Video Segmentation: how does artificial intelligence see and understand moving images?
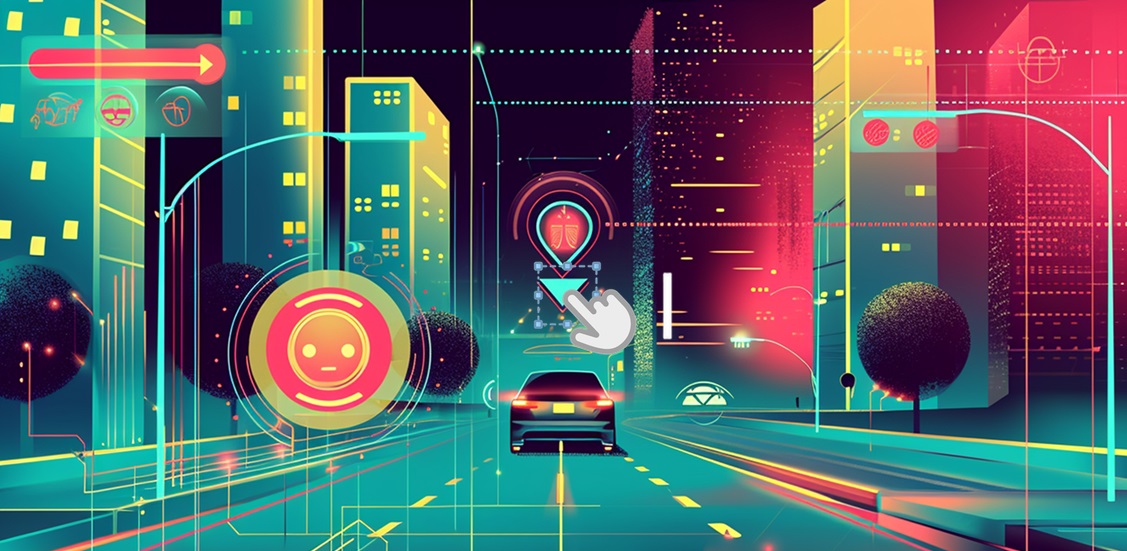
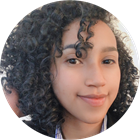
In artificial intelligence, video segmentation is an advanced technology that plays a very important role in the analysis and understanding of video sequences. Several academic articles focus on the difficulties of detecting progressive transitions in the context of video shot segmentation. Using artificial intelligence techniques, this method can divide a video into meaningful segments, facilitating the extraction and interpretation of specific information by artificial intelligence models.
β
πͺ This ability to isolate different categories of objects, people or actions within a video stream is essential in a variety of fields, from surveillance and security to augmented reality and behavioral analysis. By breaking down moving images into discrete elements, AI offers a deeper understanding of visual content, transforming the way we interact with and exploit digital video.
β
β
How does video segmentation differ from conventional image segmentation?
β
Video segmentation and conventional image segmentation are related processes, but they have important differences due to the specificities of the data they process. Benchmarks such as YouTube-VIS are often used to validate video segmentation research.
β
Here are the main distinctions:
β
Temporality vs. staticity
Video segmentation differs from π image segmentation due to the temporal dimension in videos. While image segmentation focuses on a still image at a given point in time, video segmentation deals with a sequence of images, which involves managing variations over time.
β
This temporal component requires techniques that not only segment the objects in each frame, but also track their evolution through the various images in the sequence.
β
Data volume
Video segmentation processes a much larger volume of data than image segmentation. Each video is made up of thousands of frames, each requiring individual analysis for segmentation. This multiplies the requirements in terms of storage and computing power, as each frame has to be processed taking into account its temporal context.
β
In contrast, conventional image segmentation focuses on a single image at a time, which means significantly lower storage and computing requirements. Managing this higher volume of data in video segmentation requires more robust IT infrastructures and optimized algorithms to process large image sequences efficiently.
β
Data complexity
Data complexity is higher in video segmentation than in image segmentation. In the field of Computer Vision, video segmentation techniques can process complex sequences and detect moving objects or changes in lighting with greater precision.
β
In contrast, conventional image segmentation processes a single static image, simplifying the problem by eliminating temporal and dynamic factors.
β
Techniques and algorithms
The techniques and algorithms used for video segmentation are more sophisticated due to the need to process temporal information. 3D convolution neural networks (3D-CNN) and recurrent neural networks (RNN) are commonly used to integrate data across frames.
β
In comparison, conventional image segmentation mainly uses π convolutional neural networks (CNNs), which focus solely on spatial relationships within a single image.
β
Object tracking
Object tracking is an essential step in video segmentation, but not necessary in image segmentation. In video, it's extremely important to maintain the consistency of objects across frames, which requires tracking algorithms capable of handling movement and changes in appearance.
β
In image segmentation, each image is analyzed independently, without the need to follow objects from one image to the next.
β
Management of occlusions and new appearances
Managing occlusions and objects that appear or disappear is a challenge specific to video segmentation. Objects may be partially or totally masked in some frames and reappear later, requiring techniques to maintain their identification over time.
β
In image segmentation, these problems are dealt with in the context of a single image, simplifying the analysis by focusing only on the elements present at a given time.
β
β
β
β
β
β
β
What are the main uses of video segmentation?
β
Video segmentation has a wide range of applications. Here are a few notable use cases:
β
Surveillance and security
Video segmentation is widely used in surveillance systems to detect and track suspicious people or objects in urban environments, airports or shopping malls. It can identify abnormal behavior, recognize faces, and detect unattended objects.
β
Autonomous driving
In the field of autonomous driving, video segmentation helps to identify and track objects such as vehicles, pedestrians and road signs. This technology enables autonomous vehicles to understand their environment in real time and make safer driving decisions.
β
Media and entertainment
Video segmentation is used for tasks such as trailer creation, scene detection and video editing. It can also be used to generate visual effects and animations by isolating objects or characters in video sequences.
β
Behavioral analysis
In behavioral and psychological studies, video segmentation is used to analyze people's movements and interactions. It helps to understand behavioral patterns, assess emotional reactions and improve gesture-based user interfaces.
β
Medicine and anomaly detection
In the medical field, video segmentation is applied to track and analyze patient movements, for example in physical rehabilitation. It can also be used to monitor vital signs and detect anomalies in medical videos, such as endoscopies.
β
Augmented reality and virtual reality
Video segmentation plays a key role in augmented reality (AR) and virtual reality (VR), enabling digital elements to be superimposed on real images. It helps integrate virtual objects seamlessly into the real environment.
β
Sport and performance analysis
Coaches and sports analysts use video segmentation to break down athletes' actions, analyze game strategies and improve performance. It can track players' movements, detect techniques and identify strengths and weaknesses.
β
Human interaction with machines
In vision-based user interfaces, video segmentation is used to detect user gestures and movements to control electronic devices or hand-control systems.
β
Training and education
Video segmentation is used in e-learning environments and educational platforms to create interactive content, such as simulations, hands-on demonstrations and video tutorials.
β
β
π‘ These use cases illustrate how video segmentation can transform various fields by providing detailed analysis and enabling smarter, safer interactions with visual systems.
β
β
What are the current and future trends in video segmentation?
β
News and future trends in video segmentation for artificial intelligence show a continuous evolution, with an increasing connection between new technologies and emerging needs :
β
- Artificial Intelligence and Deep Learning:
Advanced neural networks, such as transformers and 3D-CNNs, improve segmentation accuracy and efficiency by better capturing temporal and spatial relationships.
β
- Real-time segmentation:
The focus is on fast video processing for applications such as autonomous driving and real-time surveillance, requiring algorithms optimized for high performance.
β
- Advanced object tracking:
New techniques, such as graph-based trackers , improve the tracking of objects through complex sequences, even when they are masked or change appearance.
β
- AR and VR integration:
Video segmentation is integrated into augmented and virtual reality technologies, enabling seamless interaction between virtual and real objects.
β
- Medical applications:
The analysis of movements and medical images is evolving, offering more precise tools for diagnosing and monitoring patients.
β
- Mobile optimization and Edge Computing:
The algorithms are optimized for efficient operation on mobile devices and Edge Computing solutions.
β
β
Conclusion
β
Video segmentation represents a major advance in the analysis of visual sequences, enabling a fine, dynamic understanding of video data. By integrating advanced artificial intelligence and Deep Learning techniques, this technology has considerably improved the precision and efficiency of video processing.
β
Current trends, such as real-time segmentation, innovations in object tracking, and integration with augmented and virtual reality technologies, underline the rapid evolution and growing applications of this technology in various fields.
β
The future of video segmentation looks bright, with ongoing developments in the fields of optimization for mobile devices, medical applications, and energy sustainability. By enabling more accurate, real-time video analysis, video segmentation opens the way to smarter, more interactive solutions for many sectors. There will, of course, be challenges (feel free to π see our article on the most common errors in video annotation), but video segmentation promises some very fine use cases in Computer Vision!
β
Future advances will continue to transform the way we interact with visual media and push back the boundaries of what computer vision systems can achieve.