Use case: annotating satellite images, or how to decipher the earth from the sky

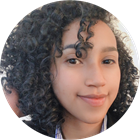
Artificial intelligence, and in particular object detection using Computer Vision techniques, has become an essential component in many fields, from environmental monitoring to natural resource management and national security.
Thanks to artificial intelligence, the ability to analyze and interpret images has undergone a major advance, making it possible to extract important information from the vast volumes of visual data contained in satellite imagery. This, however, is made possible by satellite image annotation methods and, in particular, increasingly complex and powerful data annotation workflows .
The 🔗 neural networks and Deep Learning, now make it possible to decipher details invisible to the human eye. Yet for these technologies to work, they need to rely on complete and accurate datasets. Data annotation, and satellite image annotation in particular, therefore requires a rigorous approach to guarantee the reliability of AI models, while addressing the technical obstacles specific to satellite images, such as variable resolution, atmospheric conditions and geographical diversity.
💡 In this article, we explain what satellite image annotation for artificial intelligence is all about, the tools available for this task, use cases in artificial intelligence, and the challenges and limitations of this technology. Follow the guide!
What is satellite image annotation?
Satellite image annotation is the process of marking and classifying features present in images captured from space, to enable artificial intelligence models to interpret them correctly.
This involves identifying and labeling specific objects, such as buildings, roads, forests or water bodies, in satellite images, in order to train Computer Vision algorithms.
These 🔗 annotationsoften made manually or with AI assistance, are used to create sets of 🔗 training data necessary for models to learn to recognize these elements automatically and accurately.
The aim is to make the analysis of satellite images faster and more accurate, which is useful in various fields such as agriculture, environmental monitoring, urban planning and security.

Why is annotating satellite images important for AIs?
Satellite image annotation is important for artificial intelligence because it provides sets of 🔗 labeled data needed to train machine learning models.
These precise, contextualized annotations are essential for Computer Vision's algorithms to learn how to automatically detect, classify and analyze objects and features visible in satellite images.
Below, we've compiled a few key reasons why it's so important for AI:
Improved precision
Annotations enable AI models to recognize objects (buildings, roads, vegetation, etc.) in satellite images with great accuracy, relying on annotated examples to learn how to distinguish them. The added information helps to 🔗 classify content in order to deliver accurate results to end users.
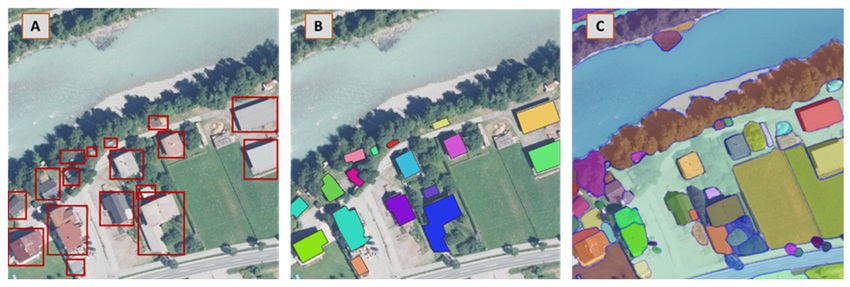
Automating complex tasks
Annotation makes it possible to automate massive image analysis tasks that would be time-consuming and costly if carried out manually, such as environmental change monitoring or resource management.
Continuous model improvement
By regularly updating annotations, AI models can constantly improve and adapt to new data, geographical developments or changing environmental conditions.
Versatile applications
AI trained with satellite image annotations can be used in many sectors, from precision agriculture to natural disaster management, urban planning and defense.
Industries benefiting from satellite image annotation and datasets
Satellite data annotation benefits a variety of industries, including:
Agriculture
In agriculture, data annotation plays an important role in optimizing crop detection, weather forecasting and water resource management systems. Thanks to annotated satellite images, farmers can obtain precise information on the condition of their crops, irrigation requirements and areas affected by pests. This data enables them to make informed decisions, improving yields and the sustainability of farming practices.
Geospatial
The geospatial sector also benefits greatly from data annotation. Annotated satellite images improve the accuracy of navigation, mapping and environmental monitoring systems. Geographers and urban planners can identify areas at risk, monitor environmental changes and plan infrastructure more effectively. Annotated geospatial data is essential for projects such as natural resource management and urban planning.
Predictive maintenance
Finally, in the field of predictive maintenance, data annotation is used to improve fault detection and forecast maintenance requirements. Annotated satellite images can be used to identify early warning signs of faults in infrastructures such as bridges or pipelines. By anticipating potential problems, technicians can plan preventive maintenance interventions, thereby reducing downtime and associated costs.
Annotation techniques
Data annotation techniques are varied and depend on the type of data and the objectives of the annotation. For example, image classification is an annotation technique that involves assigning a label or category to an image based on its content. For example, a satellite image can be classified as representing an urban area, a forest or a body of water. It is also possible to delimit or crop images if you are looking to detect a particular "object" - such as a boat, in the example below:
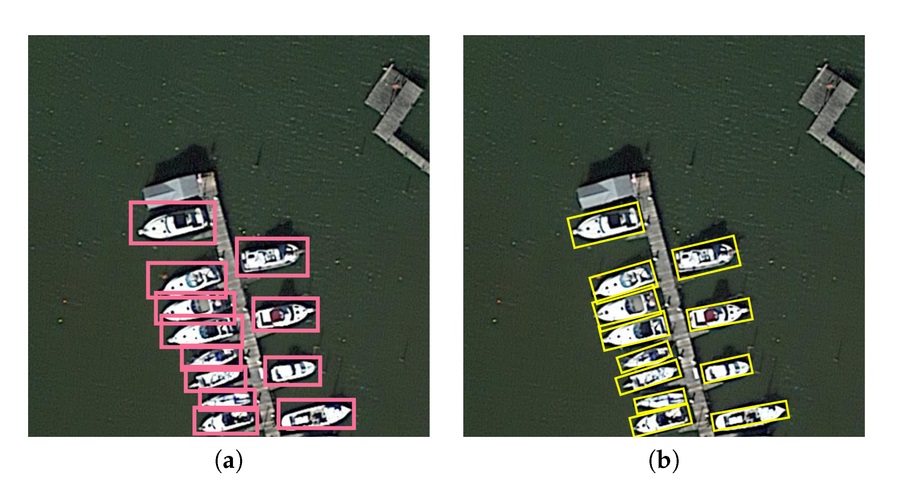
Data annotation techniques are essential for improving the accuracy of artificial intelligence and machine learning systems. Data annotation techniques are varied and depend on the type of data and the objectives of the annotation.
What are the main technical challenges involved in annotating satellite images?
Annotating satellite images presents several major technical challenges, due to the complexity of the data and the specificities of images captured from space. Here are the main obstacles facing specialists when annotating these images:
Image resolution and variability
Satellite images vary in resolution, from very high definition to blurred or granular images. This difference in quality makes object identification more difficult, especially for small details which may be blurred or difficult to distinguish.
Variations in atmospheric conditions
Satellite images are often affected by external factors such as clouds, haze or changing lighting conditions. These atmospheric variations can mask or alter the visibility of objects on the ground, making annotation more complex and less accurate.
Heterogeneous geographical environments
Landscapes captured by satellites can be extremely diverse, ranging from dense urban areas to tropical forests, deserts or snow-covered regions. This heterogeneity requires annotation techniques adapted to each type of environment, increasing the complexity of the process.
Massive data volumes
Satellites capture huge quantities of images covering vast geographical areas. Annotating this data manually is extremely time- and resource-intensive, and even with the help of AI, managing such a volume of data remains a challenge.
Object ambiguity
In some images, objects or infrastructures may be difficult to identify or distinguish, particularly when elements overlap or are partially hidden. For example, it can be difficult to distinguish a road from a river, or a building from a rocky mass.
Temporal changes
Satellite images taken at different times can show changes in the landscape (new construction, deforestation, etc.). Taking account of these temporal variations to maintain up-to-date and consistent annotations represents a major challenge.
Subjective labelling
Image interpretation may be subject to the subjectivity of the 🔗 annotatorsparticularly when it comes to defining precise boundaries or classifications (for example, defining the boundary of a forest or the demarcation of an urban area).
These technical challenges call for innovative solutions, such as improved automatic annotation algorithms, image pre-processing techniques and close collaboration between human experts and AI models to ensure accurate and useful annotations.
What types of data can be extracted from satellite images using annotation?
Thanks to annotation, a wide variety of valuable data can be extracted from satellite images, offering information relevant to many sectors. Here are just a few of the types of data that can be identified and used through this process:
Land use data
The annotation can be used to classify different categories of land use, such as urban, agricultural, forest, aquatic and uncultivated land. This information is essential for land use planning, resource management and urban planning.
Human infrastructure
The annotation of satellite images enables the identification of specific infrastructures, such as roads, buildings, bridges, railroads or pipelines. This data is used for mapping, transport network planning and infrastructure management.
Environmental data
Annotated satellite images can provide information on forests, waterways, coastlines and vegetation cover. This makes it possible to monitor the evolution of ecosystems, track deforestation, manage water resources and monitor the impact of climate change.
Topographic data
By combining satellite images with digital elevation models (DEMs), it is possible to generate data on topography, such as mountain heights, slopes and relief. This information is essential for geological studies, natural risk management and infrastructure construction.
Changes in the environment
The annotation of satellite images makes it possible to detect changes in the environment over time, such as the spread of urbanization, the melting of glaciers, or the degradation of coastal areas. These data are important for monitoring natural disasters and managing environmental risks.
Agricultural data
Annotation can be used to map crop types, plantation health and the extent of cultivated land. This information is invaluable for precision farming, managing crop yields, and monitoring droughts or floods that can affect harvests.
Climatic and meteorological data
By annotating satellite images, we can extract data on climatic phenomena such as storms, cyclones and cloud movements. This information helps forecast weather conditions and monitor large-scale climate change.
Zones at risk
Annotated satellite images can be used to identify areas at risk from natural hazards such as floods, landslides or volcanic eruptions. This helps to prevent disasters and draw up crisis management plans.
Population and urban density
Annotation of satellite images of urban areas enables population density to be estimated by identifying building types and the distribution of dwellings in a given area. These data are useful for urban planning, utility management and demographic studies.
This data, extracted by annotating satellite images, plays a key role in many sectors, including agriculture, urban planning, the environment, security and disaster management. Annotation transforms raw imagery into actionable information for more informed decision-making.
Why is regular annotation updating essential for satellite image analysis?
Updating annotations is essential for satellite image analysis, as it ensures the accuracy and relevance of the artificial intelligence (AI) models used to interpret these images. Here are the main reasons why these updates are important:
Landscape evolution
Natural and built landscapes are constantly changing due to factors such as urbanization, deforestation, natural disasters and human activities such as the construction of new infrastructures.
If annotations are not regularly updated, AI models risk becoming obsolete and offering inaccurate analyses, no longer accurately reflecting reality.
Environmental monitoring
In fields such as natural resource management and environmental monitoring, regular updates of annotations are needed to track changes in the ecosystem, forest cover or the quality of agricultural land.
Without updates, it would be impossible to accurately monitor the impact of climate change or human activities on these ecosystems.
Continuous improvement of AI models
AI models learn from annotated data, and when these annotations are updated, models can adapt and improve their performance. By using updated annotations, AI models become more accurate in 🔗 recognition of objects and geographical features, enhancing their ability to provide relevant and reliable information.
Adapting to new satellite data
Satellite technology is evolving rapidly, with continuous improvements in image resolution and capture capabilities. Annotations need to be adjusted according to new data collected in order to fully exploit these technological advances and ensure that AI models can process the latest data optimally.
Reduced interpretation errors
When annotations are not updated, errors can accumulate in models, particularly in contexts where objects or infrastructures have changed. By regularly updating annotations, it is possible to correct these errors and minimize misunderstandings in image analysis.
Critical applications
In critical sectors such as defense, national security or natural disaster management, obsolete data can have serious consequences. Updating annotations is therefore essential for providing real-time information and reacting quickly to emergency situations.
Conclusion
Satellite image annotation plays a fundamental role in the understanding and analysis of geospatial data. Thanks to the capabilities of artificial intelligence, it is now possible to process massive volumes of visual data with greater precision and speed, while overcoming technical challenges linked to resolution, atmospheric conditions and geographical variations.
However, to maintain the relevance and effectiveness of these analysis models, regular updating of annotations is essential. Sectors that depend on satellite imagery thus benefit from augmented intelligence, capable of decoding our planet's transformations in real time, and providing strategic insights for more informed decision-making.