7 criteria to choose your Data Labeling platform

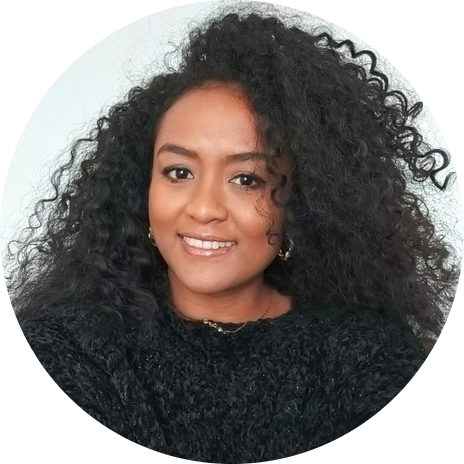
The number of platforms π Data Labeling platforms on the market has never been greater. There are a multitude of technological solutions for annotating data and producing the datasets ("Training Data") that will feed your artificial intelligence models.
β
Yet Data Scientists sometimes tend to neglect their technological setup ("I've been using LabelImg and it's been working for years, why change environment?") even though it can have a direct influence on model results, in a data-centric AI approach.
β

β
β
So, what aspects should you consider before choosing your π Data Labeling platform (or Training Data Platform)?
β
1. The user interface of your Data Labeling platform
β
It is important that the interface is intuitive and easy to use for data labelers. Make sure that the platform offers a clear and simple interface, which allows to work quickly and efficiently. The responsiveness of the interface is also a criterion, as well as the possibility to set up keyboard shortcuts that will save your team of data labelers precious time...
ββ
2. Data labelling functionalities
ββ
Check that the platform you choose meets your needs and requirements in terms of functionality, and in particular the types of annotation you're looking to achieve (Image Labeling or π Video Labeling using π Bounding Box, Polygon, Keypoint, Polyline, Semantic Segmentation, ...). Another feature that is often overlooked is the ability for the administrator or Labeling Manager to precisely monitor the activity of Data Labelers...
β
It's also worth considering the existence of π Active Learning features embedded in the platform. As a reminder, Active Learning is aMachine Learning approach in which a learning model is interactively trained, selecting the most informative learning examples for improving its performance. Some solutions on the market, such as π UBIAI (NLP annotation solution) embed this functionality, enabling pre-annotated data to be presented to a human expert (the Data Labeler) and progressively enriching the learning dataset... and therefore improving the efficiency of the processing of your labeling tasks!
β
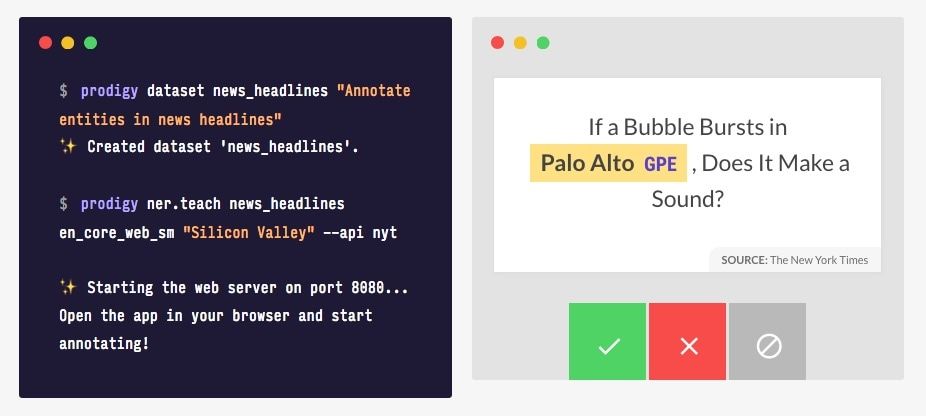
β
3. Data import and export functionalities and extraction format
β
Some platforms allow you to extract labeled data in standard (JSON) or specific (XML, TXT, YOLO, etc.) formats, with varying degrees of success. In the case of certain open-source solutions, data is sometimes "lost" during the extraction process, which can be very time-consuming because it is not optimized. The data import process can also be unintuitive (as in the case of CVAT, which is particularly complex to use when importing pre-annotated data). These are all key points to check before adopting a new tool!
β
4. The support offered by the editor of the Data Labeling solution
β
It's important to ensure that the data labeling platform offers quality support. Don't hesitate to check that the publisher of the labeling solution (SaaS or on-premise) has a team dedicated to the support and requests of users of the AI annotation solution.
β
β
β
β
β
β
5. Costs (Data Labeling platform license fees and costs incurred by the use of Data Labeling Outsourcing)
β
Finally, don't forget to compare the costs of different data-labeling platforms. Many of them appear to be free, but some features represent hidden costs for your company. Some platforms offer a free trial version up to a certain volume of data... with strings attached, i.e. limited functionality or conditions of use/appropriation of your data! Make sure you choose a platform that suits your needs and, above all, your budget!
Finally, some platforms offer on-demand data labeling services... The approach is commendable, but find out how the Data Labelers made available are sourced (are they internal teams, crowdsourced teams, a partnership with an AI and Data Labeling outsourcing specialist like Innovatiana, ...). This is generally a subcontracting process at the initiative of the labeling platform editors, and transparency should be the rule!
β
6. Cloud storage and security
β
It is always tempting to use a SaaS Labeling platform to speed up your labeling process. But also think about your data! Some vendors offer a secure environment and "guarantees"Β (ISO27001 certification, SOC2 report, ...) while others offer trial versions that seem attractive at first sight, with a counterpart: you lose ownership of your data beyond a certain volume! Remember to read the terms of sale carefully before signing a contract, whether you pay or not, with a labeling platform. Of course, this does not apply to all cases of use (some raw data or free datasets obviously do not require special attention to data confidentiality).
β
7. Finally, don't be afraid to use multiple AI labeling platforms!
β
In adata-centric approach to AI (Machine Learning & Deep Learning), if the π data quality is paramount to good results, the Data Scientist should favor theuse of a multitude of platforms depending on use cases. NLP is not the same as π Computer Vision - there is currently no perfectly ergonomic solution for all your developments. So it's up to you to define your own Data Labeling strategy, and this must include prior consideration of tooling!
β
β
TLDR : to sum up, to choose your Data Labeling platform and prepare your Machine Learning data in the right conditions, it' s important to consider the user interface, functionalities, extraction format, support and costs ! You also need to consider the nature of your use case (Computer Vision, NLP, LLM, etc.). Do your research and take the time to compare the various options to find the platform that best suits your needs. We have tested a multitude of platforms and can help you, so don't hesitate to contact us!