in AI and various Computer Vision models and techniques.
β
β
β
What about video annotation tools and platforms?
β
Video annotation platforms are available in paid and free versions, each serving different purposes and user groups.
β
What are the advantages of a paid video annotation tool?
β
Paid annotation platforms are generally preferred by professional teams engaged in commercial Computer Vision projects, particularly those in the machine learning fields. These platforms are designed to manage large datasets and provide comprehensive support throughout the machine learning lifecycle, thanks to advanced features such as collaboration or automatic annotation. Key features of these paid platforms often include:
β
- Sophisticated ontology capabilities for complex sub-classifications within labels.
- The ability to manage and annotate long videos and image sequences.
- Numerous functions for various types of annotation, such as bounding boxes, polygons, polylines, key points and primitives.
- Customizable workflows for review and annotation, enabling efficient monitoring of annotator performance and task allocation.
- Advanced features for automated π automated pre-annotationleveraging proprietary algorithms or AI models to streamline the process.
- Collaborative features for consensus annotation, as well as precise management of manual annotation activities.
β
β
β
β
β
β
βFree video annotations? What's the trick?
β
Conversely, free video annotation tools seem to be a preferred choice for academics, AI enthusiasts and students. These tools are well suited to individuals or groups working on localized projects, or those who don't require scalability for their projects. Free tools offer essential video annotation services and capabilities, making them ideal for educational purposes, small-scale research or personal machine learning projects.
β
But is it impossible to carry out large-scale projects with these tools? Recent advances prove otherwise. Open Source projects such as π Label Studio have demonstrated that they have powerful embedded functionalities capable of supporting ambitious projects. This tool offers remarkable flexibility in terms of supported annotation types and customization, enabling users to adapt it to varied and complex projects. Its Open Source nature encourages continuous improvement, thanks to an active community and a constantly evolving range of features. This demonstrates that, although designed to be accessible and inexpensive, some free video annotation tools can rival paid options in terms of capabilities and efficiency, making high-quality video annotation more accessible to a wider range of users and projects. Not convinced? Take a moment to view the following video:
β
β
β
β
In summary - each type of platform brings its own set of advantages. While paid platforms offer extensive functionality and scalability for larger, more complex projects, free tools offer an accessible, cost-effective option for learning, experimentation and smaller-scale tasks.
β
β
β
5 challenges and pitfalls in video annotation
β
β1. First pitfall: underestimating the complexity of annotation
βTheart of detail in video annotation
One of the main mistakes made by AI development teams is to underestimate the complexity inherent in video annotation. Often thought of as a simple labeling task, video annotation is actually far more nuanced than it seems.
β
Videos can contain a multitude of objects, actions and contextual information that require careful observation and precise annotation. Ignoring this complexity can result in inaccurate annotations, misleading AI models during training. To overcome this challenge, project managers need to spend time defining clear annotation guidelines, properly training annotators and establishing a quality review and correction loop, to ensure consistency and quality throughout the video annotation process itself.
β
β
2. Lack of communication with annotators
Collaboration for successful annotation
β
Smooth communication between project managers and annotators is of paramount importance in achieving accurate video annotations. A lack of communication can lead to confusion about (sometimes ambiguous) marking criteria, project objectives and deadlines.
β
To overcome this problem, open communication is essential. Regular meetings, daily monitoring via information-sharing platforms, clear documentation of guidelines and prompt responses to annotators' questions can greatly improve annotation quality. Instilling a sense of collaboration and shared responsibility ensures that the entire video annotation services team is aligned with project objectives.
β
β
3. Neglecting the iterative nature of the data annotation process
βVideo annotation: an evolutionary process
β
Video annotation is rarely a one-off project. Annotating large volumes of video data involves iterative cycles of rapid initial annotation, then revision and refinement. Some organizations fall into the trap of viewing annotation as a linear task with a fixed end. However, embracing the iterative nature of annotation enables continuous improvement in annotation accuracy and quality.
β
Regularly reviewing the annotated video data and incorporating feedback on the AI model's performance, making adjustments as necessary in response to the emergence of new patterns, are essential for refining the annotation process and achieving better results over time and trials.
β
β
4. Underestimating the importance of quality control
βEnsurethe consistency of labeled data through quality control measures
β
Quality control is the cornerstone of any successful video annotation project. Without effective quality controls, inconsistencies and errors can creep into the annotated video dataset itself, adversely affecting the performance of the AI model. Setting up a robust quality control mechanism (with Labeling Managers in charge of iterative quality reviews, for example) involves random checks, double annotations for verification and periodic audits to identify and correct inaccuracies.
β
A complementary approach can also be to implement "consensus" reviews, by asking several Data Labelers to review the same video dataset, in order to select the results that meet a consensus to guide the model. By implementing rigorous quality control measures, AI teams can guarantee the reliability and accuracy of their annotated video datasets themselves, which very often results in better performing AI models.
β
β
5. Errors associated with labeling platform performance problems in video annotation projects
β
An important but sometimes overlooked element in video annotation projects is the performance of the labeling platforms used. The quality of annotations largely depends on the labeling tool used by annotators. Robust platforms offer features to effectively manage the complexity of video annotation tasks, such as precise time synchronization, functionality to pre-annotate or draw labels easily and accurately, and advanced project management capabilities.
β
What's more, when it comes to poor-quality or low-resolution video, high-performance labeling platforms are all the more essential. Problems such as blurring, noise or compression can make annotation difficult and lead to errors. Labeling platforms with enhanced visualization tools and magnification capabilities can help annotators to accurately identify objects and label actions, even in difficult conditions.
β
β
In conclusion...
β
Video annotation projects offer considerable potential for training AI models to accurately recognize and interpret visual content. By learning from the mistakes and key pitfalls discussed in this article, it is possible to build an effective video labeling process.
β
Recognizing the complex nature of video annotation tasks, fostering open communication, embracing the iterative process and prioritizing quality control are the pillars on which successful video annotation projects are built.
β
β
Find out more about video annotation projects with Innovatiana... Find out in this article how Innovatiana's Data Labelers are making a real impact on complex video annotation projects, particularly those involving delicate medical videos(π link). Dive into this article describing one of our successful collaborations with π SDSC. And if you'd like to find out more about our services, don't hesitate to ask us for a π quotation.
β