Data annotation partner vs. Crowdsourcing: which is the best choice for your AI project?
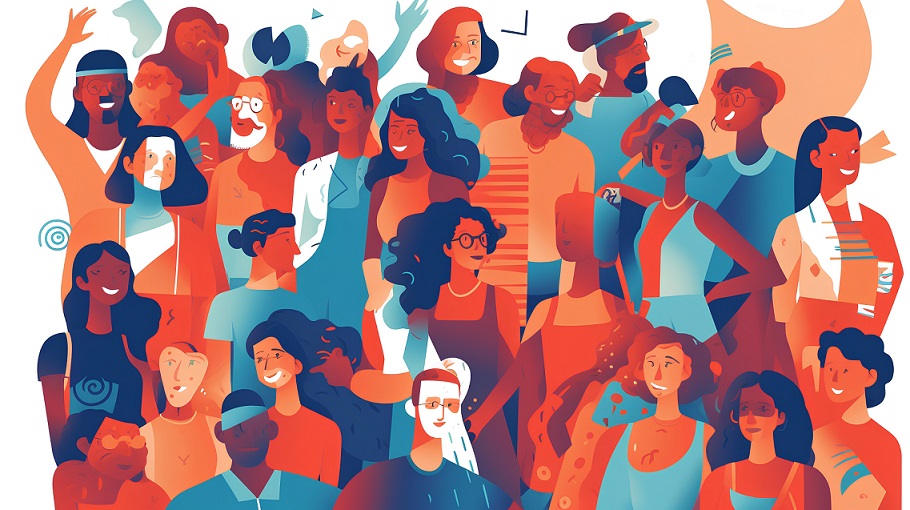
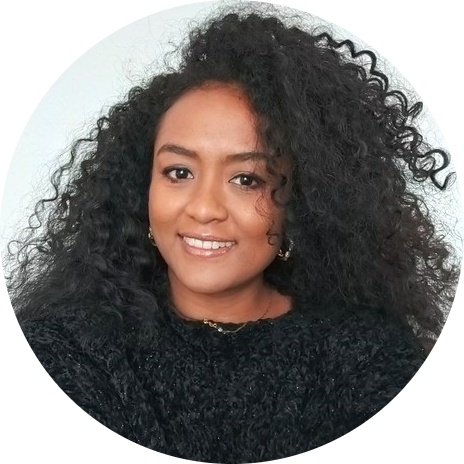
Crowdsourcing has become an increasingly popular means of obtaining data annotations for applications such as natural language processing (NLP) or computer vision. While it can be cost-effective and efficient for accumulating large quantities of labeled data, it also presents risks that potentially increase the total cost of your AI projects.
How is crowdsourcing used for data annotation?
Crowdsourcing data annotation is the process of obtaining labeled data by outsourcing the annotation (or labeling) task to a large group of contributors, usually via an online platform. Contributors are generally anonymous and can come from a variety of backgrounds and levels of expertise. The platforms used by contributors generally offer a user-friendly interface that enables them to access data and annotate it according to predefined criteria, such as labeling objects in images or transcribing speech in audio recordings. The annotations generated by contributors are then aggregated and used to train machine learning models for various applications, such as natural language processing and computer vision.
Annotating data with crowdsourcing: what are the advantages?
Crowdsourcing offers several advantages, including the ability to quickly obtain large quantities of tagged data at relatively low cost. Crowdsourcing platforms can leverage a large number of contributors to annotate data, enabling fast turnaround times and scalability. Crowdsourcing can provide a diverse range of perspectives and expertise, leading to more complete and accurate annotations, and enabling 24/7 annotations, increasing efficiency and reducing turnaround times. It can also promote data transparency and democratize access to digital work, enabling anyone with an Internet connection to contribute to the labeling process, regardless of location or socio-economic status. In any case, this is what is proposed and promoted by these platforms, even if studies have since shown that the jobs created by temporary work platforms contribute more to the casualization of the populations who use them.
Why choose a dedicated partner for data annotation?
Data annotation is a critical step in machine learning. A partner specialized in data annotation (such as Innovatiana) is a company offering services dedicated to AI and data processing. Most of these partners use trained in-house annotators with domain-specific expertise. Because of their industry expertise, training and experience, they generally provide better, more accurate and more consistent data annotations than crowdsourced annotations.
While crowdsourcing data annotation is a popular option among data scientists, there are several reasons why you should consider using a data annotation partner with an in-house workforce:
1. In-depth experience and expertise
Data annotation providers who employ trained annotators have extensive knowledge and experience in the domain-specific tasks they annotate. This expertise ensures that annotations are consistent, accurate and of high quality, which translates into higher-performance machine learning models. What's more, the teams dedicated to your Use Cases provide follow-up services and can intervene on a regular basis, as with any other service provision activity, guaranteeing continuity.
2. Quality control process and SLA
Processes are in place to guarantee annotation accuracy and consistency. For larger orders (several hundred thousand items of data to be annotated), most service providers offer guaranteed SLAs for annotation accuracy.
3. Further training
Data annotation companies generally provide ongoing training and support for their annotators (with in-house training, daily follow-up, internal pathways for data labelers to progress). In the long term, this training and support helps to improve the quality and consistency of annotation work, resulting in more accurate machine learning models.
4. More flexibility and collaboration
Specialists in image, video or text annotation tailor their services to meet specific customer needs, providing data insights via a "Human-in-the-Loop" (HITL) approach and a proactive process to improve the performance of machine learning models.
5. Confidentiality and data security
Data protection regulations require that personal data be protected, and data annotation partners must have strict policies and procedures in place to ensure that data is secure and confidential. Unlike crowdsourcing, these service providers' teams are identified, trained and made aware of information security issues.
What are the 4 main risks of crowdsourcing data annotation?
While crowdsourcing data annotation can be an effective way of obtaining large quantities of labeled data, it presents significant risks - such as inaccuracies, biases, privacy concerns and security issues - that need to be factored into the decision-making process. Here's a brief overview of these risks:
1. Inconsistencies and inconsistent annotations
Crowdsourcing platforms generally rely on a large number of anonymous contributors from various backgrounds, who may not be familiar with the specific field or task. As the tasks are accessible to the greatest number, the level of qualification is not always appropriate, which can lead to a multitude of errors being corrected using a very large number of contributors... which increases costs, and can nonetheless result in inconsistent or inaccurate annotations that can have a significant impact on the quality and reliability of the data used to train AI models.
2. Biased annotations
This can happen when contributors have personal or cultural biases that affect their annotations. For example, someone from a particular cultural background may interpret an image or text differently from someone from another cultural background. This can have a significant impact on the performance of the resulting machine learning models, especially if these potential biases are not qualified before launching the annotation process. For some use cases, it has no impact at all (distinguishing between a cat and a dog is universal!).
3. Difficulties in assessing annotators' performance and avoiding repeating errors
It is often difficult to iterate with crowdsourced annotators, as it can be complicated to manage and coordinate a large number of anonymous contributors. Turnover is also higher as contributors lose interest or move on to other projects, which can lead to delays. It can be difficult to guarantee the quality of annotations by relying on a large, unverified group of contributors with minimal training and no identified functional expertise.
4. Data security and confidentiality
When using anonymous contributors, there is always a risk that a contributor may accidentally or deliberately disclose personal or confidential information, which can have significant legal and ethical consequences. In addition, crowdsourcing annotators use their own hardware and infrastructure, which can lead to security breaches if they don't have appropriate anti-virus software or if they don't regularly update or patch their machines and applications consistently.
5. Crowdsourcing ethics
The use of crowdsourcing for data annotation raises significant ethical concerns. There is a risk of exploitation of contributors, who are often paid minimal compensation for their work, which may not reflect the true value of their contributions to artificial intelligence projects. Furthermore, the anonymity of contributors in crowdsourcing can lead to issues of accountability and quality, as it is often difficult to guarantee that annotations are carried out ethically and accurately. The ethics of crowdsourcing for data annotation depend on how it is managed, and on protecting the rights and dignity of workers and data security, which requires appropriate oversight and regulation to ensure ethical practices in this area.
In conclusion...
Using a data annotation partner offers several advantages, including higher quality annotations, greater flexibility and collaboration, and a "Human-in-the-Loop" (HITL) approach at scale. When choosing a data annotation partner, it's important to take into account its specific functional expertise, its quality control process, its privacy and security policy, and its ability to customize its services to meet your most specific needs.
Why choose Innovatiana to annotate your data and accelerate the development of your AI products?
Innovatiana offers leading data annotation solutions thanks to our ethical approach to AI, our experience and our functional expertise. We have developed a methodology for training annotators (or Data Labelers) and creating the most advanced training data, highly focused on functional application domains (medicine, architecture, legal, real estate, etc.). We do this while maintaining a strong commitment to building an ethical AI Supply Chain! Find out more here.