How much do free data labeling tools really cost?

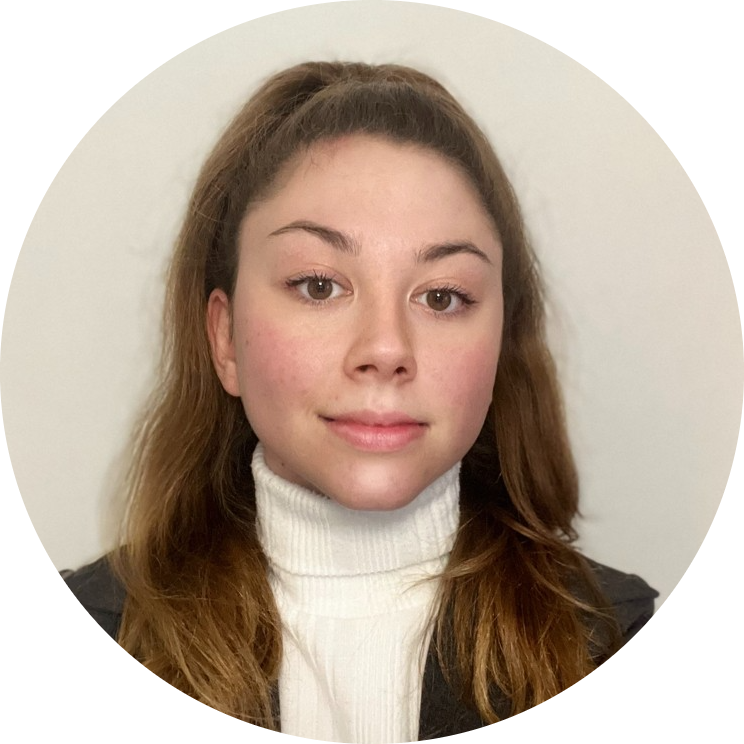
π€ Choosing a data annotation platform: what about "free" solutions?
β
β
The data labeling is an essential step in preparing high-quality datasets for training machine learning models, a cornerstone of AI. This task can be tedious and costly, especially when you opt for paid tools. Fortunately, the market offers a plethora offree data labeling tools that can be of significant help for projects on a limited budget. In this article, we explore the best free data annotation tools, while considering the real costs that can be associated with their use, an important factor in the growth and development of your AI projects.
β
Label Studioan open source data annotation tool, is one of the most popular freeware tools, thanks to its user-friendliness and its ability to handle various types of annotation, a fundamental aspect of annotated data quality. Although Label Studio is free, it offers the quality and precision to handle speech recognition and computer vision, two areas where machine learning has revolutionized technology and the use of data.
β
VGG Image Annotator (VIA) and RectLabel are further examples of data annotation tools that promote the development of accurate computer models, contributing to the development of artificial intelligence. They enable data to be annotated with great precision, including offline versions of the application, which is essential for datasets involving images and videos. These tools offer a way of manipulating objects in a variety of use cases, and thanks to their functionality, they play an essential role in the annotation process for AI.
β
β
An overview of free data labeling tools...
β
1. Label Studio Community
β
Label Studioin its "Community" version, is one of the most popular free data labeling tools. It features a user-friendly interface that allows annotators to easily add tags to different categories of objects in images or videos. This labeling software supports several types of annotation (including image annotations and text annotations), such as bounding rectangles, key points and masks, offering great flexibility for various types of project.
β
Although Label Studio is advertised as free, it's important to note that there are some advanced features that are only available in the paid version. In addition, if your project requires collaboration between several annotators or integration with existing systems, you may encounter difficulties linked to the still imperfect management of concurrent access(at the time of writing). In addition, some versions of Label Studio had problems extracting data in several formats, as well as performance issues.
β
Nevertheless, Label Studio Community remains the most powerful free / open source data labeling software on the market, and is a favorite of many data scientists.
β
β
2. VGG Image Annotator (VIA)
β
VGG Image Annotator (VIA) is an open-source data labeling tool designed by researchers at Oxford University. It can be used free of charge. It offers a simple yet powerful interface for annotating images with bounding boxesmasks and key points. VIA is customizable, enabling users to define their own annotation categories according to the specific needs of their project.
β
However, it's important to note that as VIA is an Open Source solution, itmay require some technical knowledge for installation, configuration and maintenance. If your team has no IT expertise, it may be more advantageous to opt for off-the-shelf solutions, even if they cost money. What's more, its interface may appear dated and put off even the most daring data-labellers.
β
β
3. RectLabel
β
RectLabel is another free data labeling tool that focuses primarily on image annotation. It offers an intuitive user interface that allows image annotators to draw bounding rectangles around objects of interest in images. This tool is particularly popular with Mac users, as it is specially designed for Mac OS systems.
β
However, although RectLabel is free, it's important to remember that this free version may have limitations in terms of the number of annotations or advanced features. If your project requires a large number of annotations or more advanced features, it may be necessary to upgrade to the paid version of RectLabel or explore other alternatives. What's more, as RectLabel was designed for offline annotation, using it can be a challenge when it comes to mobilizing large teams of Data Labelers to work on your most voluminous datasets.
β
β
β
β
β
β
β
While the data annotation platform is important, it is above all theefficiency and quality of the data annotation process that is essential to ensure that the data feeding your machine learning models is of the highest quality. Choosing the right data annotation tool can influence the quality and accuracy of the datasets generated and, consequently, the success of your AI.
β
For example, for speech recognition companies , the quality of annotations is crucial. Accurate annotation of audio data and effective management of different dialects and languages can directly influence the performance of natural language processing models. Similarly, computer vision, applied in technologies such as LiDAR or the development of AI for autonomous vehicles, relies on extremely precise annotation data, where every pixel counts.
β
Free tools can meet these requirements up to a point, but the trade-off often comes in terms of advanced functionality and support for the precise tracking and segmentation of objects in videos (for example: for many free or open source platforms, a semantic annotationis not possible).
β
For data-intensive projects, such as computer vision applications, the ability of tools to manage and store large quantities of data, and to enable efficient collaboration between annotators, becomes a key success factor. The tool V7 Labs (Darwin)for example, although not free of charge, offers advanced image and video recognition capabilities, as well as a highly effective collaborative environment.
β
In the context of machine learning, where data quality is often synonymous with model quality, data annotation tools must offer a balance between accessibility and sophistication. Tools such as Label Studio, VIA and RectLabel, although they may require technical knowledge for installation and maintenance, offer accessibility advantages that are essential for the implementation of a development process and the development of robust AI models.
β
β
Analysis of the real cost of free tools
β
While these data labeling tools are labeled as free, it's important to assess the real costs associated with their use.
β
1. Labour costs
β
One of the main real costs associated with free data annotation platforms is the cost of labor (i.e. the working time of annotators or Data Labelers, sourced via a specialized service provider or via a crowdsourcing platform). Even if the tool itself is free, the task of labeling requires time and human resources. Depending on the size and complexity of your project, you may need to hire qualified annotatorswhich represents a financial investment.
β
2. Storage and bandwidth costs
β
Some free tools may offer limited storage space for your annotated data, or limit bandwidth for downloading or sharing data. If your project requires a lot of storage or generates a lot of data traffic, you may exceed the allocated quotas and have to pay additional fees to increase these limits.
β
3. Training costs for annotators
β
If your project requires specially trained annotators for complex or specialized labeling tasks (as is the case in medicine, with data labelers specializing in medical data), training these annotators may entail additional costs.
β
In addition, theefficiency of the annotation platform has a direct influence on the success of machine learning projects. The integration of cloud services such as AWS S3 can facilitate data storage and sharing, while the use of APIs enables greater interoperability with other systems and software. At the same time, implementing good data management and optimizing workflows are essential to meet the growing demands for high-quality data.
β
4. Lack of on-board collaboration capabilities... provide alternatives
β
Collaboration between team members and platform users is essential, and the annotation tool must support an environment where this synergy is possible. For example, tools such as Kili Technology and LabelBox offer a collaborative interface customized to meet the needs of both companies and users. These features can enable teamwork to facilitate the recognition of specific shapes such as polygons or cuboids in images, or audio-to-text transcription for model formation. NLP.
β
Collaboration on these platforms must enable teams to work together efficiently, taking into account time constraints and production objectives. Free tools can provide a good starting point, but it is often necessary to complement them with paid solutions to match the scale and complexity of projects.
β
In the absence of collaboration functionalities, it becomes necessary to equip oneself with alternatives, whether they be project management tools, scripts for extracting the number of labels produced or the time spent by data labelers on the platform... and all this of course represents a hidden cost!
β
5. Lack of video annotation functions... an obstacle to scaling up
β
In terms of computer vision, platforms such as CVAT can offer invaluable assistance, particularly in use cases involving autonomous vehicles, or more generally in all cases of object detection. Precise annotation of video data is an area where the quality of tools can make a significant difference, enabling in-depth analysis and better understanding of image sequences. However, some platforms are not sufficiently powerful for video annotation, which may be an obstacle to future Computer Vision use cases.
β
β
Ability to meet the specific needs of AI projects
β
The data annotation tool should not only be measured in terms of cost, but also in terms of its ability to meet the specific needs of the project. Companies looking to develop AI models need to consider the full range of features offered by these tools, including their flexibility, scalability and the variety of annotation types they support.
β
1. Choose a solution adapted to your global development and labeling strategy
β
In the global context, where the need for automation and precision in data processing is on the increase, free and open source solutions can offer an inexpensive and efficient solution. However, it is vital to evaluate the various options available on the market, taking into account training requirements, the functionalities required for natural language processing (NLP), pattern recognition, and the specifics of the industry concerned.
β
The adoption of data annotation tools needs to be thought through and aligned with overall business development strategy, taking into account the impact of these tools on data quality and annotator efficiency. Data annotation platforms such as LabelBox, thanks to their user interface, offer not only a better user experience, but also the possibility of integrating advanced functionalities such as object detection and segmentation.
β
2. Choose the right solution for your application (NLP, Computer Vision, etc.).
β
Setting up a robust data annotation system can be a challenge, particularly when it comes to managing the diversity of languages required for NLP cases and quality control functionalities. The expertise of Machine Learning engineers is often called upon to adapt platforms to specific needs, such as adding capabilities for video annotation or developing specialized AI models. Data security is also a major concern, and companies need to ensure the protection of intellectual property as well as data confidentiality.
β
3. Choose a tool that evolves with project needs... adopted and maintained by a large community
β
Finally, it is essential to choose a data annotation tool that will evolve with the needs of the project. Companies need to anticipate increases in volume and ensure that the tool they choose can adapt efficiently. The tool must also be able to integrate with the existing data pipeline, facilitating the deployment of machine learning models and the application of acquired knowledge to new data sets.
β
With this in mind, the annotation platform should be evaluated according to its potential to increase annotator productivity and dataset quality, two factors that are directly linked to the success of machine learning projects. Tools like Label Studio, with their Open Source approach, offer advantages in terms of flexibility and access to a community of developers, which can be a considerable asset for companies looking for customizable solutions.
β
The addition of specific functionalities, such as speech detection for speech recognition applications or classification object classification for computer vision systems, can be important in meeting the specific demands of a project. In addition, the integration of state-of-the-art machine learning methods and the use of advanced algorithms are aspects that can determine the scope and ability of a data annotation tool to deliver reliable and accurate results.
β
β
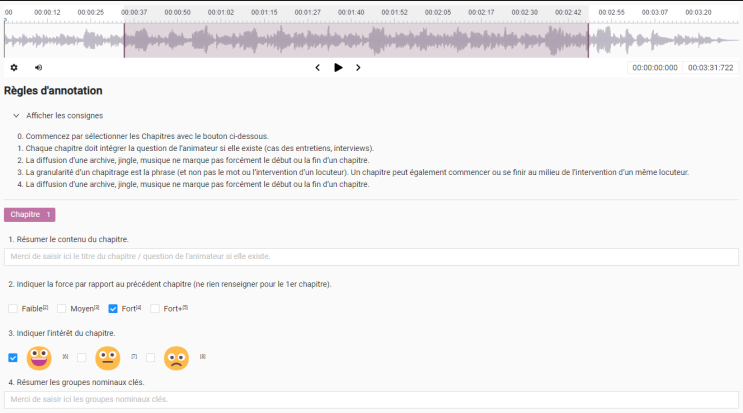
β
β
In conclusion...
β
Free data labeling tools can be of great value for projects with limited budgets. However, it's important to carefully consider the real costs that might arise from using them. The costs of labor, storage, bandwidth and annotator training need to be taken into account when selecting the right labeling tool for your project.
β
Ultimately, as well as considering cost and functionality, it's also important to consider the support and resources available for using these tools, such as tutorials, user forums, and how-to guides. Companies need to assess whether the chosen tool offers a level of support tailored to their needs, enabling the annotation team to work efficiently and unhindered, thus contributing to the overall quality and efficiency of the data annotation process.
β
The perfect solution doesn't exist (yet), so it's up to AI Directors and Machine Learning Engineers to define the best approach to building a solid AI pipeline!
β
β
π The choice of labeling tool will also depend on the specific needs of your project, the size of your team and your overall budget. Take the time to carefully analyze the benefits and costs of each option before making an informed decision for your data labeling project. Once you've chosen the right tool and planned the associated costs, you'll be able to implement an efficient, high-quality labeling process to successfully train your machine learning models.
β
β
Additional resources :
- π https://www.innovatiana.com/post/top-10-image-annotation-platforms-for-ai
- π https://www.innovatiana.com/post/how-to-choose-your-data-labeling-platform
- π https://www.innovatiana.com/post/annotation-partner-vs-crowdsourcing
- π https://www.innovatiana.com/post/what-is-data-labeling
- π https://www.innovatiana.com/post/bounding-boxes-annotation
- π https://www.innovatiana.com/post/natural-language-processing-what-is-it
β