Discover the secrets of FDA-compliant Data Labeling
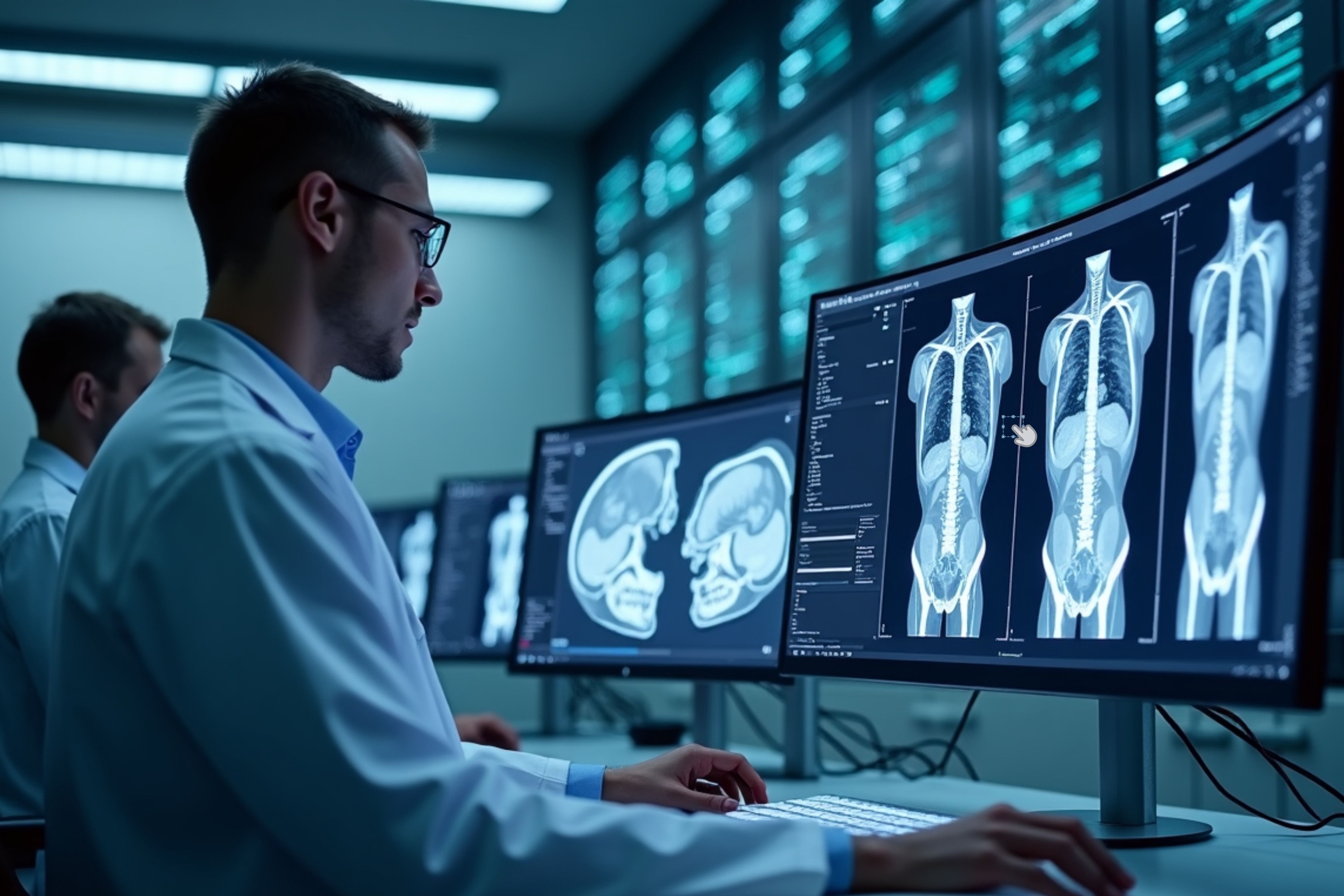
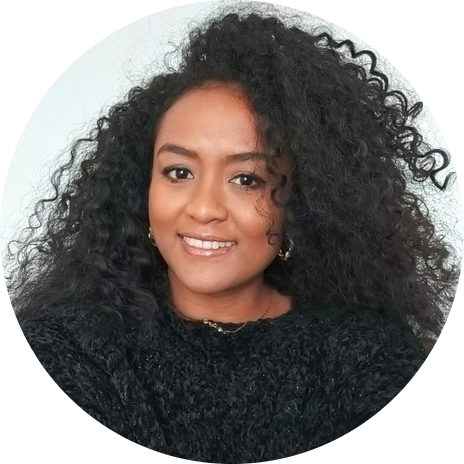
In 2020, an analysis revealed that. π 70% of diagnostic AI systems based on visual data rely on data from just three US states. This lack of diversity poses a real problem in the healthcare technology sector.
β
As medical data labeling requirements evolve, the π Food and Drug Administration (or FDA) has taken concrete steps. In January 2021, it published its π "AI/ML-Based Software as a Medical Device Action Plan", establishing for the first time a federal framework in the US, to regulate AI and Machine Learning in medical devices.
β
To ensure that these technologies remain safe and effective, for patients and healthcare professionals alike, it is essential to understand why data labeling is an important link in the chain, and what standards need to be met.
β
β
π‘ In this guide, we'll look in detail at the FDA's requirements, its approach to using training data for AI, and the key steps to implementing an FDA-compliant labeling process within your organization.
β
β
β
β
β
β
The fundamentals of medical data labelling
β
In the medical field, we face a major challenge: π 80% of healthcare data is unstructuredwhich makes it difficult to exploit. This is precisely where Data Labeling or the implementation of a LabelOps or π DataPrepOps process comes into play.
β
Medical Data Labeling is the meticulous process of annotating images, videos and other medical data with precise, relevant information (also known as "metadonenes"). Indeed, this practice enables AI algorithms to understand and interpret medical images, in particular to identify :
- Anatomical structures
- Specific pathologies
- Potential anomalies
- Important clinical signs
β
To ensure the accuracy of medical diagnoses, data annotation specialists (such as Innovatiana) use various annotation methods, including :
- Bounding boxes to delimit areas of interest
- Polygons to mark precise contours
- Benchmarks for identifying specific structures
β
β
In addition, the quality of labeling is crucial, as it directly impacts the performance of artificial intelligence models, and therefore diagnostics. At Innovatiana, we call on experts in the medical field who can accurately identify symptoms, diseases and treatments. These specialists use specialized software such as V7, Encord, RadiAnt DICOM Viewer or OsiriX to guarantee annotations in line with clinical standards.
β
β
FDA regulatory requirements for Medical Data Labeling
β
To ensure data labeling compliance in medical artificial intelligence, it is essential to follow the FDA's strict guidelines for labeling and data management. The aim is to ensure that datasets used to train AI models comply with safety, traceability and quality requirements applicable to medical devices.
β
π Classification of medical devices and impact on Data Labeling
The FDA classifies medical devices into three categories according to their level of risk, which directly influences the level of Data Labeling requirements:
- Class I(low risk): General controls, suitable for non-critical annotation systems (e.g. diagnostic tools without automated decision-making).
- Class II(moderate risk): Stricter requirements, requiring rigorous validation of the annotations used to train AI models (e.g. automated detection of anomalies on medical images).
- Class III(high risk): Requires prior FDA approval, as these systems can have a direct impact on medical decision-making (e.g. AI interpreting scans to diagnose serious pathologies).
β
β Implementation of a quality assurance program for Data Labeling
The FDA requires industry players to set up a quality assurance program incorporating good practices specific to Data Labeling, including:
- Data annotation and structuring standards : Compliance with DICOM (medical imaging), HL7 FHIR (data interoperability) and GxP (good manufacturing and data management practices).
- Annotation validation: Rigorous process including cross-reviews by medical experts and AI specialists to ensure the accuracy of applied labels.
- Full documentation and traceability: All Data Labeling stages must be recorded in a validation file to prove compliance with regulatory requirements.
β
π Controls and audits to ensure compliance
To meet FDA standards, the Data Labeling process must include strict controls, including :
- Systematic verification of annotation consistency via quality metrics (e.g. π inter-annotator agreementstatistical analyses).
- Recording changes : Any changes to labels or annotation algorithms must be documented and approved before implementation.
- Keeping version histories: Each dataset used to train a model must be stored with clear versioning to trace the origin and evolution of annotations.
β
π FDA validation and acceptance of datasets
Before using an annotated dataset to train medical AI, the FDA requires a formal evaluation guaranteeing that it meets quality and safety criteria. This validation includes:
- Performance tests on annotated samples to ensure model robustness.
- Checks for compliance with FDA standards and guidelines on AI/ML-Based Software as a Medical Device.
- Audit of Data Labeling methods to avoid bias and guarantee data representativeness.
β
β
π‘ By integrating these regulatory requirements right from the start of the Data Labeling process, we contribute to the compliance of medical AI models and facilitate their approval by the FDA, ensuring reliable and safe solutions for patients and healthcare professionals.
β
Setting up a compliant Labeling process
β
To ensure Data Labeling complies with regulatory requirements in the development of medical AI, it is essential to structure a rigorous process integrating both human expertise and advanced technological tools.
β
The first step is to define π precise annotation protocolsaligned with FDA recommendations and industry standards, such as DICOM for medical images and HL7 FHIR for data exchange. We use specialized annotation platforms such as V7 or Encord, which ensure consistency and high quality through cross-validation by medical experts and machine learning specialists.
β
The integration of multi-level annotations is also key:
- Automatic pre-annotation using AI models to speed up processing.
- Human validation by radiologists or clinicians to guarantee accuracy.
- Audit and correction via iterations based on reliability metrics.
β
To process large volumes of data while respecting patient confidentiality, we implement pseudonymization protocols and apply HIPAA or GDPR requirements. In addition, the use of synthetic data is an interesting alternative to overcome the limitations of real medical datasets, particularly in terms of diversity and protection of sensitive data.
β
β
π‘ Looking for datasets to experiment with and develop medical AI models? Don't hesitate to π consult our Top 15 !
β
β
Finally, a continuous monitoring system ensures that annotation models and datasets evolve in line with the latest FDA regulations and industry best practices, guaranteeing reliable and exploitable labeling for training AI models.
β
Conclusion
β
FDA requirements for medical data-labeling may seem complex at first glance. Nevertheless, our analysis shows that a methodical, structured approach can achieve the required compliance.
β
The success of a Compliant Labeling program rests on three essential pillars. Firstly, the expertise of specialized medical annotators guarantees data accuracy. Secondly, a robust validation and traceability system ensures the quality of the process. Finally, rigorous security protocols protect sensitive patient information.
β
The future of medical data-labeling will depend on the ability to maintain these high standards while adapting to technological developments. We are convinced that companies who invest in FDA-compliant processes today are positioning themselves favorably for tomorrow's medical innovations...
β
β