How do you recruit the best data annotators for your AI projects?

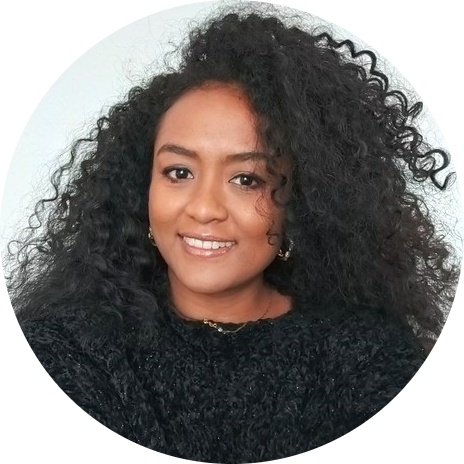
Data annotators are often regarded as the unsung heroes behind the rapid advances in artificial intelligence. Every day, we discover incredible new products designed with AI in mind. One of the latest is the Apple Vision Pro, a futuristic headset that relies heavily on technologies such as Computer Vision.
Behind the scenes of AI, teams of data annotators play a very important role in system development. These professionals label/mark data and ensure the quality and accuracy of the annotated data. In short, the accuracy of AI models largely depends on the different data annotation methods used by these annotators (also known as "Data Labelers") in the AI development cycle.
Whether you're looking for in-house data annotators, freelancers or external professionals from third-party companies specializing in data annotation for AI, you need the best experts capable of bringing your AI projects to fruition. That's why we've compiled a comprehensive guide that covers everything you need to consider when recruiting data annotators, or preparing a call for tenders for dataset labeling. Let's get started!
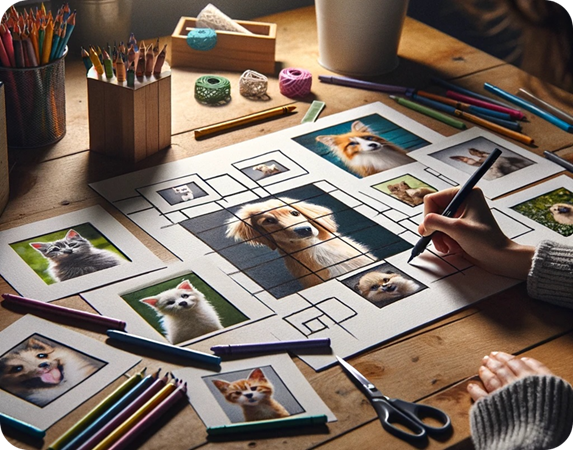
What is a data annotator?
Let's start with the basics. What is a data annotator or data labeler ? A data annotator is a person who labels and tags data used to train machine learning models (i.e. to produce training data for AI). Working in teams, these professionals meticulously examine and interpret the data and add labels, text annotations and metadata that help machine learning algorithms understand the models and make accurate predictions.
To feed an AI model with data, a large amount of raw or unstructured data is first collected. Then, data annotators carry out a tedious process to label and categorize the data and make it more structured. Once data annotation is complete, the organized data is used to "feed" the AI model and train it to autonomously reproduce the same tasks of object detection or recognition.
In short, data annotators play a key role in training AI models by annotating and labeling large volumes of data. For example, chatbots rely heavily on large volumes of pre-processed and labeled text to function. When the data annotator labels samples of text data to add hints about their meaning and concrete intent, this helps the chatbot to learn correctly by giving it precise contextual indications.
Data annotators also validate annotated data to ensure accuracy when training models. Therefore, it's necessary to assemble teams of expert data annotators who you can trust and who can contribute to the success of AI projects.
Today, data annotators help develop highly capable AI systems that power a wide range of applications, such as natural language processing (NLP), image recognition and sentiment analysis. This implies that the ability to analyze, label and tag data is the key skill to look for in a data annotator. Often misperceived (some would say: "anyone or any clickworker can annotate images, this work doesn't deserve to be paid properly"), this profession requires technical skills, rigor as well as a significant work capacity to produce quality "ground truth" data sets.
What are the main responsibilities of a data annotator?
Data annotators are involved in various data collection and processing responsibilities. We have identified two key responsibilities of a data annotator, namely labeling/marking data and validating annotated data.
1. Data labelling and marking
The main responsibility of data annotators is to label data types using tagging and labeling tools. This involves associating metadata with a set of thematic data, in the same way as adding subtitles to a film. The job of annotators is to accurately assign labels and tags to a wide variety of unstructured data types, such as videos, images or text.
Data labeling essentially requires the data annotation specialist to assign sentiment scores to texts or images, or to categorize images into relevant classes using objects such as Bounding Box or Polygons. The task of annotating or labeling data involves marking specific characteristics or attributes within the data.
In short, data labeling and tagging enable artificial intelligence models to classify objects, recognize patterns and deliver accurate results by learning from quality data.
2. Validation of annotated data
Another important responsibility of data annotators is to validate the annotated data. This involves validating the quality, accuracy and consistency of the labeled data.
Validating annotated data is important because it eliminates inaccuracies, biases and inconsistencies in training data. Therefore, data annotators help to validate annotated data and ensure that models are trained with reliable data sets.
In concrete terms, what are the day-to-day tasks of a data annotator?
Although labeling/marking and validation are the core responsibilities of a data annotator, it's essential to delve deeper into their day-to-day tasks to get a full understanding of their role. Here's an overview of the tasks these data professionals perform on a daily basis:
Analyze data
Data annotators meticulously examine and dissect raw data to identify unique attributes, patterns and features that will facilitate annotation processing by AI. This analysis ensures that the annotator understands the context and complexity of the data, leading to more accurate and meaningful annotations.
Developing guidelines
To maintain consistency and accuracy in the annotation process, data annotators create comprehensive guidelines and instruction manuals. These resources serve as a reference for other annotators, ensuring that everyone follows a unified approach and adheres to the same standards. Sometimes, it's useful to develop a register of errors and atypical cases, which is fed in over the course of the project, to serve as a reference base for dealing with the most complex cases.
Assigning labels and other tags
With an eye for detail and a rigor characteristic of this profession, data annotators assign relevant labels and tags to raw and unstructured data. This process involves categorizing, classifying and adding metadata to data, making it more accessible and valuable to machine learning algorithms.
Validate annotated data
Data annotators review and verify the quality, accuracy and consistency of annotated data, ensuring that it meets project requirements and standards. This may involve identifying and correcting errors, resolving ambiguities and providing feedback to other annotators to improve overall data quality.
Interact with other teams
Collaboration is an important aspect of a data annotator's role. Data Labelers work closely with Data Scientists, Data Engineers and other stakeholders to ensure effective execution of annotation activities. This collaboration can involve discussions on project objectives, progress updates and the resolution of any challenges or concerns through daily exchanges (for example: "I don't know how to classify this medical instrument, can someone help me?" or "The image is very poorly legible, should I annotate or is it better to ignore this image. I'm afraid of impacting the model's results with approximate data).
In addition to these responsibilities, data annotators are charged with maintaining the confidentiality of sensitive data and adhering to strict data security protocols. They must handle data with care, ensuring that it is protected from unauthorized access, use or violation. By doing so, data annotators maintain the integrity of the project and the products using AI.
Different strategies for finding data annotators
Now that we're clear on the role and responsibilities of data processing and annotation experts, let's move on to the main point of this guide: how can I recruit the best data annotation experts? If you've ever explored the possibility of using existing datasets or preparing your own data for your AI, you've certainly come up against this difficulty. Do I need to annotate 5,000 images or 30,000 to get results? Is my dataset sufficiently diverse? Where can I find the equipment to process my data: it's a job that seems extremely time-consuming, repetitive and laborious. It must be extremely expensive!
Don't panic, we're here to help. There are different strategies for finding data annotators. If you talk to older people, they'll probably suggest you use Amazon MechanicalTurk or platforms like Upwork. Is this really the best solution for preparing your data? That may have been the case 10 years ago, but it's not so sure today. ChatGPT and Mistral AI.
Let's take a look at each of these strategies and assess their advantages and disadvantages:
1. Recruit and train in-house data annotators
The first option to consider when building your data annotation team is to hire data annotators in-house. This approach involves recruiting individuals who will work exclusively for your company, dedicating their time and expertise to your projects. By having a dedicated team in-house, you can foster a stronger commitment to the project and develop a deeper understanding of its complexities, as team members focus solely on your organization's goals and objectives.
One of the main benefits of this option is the enhanced collaboration and communication offered. In-house data annotators work closely with other team members. This proximity facilitates seamless collaboration and open communication channels, enabling them to address challenges, share information and streamline the annotation process more effectively. As a result, your team can work together cohesively, ensuring that everyone is on the same wavelength and working towards the same goals.
Another advantage of having an in-house team is improved data security. By keeping sensitive data within your organization, you can reduce the risk of unauthorized access or data breaches. In-house data annotators are more likely to be well-informed about your company's data security protocols and adhere to strict confidentiality guidelines, ensuring that your valuable data remains protected. This is not to say that you should absolutely secure data by neglecting your annotation software. We've already come across customers using very non-ergonomic devices, requiring the use of a certain type of hardware or screen. This brings us back to the 2000s, with a hint of nostalgia perhaps... You have to find a compromise between ergonomics and securing your data (not all data deserves to be secured!).
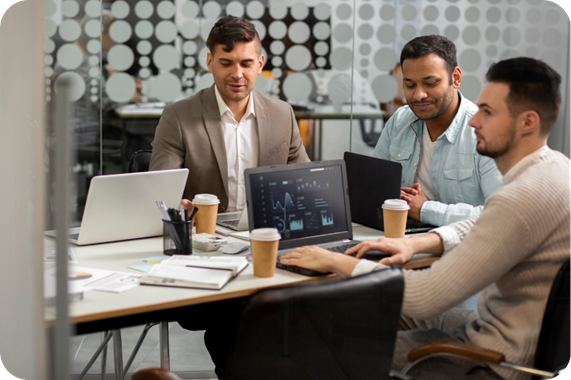
Finally, hiring in-house data annotators represents a long-term investment in your organization's data annotation capabilities. As they gain experience and expertise in your specific field, they become valuable assets who can contribute to multiple projects and help drive your company's data-driven initiatives. By encouraging and developing your in-house team, you can create a solid foundation for future success in your data annotation and analysis projects.
On the other hand, in-house data annotators also present challenges. It's sometimes reassuring to have an in-house team, on site. But it's also expensive. Some companies have told us that they use temporary staff, or even trainees, to carry out labelling tasks. If you're looking for quality data, you're likely to be disappointed. Not that interns and temps aren't (potentially) qualified for annotation work. It's just that you run a high risk of disengaging staff with little or no interest in the AI data business, which will impact on the quality of your data. It is therefore rarely advisable to entrust labeling tasks to your Data Scientists trainees, even if it sounds practical! They will quickly become disengaged due to the complex and time-consuming nature of the task (which is sometimes deemed uninteresting). Give them the task of sourcing AI service providers instead! You'll save time and improve quality.
Advantages of in-house data annotators
(+) Better understanding of the project
(+) Effective collaboration and communication
(+) Higher data security
Disadvantages of in-house data annotators
(-) Tedious recruitment process
(-) Requires resources and effort for training
(-) It's very expensive to maintain an in-house / onshore team, with the risk of discouraging over-qualified teams (e.g. the Data Scientist trainee who becomes a Data Labeler in spite of himself).
In short, having a team of in-house data annotators has both advantages and disadvantages. The final decision depends on your needs. If you want a dedicated team that remains committed to the project, if you have substantial resources: building a team of in-house data annotators seems conceivable. But don't dream: if you process medical data, it's unlikely that a doctor will agree to annotate your data at an hourly rate similar to that of Amazon SageMaker or Clickworker. Alternatively, you can opt for outsourced solutions. Two solutions: freelancers and specialized service providers (such as Innovatiana).
2. Hire freelance consultants for your annotation tasks
Freelance consultants, data processing specialists, AI experts or non-experts, represent another popular choice for companies wishing to hire data annotators on an on-demand, project basis. This approach allows organizations to engage with professionals who may possess specific expertise that matches their project needs, without the long-term commitment associated with in-house hires.
One of the main advantages of hiring freelance consultants is cost-effectiveness and return on investment. By hiring freelancers, you can access the same level of expertise as in-house data annotators, but at a considerably lower cost. This flexibility enables your organization to tailor its data annotation efforts to project requirements, without the financial strain of maintaining a permanent workforce.
What's more, working with freelance data labelers can save your company valuable time in training and integration. The market is full of professionals with diverse expertise and skills, so you can find the right person for your project with minimum effort. As a result, you can quickly assemble a team of experienced data annotation freelancers who can start work immediately and deliver high-quality results within your desired timeframe.
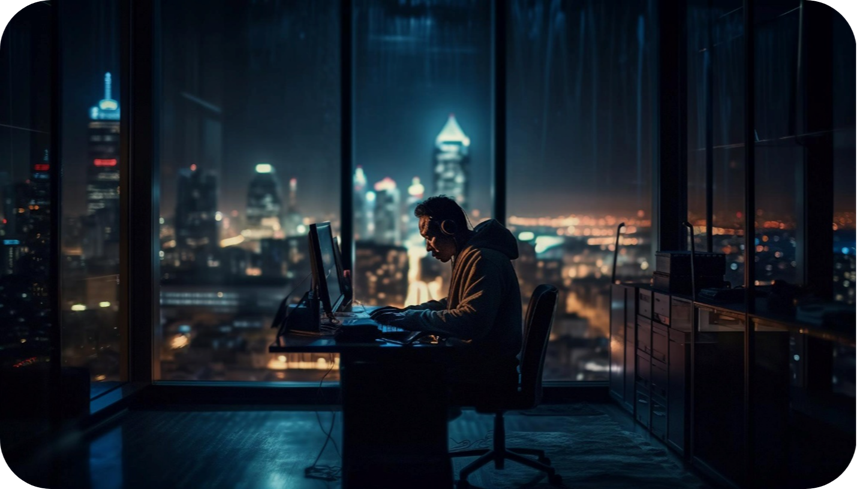
In addition to cost savings and efficiency, consultants have specialized knowledge and experience. They may have worked on projects competing with your company. They bring a wealth of knowledge and best practices to your project. This diverse expertise can prove invaluable in meeting the challenges of annotating complex data, and ensuring that your project benefits from the latest techniques and innovations in the field.
Finally, engaging with freelance data annotation experts gives your organization the flexibility to adapt to changing project requirements. As your data annotation needs evolve, you can easily increase or decrease the size of your team, depending on the scope and complexity of the project. This adaptability ensures that you always have the right resources at your disposal, without the constraints of a fixed workforce.
However, recruiting freelancers also has its drawbacks. The most important is the data security risk. You need to trust these consultants. For this, we recommend signing a non-disclosure agreement. In addition, you may not be able to achieve the same quality of work as with an in-house team, because the in-house team is more committed to your project and has a better understanding of the objectives. Also, the use of freelance consultants requires a major effort in terms of team qualification and mobilization... while this may work well on small data sets, putting together a team of over 5 people who don't know each other and have never worked together will require as much investment as internal recruitment before results can be obtained...
Advantages of hiring freelance consultants
(+) Profitable
(+) Rapid access to expertise and specialized skills
(+) Scalable and flexible
Disadvantages of recruiting freelance consultants
(-) Data security risks
(-) Uncertainty about quality of work and collaborative working mechanisms
(-) Less committed/responsible
Therefore, it's important to strike a balance between cost-effectiveness and quality of work if you opt for freelance data annotation specialists. In addition, make sure you check their qualifications properly and monitor/evaluate the quality of the work regularly.
3. Outsourced professionals from third-party companies
The third strategy for finding data annotators is to outsource to third-party companies specializing in Data Labeling. These organizations have a pool of well-trained and experienced data annotation professionals who can be hired on demand, offering a flexible and efficient solution for your data annotation needs.
Outsourcing data annotators to third-party companies offers many advantages, the most important of which is access to first-rate expertise and experience in the field of data annotation. These professionals are constantly updated with the latest techniques and tools, ensuring that they deliver high-quality data annotation tasks in line with industry best practice. By leveraging their in-depth knowledge and skills, you can ensure that your projects benefit from accurate annotations, helping to ensure the success of your data-driven initiatives.
In addition, AI annotation service providers offer a well-structured methodology that includes appropriate workflows and processes. This structured approach ensures that your annotation projects will be managed professionally, with clear communication channels, well-defined milestones and rigorous quality control measures in place. As a result, you can expect seamless and efficient collaboration leading to timely project completion and high-quality results.
Another advantage of outsourcing data annotators to these providers is the ability to tailor your data annotation efforts according to project requirements. These organizations typically maintain a roster of professionals with a variety of skills (medical annotators, specialists in certain rare languages, etc.), enabling you to quickly increase or reduce the size of your team as required. This flexibility ensures that you always have the right resources at your disposal, without having to maintain a permanent in-house workforce.
Finally, partnering with a reputable third-party data annotation company can help alleviate data security and confidentiality concerns. These organizations often have strict data protection measures in place, ensuring that your sensitive data remains secure and protected throughout the annotation process. By entrusting your data annotation needs to a reliable external partner, you can focus on your objectives with peace of mind.
Beware, however: some of these service providers will offer to lock you into a proprietary, paid-for software solution ("Are you using an open-source platform or in-house development to process your data? It's not efficient, take out a subscription to our solution instead, billed at XXX EUR per user). At Innovatiana, we believe that the best way to produce quality "ground truth" data is to train qualified professionals. While we have our own opinions on the various existing platforms (some features are highly appreciated and influence AI developments), we refuse to accept an overly closed model that would impose the use of one solution rather than another.
Benefits of outsourcing to specialist annotation providers for AI
(+) Instant access to experienced and skilled data annotators
(+) On the whole inexpensive for the level of quality, profitable
(+) Professionally managed annotation projects
(+) High-quality annotation
Disadvantages of outsourcing to specialist annotation providers for AI
(-) Possibility of different views on your IA pipelines
(-) For some service providers, locking of services with proprietary labelling tools (software solutions)
In summary, outsourcing data annotators to third-party companies offers an effective solution for organizations wishing to integrate qualified professionals within a short timeframe. This approach offers many advantages, such as access to first-rate expertise, a well-structured methodology and the ability to tailor resources to project requirements. However, it is essential to carefully weigh up the pros and cons of outsourcing before making a decision, as this method may not be suitable for all organizations or all projects.
On the one hand, outsourcing data annotators can offer significant advantages in terms of cost savings, time efficiency and access to specialist knowledge. By partnering with a reputable third-party company like Innovatiana, you gain access to a vast pool of experienced professionals who have mastered the latest annotation tools and techniques, guaranteeing high-quality results for your projects.
How can I find effective data annotators? Our tips
Below, we've listed 3 ways to find the best data annotators for your AI projects:
1. Use Data Labeling outsourcing specialists
You can contact outsourced data annotation professionals, who have teams of trained and experienced Data Labelers and Data Labeling Managers. This will help you gain rapid access to experienced data annotators and save significant time and resources. Companies such as Innovatiana or Sama specialize in data annotation services and offer first-rate services with a focus on certain geographies.
2. Publish job offers on dedicated platforms
You can post jobs for data annotators on LinkedIn, Indeed, Glassdoor or other popular platforms. This will of course take more time, and is recommended if you have substantial resources and work in sensitive industries (medical, automotive, etc.).
3. Freelance or crowdsourcing platforms
You can search for data annotators on freelance platforms, such as Upwork, Fiverr and similar platforms. You can publish the job requirements or search for data annotators yourself. However, bear in mind that the level of quality may be inconsistent because freelance consultants are potentially poorly trained or oversell their skills to sell work on these highly competitive platforms.
All of the above methods can help you easily find data annotators who match your project's needs. However, be sure to focus on finding data annotators with the right skills by carefully evaluating their expertise and experience.
7 other factors to consider when hiring data annotators
When hiring data annotators, consider the following factors to recruit the best talent:
In conclusion
The role of data annotators has become increasingly important in AI projects. As a result, it's important to recruit the right talent who can lead your AI projects to success. Above, we've discussed in detail how to hire data annotators using various approaches, such as in-house recruitment, the use of freelance services and outsourcing. Choose the approach of your choice and start your search today!
Each approach has its unique advantages, from the dedicated commitment and in-depth understanding of the project offered by in-house data annotators to the professional methodology found in data annotation service providers. By carefully assessing your project requirements, organizational goals and available resources, you can determine the most appropriate approach for your specific AI needs.
As you embark on your search for ideal data annotators, remember that the quality of your annotated data will have a profound impact on the performance and accuracy of your AI models. Therefore, it's essential to prioritize factors such as domain expertise, familiarity with the latest annotation tools and techniques, and excellent communication skills.
A final point that is close to our hearts at Innovatiana is ethics: unfortunately, this is a factor that is often overlooked by certain service providers or platforms. We reject the anti-competitive practices of offering excessively low or non-transparent rates for data annotation services. Such practices conceal working conditions for annotators that are incompatible with our CSR policy.
In summary, the importance of data annotators in shaping the future of AI cannot be underestimated. By following the guidelines and considerations presented in this discussion, you'll be well equipped to make informed decisions and recruit the best talent to drive your AI projects. Choose the approach that matches your objectives and start your search for outstanding data annotators today.