What about synthetic data in AI development?
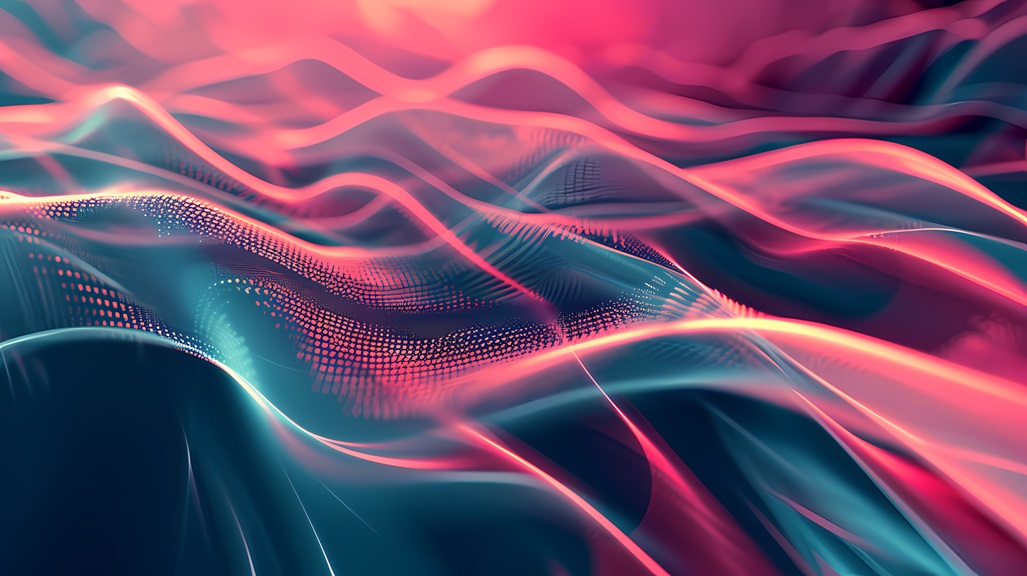
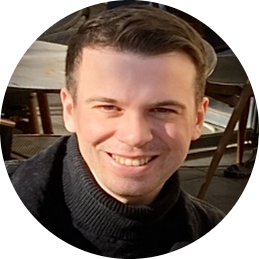
In the field of artificial intelligence (AI), π synthetic data has become a major concept familiar to most data scientists and model specialists. As fuel for AI models, quality data is important. Yet they are often scarce or sensitive. Synthetic data represents a promising solution - it's artificial information generated by computers to mimic real-world data. This advance means that developers can train AI systems more efficiently and ethically without compromising individual privacy, for example.
β
Let's dive in and explore how synthetic data is an important lever for AI development , and why it's a near-indispensable tool for your future AI developments.
β
Why is Innovatiana interested in this subject? This may seem counter-intuitive to you, since Innovatiana is a specialist in manual, human annotation of data. However, one of our objectives is to accelerate the development of AI products, by relying on π quality data. It therefore seems essential to us to insist on this concept, which, combined with π manually generated datacan significantly improve the efficiency and accuracy of AI models. By combining human expertise and advanced techniques such as synthetic data, Innovatiana aims to optimize the AI model training process while ensuring the relevance and authenticity of the data processed.
β
β
π€― BREAKING NEWS(17.09.2024) - Argilla has just published "π DataCraft", an interface using Distilabel to create synthetic datasets! You can test the tool at this address (π https://huggingface.co/spaces/argilla/distilabel-datacraft) and if you'd like to review, enrich or complete your dataset with manual reviews, don't hesitate to contact π Innovatiana ! If you'd like to find out more about Argilla, please π consult our article.
β
β
How do you define synthetic data?
β
Synthetic data is like a clone of original data. Think of it as a copy that isn't real, but looks and acts almost like a real entity. This type of artificial data is manufactured using a computer program that understands how the original data used in the real world appears and functions.
β
This computer program creates new data that has the same patterns and behaviors as the original copied object. It's a bit like the way video games create worlds that look real but are actually made and generated by a computer.
β
What's special about creating synthetic data is that it can be used to test and train AI without touching sensitive or private data belonging to "real" people. This preserves sensitive information. For example, in the healthcare field, AI can learn from synthetic data similar to real patient data, but without any risk of revealing personal information about an individual's health.
β
Synthetic data is used in computer vision and computer simulation! This kind of data can be manufactured in large quantities, and AI needs a very large volume of data (synthetic or real) to learn properly as part of the training process. Using synthetic data allows AI to become "smarter". And with better AI... we can get useful information more efficiently, like predicting the weather better, making smarter robots, or helping doctors determine the best treatments for their patients.
β
Why are synthetic data important?
β
Synthetic data is very important because it helps us solve big AI problems. Remember that AI needs to learn from large data sets. Without sufficient data, AI cannot improve. Sometimes we can't use real data because it's private, like people's medical records or personal information.
β
This is where synthetic data comes in. These are fictitious data that the AI can use to learn. With synthetic data, we don't have to worry about the safety of real data, because the AI doesn't use any in the training process.
β
This means we can create huge amounts of synthetic data and allow AI to learn from it without endangering anyone's privacy. With synthetic data, AI can train again and again, since another AI will be able to generate training data on demand, or almost. In short, synthetic data is a powerful tool for AI.
β
β
β
β
β
What uses are there for synthetic data?
β
Synthetic data is used to generate data for many things, particularly in AI. They're also used as training data to produce original data on demand! Here's how:
β
Training AI models
We use synthetic data as training data to teach the AI. It's like giving the AI a textbook full of examples so it can learn to do things for itself.
β
Testing AI systems
Before AI is ready to really work, it needs to be trained. Synthetic data is ideal for testing, as it doesn't risk using real, sensitive data.
β
Accelerating research
Scientists and engineers can use synthetic data to create AI faster, because they don't have to wait for real data.
β
Privacy policy
This means that AI doesn't need to use private details such as names or health information to generate synthetic data. The dummy data produced preserves people's privacy, since it is generated randomly.
β
Data availability
Sometimes, for many use cases, we just don't have enough real data. Synthetic data fills this gap, providing AI with larger, more accessible data.
β
Cost reduction
Collecting and managing real data can be costly. Synthetic data reduces data collection and retrieval costs, making the AI development cycle less time-consuming and less expensive!
β
By using synthetic data, we ensure that our AIs learn from lots of good examples, without jeopardizing the private information of real people or spending a fortune. It's a smart way of teaching AI to do useful things while using known, responsibly produced data.
β
β
How can synthetic data help AI development?
β
Synthetically generated data aims to generate data to train AI models and generate data based on real scenarios (even if this data itself cannot be described as "real"). Synthetically generated data are important in building advanced AI models. They are also useful for labeling data and providing operational data to make the AI model more intelligent.
β
Let's take a look at how relevant data or synthetic datasets help in the development of AI!
β
Making AI smarter without risk
Synthetic data makes AI smarter, in much the same way as regular running training makes you better able to take part in an Iron Man, or regular revision sessions make you better at exams. AI uses synthetic data to learn how to do things before it does them in the real world. It's a bit like a pilot learning to fly an Airbus A320 on a flight simulator, before actually flying a real airplane.
β
Safe, solid learning
Since synthetic data isn't real, using it means that real private information remains safe. Imagine teaching AI about health without using real patient information - that's what synthetic data allows, in some cases. No real names, no real faces, just machine-learning models without any danger of revealing secrets or compromising an individual's safety.
β
Inexpensive, easy-to-obtain global data
Real data can be hard to find, but AI needs lots of it to learn well. Synthetic data can be created at any time, in any quantity, as long as you have the right tools.
β
Save time and money
Getting real data takes time and money. You need to be careful not to break any laws, depending on the nature of the data you're using or the jurisdiction in which you operate. Producing synthetic data is faster and cheaper. Data is the "raw material" of AI: with synthetic data, you have access to raw material of reasonable quality at low cost, enabling you to start building your AI model very quickly.
β
By using synthetic data in AI, we teach models safely and effectively. We give AI plenty of examples to learn from, and because it's low-cost and risk-free, we can use synthetic data to make AI competent at many jobs, at low cost. This benefits everyone, making life easier and safer.
β
β
How to generate synthetic data for machine learning models?
β
Artificially generated data or synthetic data can be generated through comprehensive planning and meaningful data refinement practices. Data Scientists use synthetic data to produce original data for better machine learning models. Here's an overview of the process applied to turn unstructured data into comprehensive synthetic data, usable for training models!
β
Start with a plan
Before creating synthetic test data, decide what you want your AI to learn. Think about the real data and try to copy its important parts. This means that your fake synthetic test data should have the same types of information as the real thing.
β
Choose your tools
Use special computer programs to create synthetic images or data with the help of natural language processing.
Some programs are called 'generative models' and are very good at producing synthetic data that completely outperforms real data. A popular choice is 'GAN' or Generative Antagonistic Network.
β
Create data
Now start creating data with your tool. The program will look at the actual data points used and try to create new data points used that are similar. We create mathematical models, then train them to produce original data for machine learning!
β
Test and improve
After creating the synthetic data, test it to see if the AI can learn from it. If the AI doesn't do well, change the generation of artificially generated synthetic data a little.
Keep testing and improving until the AI can learn from artificially generated synthetic data as if it were real. To validate mathematical models, it's important to do thorough testing!
β
Use lots of data
Remember, AI needs a lot of synthetic training data to learn well.
Make sure you create plenty of synthetic training data for the AI to practice on. It's like giving someone lots of books to read, and reading goals (for example: read 10 books in 1 month) so they can learn and progress.
β
Control your synthetic data... for greater security
Make sure that the synthetic data generated does not contain any real private information. This avoids potential security problems.
β
By following these steps, you can produce a veritable vault of synthetic data. You can create excellent synthetic data that helps AI models learn safely and quickly. This saves time and money, as well as being an approach that protects people's privacy, and ensures that data is produced ethically.
β
β
Synthetic data vs. real-world data: what's the difference?
β
Synthetic datasets and real-world data are like two flavors of the same ice cream. Both are tasty, both can be combined, but they're not the same. Let's look at how they differ:
β
Synthetic data sets
It's like a robot creating never-before-seen drawings of cats. It's a synthetic data vault designed to be similar to real data. Yet this data is not from the real world. This means that there are no real people or situations, and that a face used, even if it resembles a known person, has been produced entirely by a computer.
β
Real data sets :
This data is extracted directly from everyday life, encompassing names and images of real people. For example, the image of a photographer who captures the essence of urban life through shots of cats in neighborhoods. Data science experts describe this process as an attempt to immerse artificial intelligence in the complexity and diversity of the real world. This approach carries risks, as it sometimes involves the use of data relating to real individuals, thus requiring particular attention to protecting confidentiality and privacy.
β
Acquiring this data can be costly, as it requires a meticulous verification and validation process to ensure its legitimacy and ethical compliance. What's more, the amount of data available is limited by the collection capacities and authorizations required for its use. This poses unique challenges for researchers and developers seeking to integrate this data into artificial intelligence applications, while complying with ethical and legal standards.
β
β
β
β
Why do Data Scientists and Data Managers need synthetic data generation tools?
β
Dat Scientists and Data Managers need tools to create synthetic data, as this is essential to train AI safely and without confidentiality issues. These tools help them to produce large quantities of synthetic data quickly and cost-effectively. They don't have to worry about breaching confidentiality rules because synthetic data doesn't come from real people. What's more, real data may be limited or difficult to obtain, but with synthetic data, as much can be created as required. This means that AI can learn and become very efficient at its tasks, for many use cases, without using real data.
β
Another reason why these tools are valuable is that they create synthetic datasets to help avoid bias in AI training. Real-world data can sometimes be unfair or not include everyone equally. By creating a synthetic dataset, we can create a balanced set of examples for AI to learn from. It's like making sure a teacher has books on all sorts of subjects for his students.
β
Synthetic data generation tools use techniques such as GANs (Generative Adversarial Networks), which are very effective at creating synthetic data anonymously, i.e. something that looks real but isn't. This is perfect for generating synthetic data and test data, enabling AI to be tested and improved, making it ready for the real world without any risk. This is perfect for generating synthetic and test data, enabling AI to be tested and improved, making it ready for the real world without any risk.
β
For example, in healthcare, synthetic data can simulate patient information to train AI without using real patient details. This keeps patient information safe while allowing AI to learn how to help doctors before being used in real-life situations. Similarly, in finance, AI can learn about fraud detection systems without the need for real transactions that might be regulated, or sensitive data.
β
In short, these tools give data scientists the power to harness sensitive customer data to form smarter, more ethical AI systems. This is important because AI is everywhere, helping us in our daily lives, and it needs to be as efficient and fair as possible!
β
β
Final thoughts
β
Ultimately, synthetic data is extremely useful for the AI training process. They are safe, cost-effective and respectful of everyone's privacy. What's more, they're excellent for making AI fair for everyone. We'd love to hear about your own experiences with synthetic data! Have you used them? How have they worked for your AI projects? Share your stories and continue to explore more of this interesting technology. π Let's keep learning and growing together!