Argilla: the ultimate tool for creating quality datasets for your LLMs?
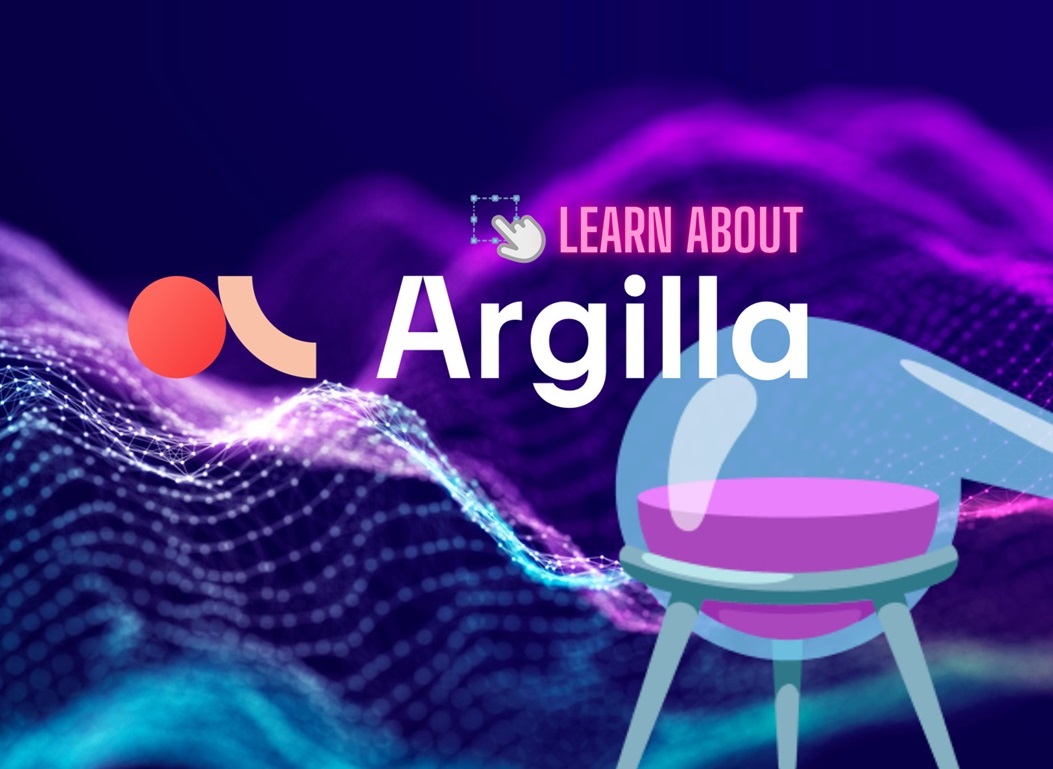
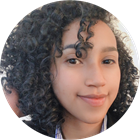
β
β
β
π‘ This article explores the features and benefits of this innovative tool, as well as its potential impact on improving the performance of AI models.
β
β
β
π€― BREAKING NEWS(17.09.2024) - Argilla has just published "DataCraft", an interface using Distilabel to create synthetic datasets! You can test the tool at this address (https://huggingface.co/spaces/argilla/distilabel-datacraft), and if you'd like to review, enrich or complete your dataset with expert help, please don't hesitate to contact Innovatiana !
β
β
β
What is Argilla and what role does it play in data annotation?
β
Argilla is a data annotation platform designed to simplify and improve the process of creating high-quality datasets, essential to the development of artificial intelligence (AI) models.
β
It stands out for its ability to manage large amounts of data, while offering collaboration tools and advanced features for customizing annotations to specific project needs.
β
β
β
β
β
β
β
β
β
β
β
How does Argilla differ from other data annotation tools?
β
Intuitive, customizable user interface
The latest version of Argilla features a user interface designed to be both intuitive and flexible, acting as a central hub for annotation management. New features in Argilla's user interface include enhanced functionality for a better user experience. Unlike many other tools, it enables extensive customization of text annotations, adapting perfectly to the specifics of each project.
β
This flexibility is essential to meet the varied needs of artificial intelligence projects, which may require very specific types of annotation.
β
Facilitated collaboration for efficient teamwork
β
Machine Learning-driven annotation
Argilla also innovates with its hybrid approach to annotation, combining human expertise with Machine Learning models. This feature enables annotations to be suggested based on pre-trained models, speeding up the process and increasing dataset accuracy. This represents a significant time-saving while improving annotation quality.
β
Seamless integration into a development environment (Python)
Last but not least, Argilla stands out for its ability to integrate easily into a variety of development environments, particularly those based on the Python library. This compatibility enables users to continue working in their familiar environments, while taking advantage of Argilla's ability to set up powerful data annotation workflows.
β
β
πͺ Argilla is a particularly valuable tool for development teams looking to optimize their dataset creation workflow without disrupting their work habits.
β
β
List of data types that can be annotated with Argilla
β
Argilla is designed to be a versatile tool, capable of handling a wide range of data types. Here's an overview of the main data types that can be annotated with Argilla:
β
Text
β
Sequential and temporal data
For projects requiring the annotation of sequential or temporal data, Argilla offers tools adapted to the annotation of data sequences. This includes applications such as time series labeling, annotation of sensory data streams, or video analysis.
β
Multimodality
Argilla is also capable of handling multimodal datasets, where several data types (text, image, audio) are combined. This enables consistent annotation across different media types, which is essential for complex projects integrating multiple data sources.
β
Structured data
Finally, Argilla can be used to annotate structured data, such as tables or databases. This is particularly useful for projects requiring the tagging of specific features or the creation of datasets from structured data sources.
β
β
Distilabel: A powerful Argilla extension for dataset enhancement
β
β
How does Distilabel work?
Distilabel is based on advanced knowledge distillation algorithms, where a pre-trained model ("teacher") is used to generate annotations for unlabeled data. These annotations are then reviewed and validated by human annotators, creating a feedback cycle that continually improves dataset quality. This hybrid approach not only saves time, but also reduces the costs associated with manual annotation, while maintaining a high level of accuracy.
β
The benefits of Distilabel for AI projects
One of Distilabel's key advantages is its ability to handle massive volumes of unlabeled data, transforming them into valuable resources for model training. This extension is particularly useful for projects requiring extremely large datasets, such as those involving natural language processing (NLP) or computer vision models. What's more, Distilabel integrates seamlessly with Argilla, offering a unified interface to manage the entire annotation process, from data collection to final labeling.
β
β
How does Argilla improve dataset quality for training artificial intelligence models?
β
β
AI-assisted annotation
Argilla integrates Machine Learning models to assist annotators by suggesting annotations based on automated predictions.
β
This hybrid approach not only saves time, but also improves the consistency and accuracy of annotations, reducing human error. Suggestions provided by AI are then validated or adjusted by human annotators, ensuring a balance between automation and quality.
β
Quality control and validation of annotations
An essential aspect of Argilla is its integrated quality control system. Annotations can be reviewed, validated or corrected by other team members, ensuring double-checking of annotated data. This collaborative process reduces individual bias and improves data reliability.
β
Flexible, customizable annotation workflows
Argilla lets you create customized annotation workflows, tailored to the specific needs of each project. This flexibility ensures that annotations are performed according to precise criteria, corresponding to the requirements of the AI model to be trained.
β
The ability to define annotation schemes in detail helps to standardize the process, which is essential for consistent, high-quality datasets.
β
Easier collaboration for greater consistency
Argilla's collaborative features enable multiple annotators to work simultaneously on the same dataset. This collaborative approach enhances annotation consistency, as annotators can share feedback in real time, discuss ambiguous cases, and harmonize their annotation practices.
β
Centralizing annotations in a shared environment also helps maintain high quality throughout the dataset.
β
Real-time analysis and feedback
Finally, Argilla provides real-time analysis tools to monitor annotation progress and quickly identify any inconsistencies or errors. Argilla offers valuable insights into the quality of data being created, enabling immediate adjustments if necessary. Continuous analysis improves the efficiency of the annotation process and ensures that the final dataset meets the quality standards required for training AI models.
β
β
What are the main use cases for Argilla in AI model development?
β
Argilla is used in a variety of use cases in the development of artificial intelligence (AI) models, particularly where data annotation plays a major role in training and improving model performance. Here are some of the main use cases:
β
Time series annotation
Argilla is proving useful in the annotation of sequential and temporal data, such as time series. This includes applications in fields such as finance, where AI models need to analyze historical data to predict future trends, or in medicine, for the analysis of biometric data.
β
The ability to annotate and manage sequential data efficiently means that robust datasets can be created for these types of models.
β
Multimodal projects
Projects requiring the integration of multiple data types (text, image, audio) also benefit from Argilla. Multimodal annotations are often complex, and Argilla makes it possible to manage them consistently, ensuring that annotations from different data types are aligned.
β
This is particularly useful in advanced applications such as context recognition or the creation of interactive systems where several types of media need to be processed together.
β
Creating and managing knowledge bases
Argilla is also used to annotate structured data, such as tables or databases, which is essential for applications such as recommendation systems, knowledge management and data analysis.
β
These annotations help to structure information in a way that is useful for training AI models that depend on organized, interconnected data.
β
β
Conclusion
β
Argilla has established itself as an essential tool in the field of artificial intelligence, offering advanced solutions for data annotation, an important aspect of high-performance model development.
β
Thanks to its flexibility, seamless integration into various development environments, and innovative features such as AI-assisted annotation, Argilla enables teams to create high-quality datasets more efficiently and collaboratively.
β
Whether for natural language processing projects or other Machine Learning applications, Argilla stands out for its ability to meet the complex needs of annotators and developers.
β
Ultimately, the use of Argilla not only improves data quality, but also represents a significant advance in the reliability and accuracy of AI models, contributing to the success of large-scale AI projects. Just goes to show... it's still possible to innovate in the world of Data Labeling!