Sentiment analysis: AI-accelerated technology revolutionizing modern marketing
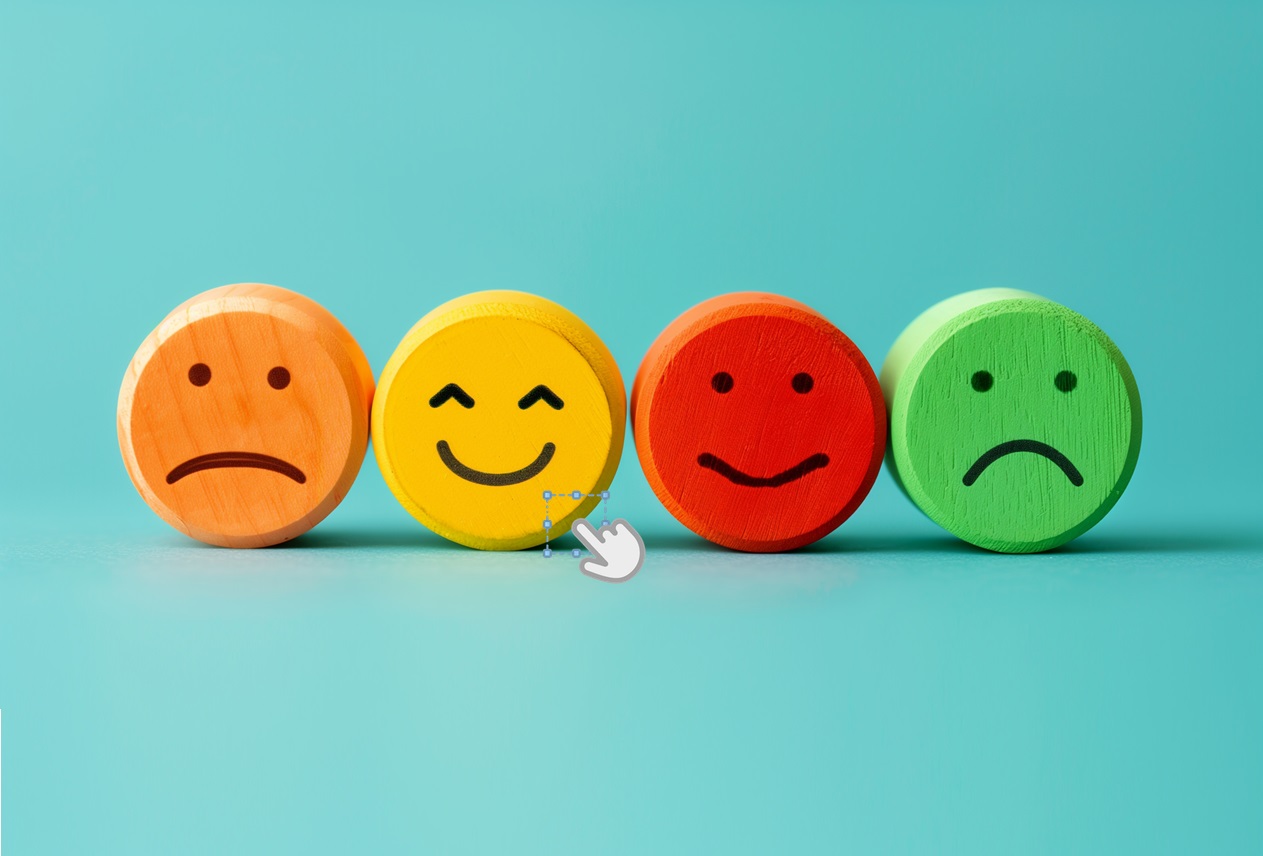
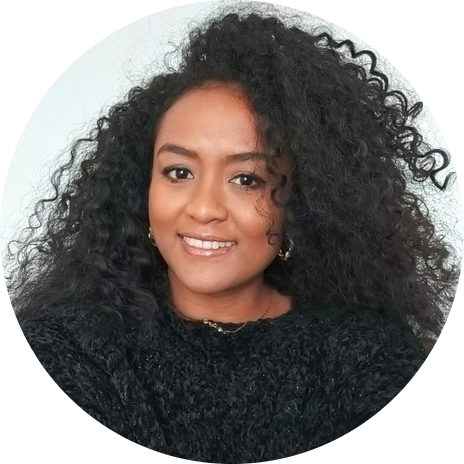
Sentiment analysis is a fast-growing technology. It enables us to understand the emotions and opinions expressed in textual data. Using advanced algorithms and π natural language processing or NLP, this technology offers valuable insights . Among its main use cases, it has become essential for companies seeking to optimize their marketing strategies. But is it its only application? In this article, we'll explore the various applications of sentiment analysis.
β
In a world where consumers share their opinions continuously, on social networks and online platforms, sentiment analysis helps to capture market trends and sentiments in real time. It transforms the way companies interact with their customers, enabling them to respond in a more personalized and effective way.
β
The impact of sentiment analysis on modern marketing is immense. It doesn't just provide data, but offers profound insights that influence strategic decisions. Companies that embrace it and know how to exploit it can better understand their consumers, anticipate their needs and create more engaging experiences.
β
β
π‘ Sentiment analysis is therefore more than just a tool. It's a revolution that's redefining the rules of marketing and opening up new opportunities for businesses of all sizes. Eager to learn more about this new technology and how it's being used to understand customer emotions and attitudes? We'll tell you all about it!
β
β
β
What is sentiment analysis?
β
Sentiment analysis, also known as "sentiment analysis", is first and foremost a field of artificial intelligence (AI) and natural language processing (NLP). It aims to identify and extract emotions and opinions expressed in writing.
β
As previously mentioned, it is commonly used to analyze customer reviews, comments on social networks, product reviews, and other forms of online textual communication. It's a technique for assessing an author's emotional attitude towards a specific subject. This attitude can be positive, negative or neutral.
β
Simply put, it helps us to understand what people are feeling and thinking based on their writings. Companies use this technology to gain valuable insights into consumers' perceptions and feelings about their products, services or brands.
β
Indeed, sentiment analysis enables companies to understand and interpret consumer emotions in a thorough and accurate way. By harnessing this information, they can make informed strategic decisions to improve customer engagement and strengthen their market position.
β
β
β
β
β
β
β
β
How does sentiment analysis work?
β
Sentiment analysis works in several stages, involving various techniques and algorithms.
β
1. Data collection
Textual data is collected from a variety of sources, such as social networks, websites and forums, blogs, and online reviews. This data can be structured (such as questionnaires) or unstructured (such as online comments).
β
2. Data pre-processing
Before analyzing the natural language in a text, it is essential to clean and prepare it. This includes removing unnecessary words(stop words), normalizing words(lemmatization or stemming), and correcting typos.
This work can be carried out by annotators, or Data Labelers, whose mission is to manually review data sets to improve their quality, or in some cases to annotate them using tools such as π Prodigy or π UbiAI.
β
3. Feature extraction
Relevant features are extracted from the text for analysis. These may include individual words, n-grams (groups of words), or syntactic and semantic aspects of the text.
β
4. Classification of feelings
Machine Learning algorithms or NLP (Natural Language Processing) techniques are used to classify text into different sentiment categories. Various sentiment analysis tools are used to classify text into different sentiment categories. The most common models include logistic regression models, support vector machines (SVMs), and deep neural networks (such as LSTMs and Transformers).
β
5. Analysis and interpretation
The results of the analysis are interpreted to draw conclusions. Companies can use these insights to improve their products, adjust their marketing strategies, train their customer service, and respond more effectively to consumer needs.
β
β
What techniques are used in sentiment analysis?
β
Sentiment analysis uses a variety of techniques to understand and interpret the emotions and opinions expressed in textual data, including :
β
Feelings glossaries
Sentiment lexicons are dictionaries of words or phrases associated with specific emotions. To capture specific emotional nuances, these lexicons need to be adapted to different languages. Each word or phrase is assigned a sentiment score (positive, negative or neutral). This method is simple and quick to implement, but it can lack nuance, as it does not take context into account.
β
Supervised learning
Supervised learning involves training machine learning models is based on rules of π labeled data. These labeled data are consituted in datasets generally made by Data Labelers or professional annotators. The models learn from the examples in the datasets to predict the sentiment of new texts. Commonly used algorithms include :
- Logistic regression ;
- Support vector machines (SVM) ;
- Decision trees ;
- Random forests ;
- Naive Bayes.
β
Unsupervised learning
Unsupervised learning techniques do not use labeled data. They identify structures or patterns in the data without explicit supervision. Common methods include :
- Clustering :
Techniques like K-means or DBSCAN for grouping similar texts.
- Principal component analysis (PCA) :
Dimensional reduction to identify the main emotional components in texts.
β
Neural networks
Neural networks, and Deep Learning networks in particular, are being used to capture complex nuances in the sentiments expressed in texts.
β
Common approaches include :
- Convolutional neural networks (CNN) :
Used to extract local features from text.
β
- Recurrent neural networks (RNN) and Long Short-Term Memory (LSTM) :
Ideal for processing text sequences and capturing long-term dependencies.
β
- Transformers :
Models like π BERT (Bidirectional Encoder Representations from Transformers) and GPT (Generative Pre-trained Transformer) that use attention mechanisms to understand the global context of words in a text.
β
Hybrid models
Hybrid models combine several techniques to improve the accuracy of sentiment analysis. For example, a model might use a sentiment lexicon for a preliminary analysis, then refine the results with a supervised machine learning model.
β
Aspect-based Sentiment Analysis (ABSA)
This technique goes beyond simple sentiment classification by analyzing sentiments relating to specific aspects of the product or service. For example, a review can be analyzed to determine feelings about product quality, customer service and price.
β
Rule-based approaches
These approaches use predefined rules to determine sentiment. The rules can be based on regular expressions, syntactic patterns, or specific word combinations.
β
Word Embeddings
Word Embedding techniques like π Word2Vec, π GloVeand π FastText represent words in a vector space to capture their contextual meanings. These vector representations are then used in Machine Learning models to analyze sentiment.
β
β
π‘ By combining these different techniques, sentiment analysis becomes more accurate and effective, enabling companies to better understand their customers' opinions and emotions. This helps to make more informed decisions and improve marketing strategies and customer engagement.
β
β
How can sentiment analysis improve customer engagement?
β
Sentiment analysis plays a key role in improving customer engagement by enabling companies to better understand and respond to their customers' emotions and opinions. Here's how this technology can enhance customer engagement:
β
Personalized interactions
Sentiment analysis helps companies understand specific customer sentiments from the perspective of their interactions on social networks, online reviews and direct feedback. Using this information, companies can personalize their responses to show that they care about each customer's individual concerns. An empathetic response to a negative comment, or an acknowledgement of positive feedback, can strengthen the customer relationship.
β
Enhanced responsiveness
By monitoring customer sentiment in real time, companies can react quickly to negative comments and reviews. This responsiveness shows consumers that their opinions are taken seriously, and that the company is committed to solving their problems, thereby improving customer satisfaction and loyalty.
β
Improving products and services
Insights gained from sentiment analysis can reveal specific aspects of products or services that appeal to or displease targets. By making improvements based on this feedback, companies show that they are listening to their customers and are committed to providing solutions that better meet their needs. This reinforces customers' trust and commitment to the brand.
β
Proactive communication
Sentiment analysis identifies emerging trends in opinions and feedback. Companies can use this information to proactively communicate the actions they are taking to address customer concerns. For example, if a recurring problem is identified, proactive communication about the steps being taken to remedy it can improve brand perception and encourage positive dialogue with consumers.
β
Effective segmentation and targeting
By segmenting customers according to their feelings, companies can better target their marketing campaigns and communications. For example, customers expressing positive feelings can receive loyalty offers or special thanks, while those with negative feelings can be targeted with repair offers or surveys to better understand their issues. This allows each segment to be engaged in an appropriate and personalized way.
β
Optimizing marketing campaigns
Sentiment analysis enables the emotional impact of marketing campaigns to be assessed in real time. Companies can adjust their campaigns based on customer reactions to maximize positive impact and minimize negative reactions. This helps create more effective campaigns and engage customers in a more meaningful way.
β
Monitoring customer satisfaction and strengthening customer relations
By constantly monitoring customer sentiments, companies can gain a continuous view of customer satisfaction. This enables changes in customer sentiment to be identified and corrective action taken quickly.
β
Regular monitoring helps to maintain a high level of customer satisfaction and avoid problems before they become critical. Sentiment analysis helps identify the most engaged customers and those requiring special attention.
β
By recognizing and rewarding loyal customers, companies can strengthen their relationship with these customers. Similarly, by proactively engaging with less satisfied customers, companies can demonstrate their commitment to improving their experience, which can turn disgruntled customers into brand advocates.
β
β
How does sentiment analysis influence the creation of marketing content?
β
Sentiment analysis significantly influences the creation of marketing content by providing detailed insights into consumer preferences, emotions and opinions.
β
Identifying consumer preferences
Sentiment analysis enables us to understand which types of content and messages resonate best with readers.
β
By analyzing customers' emotional reactions to various types of content (articles, π videossocial media posts), marketers can identify themes and formats that elicit positive reactions. This makes it possible to create content that is more likely to captivate and satisfy the target audience.
β
Adjusting tone and style
The insights gained from sentiment analysis help content creators to adjust the tone and style of their communication to better match customer expectations and preferences. For example, if analysis reveals that customers respond favorably to a friendly, informal tone, content can be adapted accordingly to maximize engagement.
β
Rapid response to trends and events
Real-time sentiment analysis enables companies to react quickly to emerging trends and current events. By monitoring discussions and sentiments on social networks and other online platforms, companies can create and deliver timely, relevant content that captures the attention of their audience and aligns with current conversations.
β
Personalized content
Sentiment analysis facilitates content personalization by providing detailed information on audience segments.
β
By understanding the specific sentiments of different customer groups, marketers can create tailored content that addresses the unique needs and preferences of each segment. This personalization improves content relevance and increases engagement.
β
Optimizing advertising campaigns
Advertisements can be optimized according to the sentiments expressed by consumers. By analyzing reactions to past advertising campaigns, companies can identify what works well and what needs to be improved. Advertising messages can be adjusted to maximize positive reactions and minimize negative ones, improving campaign effectiveness.
β
Brand reputation management
Sentiment analysis helps monitor and manage brand reputation online. By detecting negative sentiment or criticism, companies can create content to address customer concerns, correct misunderstandings, and reinforce the positive aspects of the brand. This helps maintain a positive image and manage potential crises before they escalate.
β
Assessing the impact of content
Sentiment analysis is used to assess the emotional impact of existing content. By measuring emotional reactions to blog posts, videos, social media posts, etc., marketers can determine which content generates the desired impact, and which requires adjustment. This enables continuous optimization of content to better meet consumer expectations.
β
Creating engaging and interactive content
Insights into consumer sentiment can inspire the creation of more engaging and interactive content. For example, if analysis shows that customers appreciate interactive content such as quizzes, polls or live video, marketers can incorporate these elements into their content strategy to boost engagement.
β
Predicting future needs
By analyzing consumer trends and sentiments, companies can anticipate future needs and expectations. This enables them to create proactive content that not only meets current needs, but also sets the scene for future trends. This positions the company as an opinion leader and innovator in its sector.
β
β
How to measure the effectiveness of sentiment analysis in marketing strategies?
β
Measuring the effectiveness of sentiment analysis in marketing strategies is crucial to understanding its impact and optimizing future efforts.
β
Monitoring specific KPIs
β
Net Sentiment
Compare the volume of positive sentiment to the volume of negative sentiment. An increase in Net Sentiment after the implementation of strategies based on sentiment analysis indicates an improvement in brand perception.
β
Commitment rate
. On social networks: Measure likes, shares, comments and mentions before and after using sentiment analysis.
. On content: Track views, shares and click-through rates (CTR) on site or blog articles, newsletters and other marketing content.
β
Customer satisfaction rate (CSAT)
Compare customer satisfaction scores before and after the integration of sentiment analysis into communication strategies.
β
Net Promoter Score (NPS)
Measure whether customers are more likely to recommend the brand following personalized campaigns based on sentiment analysis.
β
Analysis of customer feedback and opinions
. Review volume and quality: Compare the number and positivity of customer reviews on review platforms (such as Yelp, Google Reviews) before and after implementation.
. Recurring themes : Identify whether recurring topics of satisfaction or dissatisfaction are evolving favorably.
β
Evaluating the performance of marketing campaigns
. Conversion rate: Measure the increase in conversions (purchases, registrations, etc.) after marketing campaigns optimized by sentiment analysis.
. Campaign ROI: Calculate the return on investment of campaigns based on sentiment analysis compared with those that are not.
. Feelings before and after campaigns: Analyze the feelings expressed before and after a campaign to assess its emotional impact.
β
Customer engagement and loyalty
. Retention rate: Compare the customer retention rate over a given period before and after the use of sentiment analysis.
. Customer Lifetime Value (CLV): Measure whether sentiment analysis has led to an increase in customer lifetime value by strengthening loyalty.
β
Monitoring social networks
. Volume and tone of mentions: Track the number of brand mentions and their tone on social networks to detect post-implementation changes.
. Sentiments by topic: Use sentiment analysis to identify whether discussions around certain products or services are improving.
β
A/B tests
. Controlled experiments: Conduct A/B tests to compare the performance of campaigns or content created with and without the use of sentiment analysis.
. Variant results : Analyze performance differences between variants in terms of click-through rates, engagement and conversions.
β
Integration with CRM and marketing automation tools
. Interaction analysis: Integrate sentiment analysis data into CRM to assess the impact on customer interactions and sales.
. Customized automation: Measure the effectiveness of sentiment-based automated campaigns (emails, notifications) in terms of open rates, clicks and conversions.
β
Case studies and customer testimonials
. Qualitative feedback: Gather customer testimonials and case studies to obtain qualitative feedback on the impact of sentiment analysis.
. Perceived satisfaction: Analyze customer feedback on the relevance and impact of personalized communications.
β
β
How can sentiment analysis evolve?
β
Sentiment analysis continues to evolve as new technologies emerge and the needs of businesses and consumers change. In the next few years, it's not impossible that this technology will be more mature, and therefore more effective in many respects, thanks to improved Machine Learning :
β
Improved accuracy thanks to Deep Learning
- More advanced language models: The development of even more advanced language models, based on deep learning and transformers such as GPT (Generative Pre-trained Transformer), will enable a finer understanding of context and nuance in textual data, improving the accuracy of sentiment analysis.
β
- Continuous learning: Deep learning models will continue to be trained on large datasets to improve their ability to understand the subtleties of natural language and adapt to changing linguistic expressions, processes and cultural trends.
β
Multimodal analysis
We're talking here about a combination of textual, visual and audio data. Integrating sentiment analysis with other data modalities, such as images, videos and audio, will enable a more accurate and in-depth understanding of users' emotions and opinions. This could open up new possibilities for applications in social media, advertising and brand monitoring.
β
Cultural adaptation
Progress in understanding cultural and linguistic differences will enable sentiment analysis systems to adapt more effectively to the linguistic and cultural peculiarities of different regions of the world. This will make sentiment analysis more accurate and relevant on a global scale.
β
Real-time sentiment analysis
Companies will be looking to integrate real-time sentiment analysis into their social media monitoring tools. This would enable them to quickly detect and react to emerging trends, potential crises and customer engagement opportunities.
β
Taking the context into account
Future sentiment analysis systems will be able to better understand the context in which expressions are used. This will enable more accurate interpretation of users' feelings and intentions. This will reduce the risk of misinterpretation and improve the quality of the insights generated.
β
Privacy and ethics
Future developments in sentiment analysis will focus on protecting user privacy by ensuring that personal data is handled responsibly and in compliance with data protection regulations.
β
Advanced customization
Companies will use sentiment analysis to offer more personalized experiences, tailored to users' emotions and preferences. This, by offering content, products and services that better meet their emotional needs.
β
Integration with conversational AI
Integrating sentiment analysis with conversational AI technologies, such as chatbots and virtual assistants, will enable more empathetic and personalized interactions. This will enhance the user experience while strengthening customer relationships.
β
β
Conclusion
β
In conclusion, sentiment analysis or sentiment analysis in AI offers a diverse range of applications that enable companies to better understand their customers and enhance their engagement. By harnessing the insights generated by sentiment analysis and natural human language, companies can adapt their strategies to respond more effectively to consumers' needs and emotions, creating more relevant, personalized and satisfying experiences.
β
As sentiment analysis continues to evolve with the advent of new technologies and the emergence of new challenges, it's clear that its importance in the marketing landscape will only grow. However, it is important to remain mindful of the ethical and privacy issues associated with the use of this technology, to ensure that its deployment is carried out responsibly and respects the rights of individuals.
β
Ultimately, sentiment analysis is much more than a simple data analysis tool. It's a window into the minds of consumers, offering companies the opportunity to improve their customer service in a world where emotions play a central role in purchasing decisions and relationship building. By integrating sentiment analysis into their marketing strategy, companies can position themselves at the forefront of innovation and market success, while building customer trust and loyalty.