How the COCO dataset accelerates AI developments

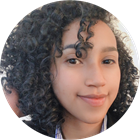
In the constantly evolving field of artificial intelligence, advances often depend on the availability of high-quality, usable datasets. Among the resources available free of charge, the COCO Dataset is a cornerstone for experimentation and development in the field of computer vision and machine learning.
β
Among the datasets available, the COCO Dataset encompasses a database of labeled images designed specifically to train machine learning programs. It's a goldmine of annotated information, offering researchers and AI developers a detailed perspective on the visual world around us. Across thousands of images, this dataset provides a diversity of scenes, contexts and objects, from urban landscapes to domestic interiors, from animals to consumer products.
β
π‘ To access the COCO Dataset, you can visit the official website, where it can be downloaded in various formats. At this address, you can also get more information about the dataset and its creators.
β
β
What is COCO Dataset and what are its key components?
β
The COCO dataset, also known as MS COCO(Microsoft Common Objects in COntext), is a standard reference in the field of computer vision and machine learning, particularly for object detection and segmentation tasks. It was created by Microsoft in collaboration with several academic institutions.
β
The essential components of the MS COCO data set include the following:
β
Various images
The COCO Dataset contains a collection of over 200,000 images covering a wide variety of scenes and objects. Coming from a variety of sources, these images are diverse in terms of resolution, context and complexity.
β
Object annotations
Each image from the MS COCO dataset is accompanied by annotations (or metadata) detailing the locations and categories of objects present in the image. These annotations are often used for supervised learning in object detection and segmentation tasks. In addition, annotations of key points in the dataset enrich the possibilities of computer vision applications, notably for key point estimation, image captioning and panoptic segmentation.
β
Object categories
The COCO Dataset covers 80 different object types, ranging from common objects such as people, cars and animals, to less common objects such as furniture and tools. This diversity enables AI models to be trained to detect a wide range of objects in a variety of contexts.
β
Captions or Subtitles
In addition to object annotations, some parts of the MS COCO dataset include textual descriptions (or"captions") associated with each image. These captions provide additional information on the image content and are often used in image comprehension and automatic description generation tasks.
β
Semantic segmentation
Some versions of COCO Dataset also provide semantic segmentation masks. semantic segmentation for each object. In addition, this dataset includes annotations for instance segmentation, thus enriching application possibilities in the field of computer vision. This makes it possible to precisely delineate the contours of objects in images.
β
β
β
β
β
β
β
β
What's the difference between annotations and subtitles?
β
Annotations and captions are two types of metadata used in the context of image and video analysis, but they have different purposes:
β
Annotations
Annotations are structured metadata describing the specific characteristics of an element in an image or video. In the context of the MS COCO dataset, annotations of various objects are examples of annotations.
β
They indicate the location and nature of objects in an image. Object annotations are often used for tasks such as object detection and object detection and segmentationwhere the model needs to identify and locate different objects in an image.
β
Subtitles
Subtitles are text descriptions associated with visual elements, such as images or video sequences. In the COCO Dataset, subtitles are examples of text descriptions associated with each image.
β
Captions are generally used to aid human understanding of the image or video, as well as to train machine learning models to generate automatic descriptions of visual content.
Β
In short, annotations describe the specific visual characteristics of objects in an image, while subtitles provide more general textual descriptions of the image's visual content.
β
β
How is the COCO Dataset used to train artificial intelligence models?
β
The COCO Dataset is widely used for training artificial intelligence models, particularly in the field of computer vision. Its contribution is important for Computer Vision research, facilitating research on object instance segmentation, particularly for the model training process. YOLO model training process, and the advancement of algorithms and techniques used in computer vision.
β
Object detection
MS COCO object annotations are used to train object detection models. These models are able to identify and locate different objects in an image. This is often done using convolutional neural network (CNN).
β
Semantic segmentation
Object annotations also provide information about the contours of each object in an image. This is used to train semantic segmentation models. These models assign a semantic label to each pixel in the image, enabling the image to be segmented into different object classes.
β
Image classification
The object categories in the COCO dataset can be used to train models for image image classification. These models are able to classify an image into one of the predefined types or categories according to its visual content.
β
Generate image descriptions
Subtitles from the MS COCO dataset can be used to train automatic description generation models for images. These models learn to generate textual descriptions that describe the visual content of an image naturally and accurately.
β
Learning transfer
Given the size and diversity of the COCO dataset, it is often used as a data source for training transfer. Models pre-trained on this dataset can be fine-tuned on specific tasks with smaller or more specialized datasets.
Β
By combining these different approaches, the MS Coco dataset provides a solid basis for training artificial intelligence models in various fields of computer vision.
β
β
Does the MS COCO dataset offer better object recognition than other datasets?
β
The MS COCO is one of the most widely used and recognized datasets in the field of Computer Vision, particularly for object detection and semantic segmentation tasks. The evaluation of models trained on the COCO dataset is often used to measure their performance and robustness, particularly with regard to average precision (AP) and average recall (AR) across different object sizes and levels of overlap. It offers several advantages that make it an attractive choice for object recognition:
β
Size and diversity
As previously mentioned, the COCO dataset contains several thousand annotated images with over a million objects in 80 different categories. This large size and diversity enables us to train more robust models capable of generalizing to a wide range of scenarios and contexts.
β
Precise annotations
Object annotations in the MS COCO dataset are renowned for their accuracy and completeness. Each object is annotated with a bounding box and a corresponding category label. This guarantees rich information for model training.
β
Variety of scenes and objects
The MS COCO dataset covers a wide variety of scenes and objects, including common and less common objects in a variety of contexts. This wide variety makes it possible to train models capable of recognizing and locating different types of object under a variety of conditions.
Β
It is important to note, however, that the "best" object recognition often depends on the specific context of the application and the expected performance requirements of the model. Admittedly, the MS Coco dataset is widely used and offers many advantages... however, it can be limited in very specific contexts.
β
By way of example, there are other data sets specialized in a particular field, which may be better suited to certain applications. These include ADE20K for semantic segmentation, Cityscapes for object recognition, and PASCAL VOC for object detection in images.
β
β
Ultimately, the choice of dataset will depend on the specific needs of the project and the desired performance! While MS COCO is an excellent starting point for experimenting and training models on simple cases, it is likely to prove insufficiently comprehensive for training your most complex models or those requiring highly specific data!
β
β
Conclusion
β
The COCO dataset has already had a significant impact on artificial intelligence for several years, particularly in the field of computer vision. However, several future developments are expected around this dataset, potentially enhancing its impact on artificial intelligence. Future developments around the COCO dataset are likely to focus on several main areas. These include:
- An increase in size and diversity;
- Improved annotation quality;
- Expansion into new fields of application (such as human action recognition or sentiment detection in images, as well as multimodal data integration).
β
These developments should strengthen the impact of the COCO dataset on artificial intelligence, providing richer training data and opening up new prospects for innovative applications in computer vision and beyond. In the meantime, you can always contact us We can enrich the COCO Dataset for you, or even better, build a customized dataset to meet your most specific needs!