Annotation guide or manual: the basis of a successful Data Labeling project!

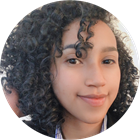
Dans le vaste domaine de la science des données, la précision et la cohérence des annotations (ou métadonnées) venant enrichir un dataset sont des éléments déterminants pour la réussite d’un projet IA. La nature et le contenu des annotations sont importants pour structurer et préparer les données pour les algorithmes d'intelligence artificielle. Pour préparer ces données, un guide ou manuel d’annotation joue un rôle essentiel en fournissant des directives claires et uniformes aux annotateurs, garantissant ainsi une qualité optimale des données annotées.
The annotation guide or manual, often perceived as unhelpful or unstructured, is in fact one of the pillars for guaranteeing the integrity and reliability of datasets. By establishing precise standards and describing annotation procedures, annotation manuals help minimize errors and subjective variations, thus facilitating machine learning and data analysis by AI models.
What is an annotation guide or manual, and why is it important?
An annotation manual is a comprehensive document that provides clear and detailed guidelines on how data should be annotated during the coordination of a data science Data Labeling project. This manual is essential to ensure that annotations are accurate, consistent and in line with each specific project objective or requirement.
It is typically used by Data Science teams, annotators and AI project managers to ensure that all team members follow the same rules and standards when annotating data. Here's what makes it so important:
Consistency of annotations
An annotation guide or manual helps to establish clear, uniform standards for data annotation. Structured data with precise guidelines influences the consistency of annotations by facilitating prior structuring, which can obviate the need for annotation. This ensures that all data is annotated consistently, minimizing subjective variations between different annotators.
Data quality
By providing precise guidelines, an annotation manual helps reduce errors and ambiguities in annotations. This improves data quality, which is critical for the development of high-performance, reliable Machine Learning models.
Operational efficiency
A well-written manual facilitates the work of annotators by providing clear, detailed instructions. This can reduce the time needed to train new annotators and improve the overall efficiency of the annotation process.
Bias reduction
Biases in the data can have a significant impact on the results of Machine Learning models. The creation of annotation units can give rise to discussions between members of the same Data Science team, helping to reduce bias by promoting a common understanding of annotation criteria. A well-designed annotation manual can help identify and mitigate these biases by defining objective criteria for annotation, known to all.
Documentation and traceability
The annotation manual also serves as official documentation for the project, enabling annotation decisions and processes to be traced. This is particularly useful for audits and data quality assessments. To date, the majority of datasets available are not accompanied by a precise description of the rules that enabled their collection and construction: this is a mistake, and we feel it is important to point out that every dataset used in AI should be accompanied by a precise guide describing the means used to make the dataset reliable. For example, an annotation manual could explain why Bounding Boxes have been used instead of Polygons, or why certain labels have been deliberately ignored.
Facilitating collaboration
When several teams of annotators are working on the same project, an annotation manual helps maintain a consistent, collaborative approach. This fosters better communication and a shared understanding of project objectives. It also enables data to be reworked at a later date, to optimize quality, for example.
What are the essential elements of a good annotation manual?
Here are the key elements that an annotation manual should include:
Introduction and background
A good annotation manual begins with a clear introduction that explains the overall purpose of the project. This section should provide an overview of why the annotations are needed and how they will be used. For example, if the project concerns the annotation of text for a natural language processingthe introduction should explain how these annotations will help improve the accuracy of the model. In addition, it is important to define the manual's target audience, whether annotators, supervisors or any other stakeholder. This clarification ensures that all parties understand the context and expectations of the project.
Terminology and definitions
A section dedicated to terminology and definitions is essential to ensure that all annotators understand terms and concepts in a consistent way. A well-structured glossary with clear definitions of all relevant terms is essential. For example, in a sentiment annotation project, terms such as "positive", "negative" and "neutral" need to be clearly defined. Including concrete examples for each term can greatly help eliminate ambiguities and ensure consistent annotations.
Annotation guidelines
The annotation guidelines are the heart of the manual. They should begin with general rules, such as the importance of precision, consistency and objectivity in annotations. Then, each annotation category must be clearly defined. For example, if you're working on annotating speech types, each type must have a precise definition. Inclusion and exclusion criteria are also necessary to ensure that annotators know exactly what should and shouldn't be annotated in each category. To deal with special cases or ambiguous situations, specific instructions must be provided. These detailed guidelines help minimize variations between annotators and maximize the quality of annotated data.
Annotation tools and interface
It's important to detail the annotation tools and interface that annotators will be using. Annotation software and online tools are essential to facilitate collaboration and interaction on the Web. A clear description of the tool's functionality, accompanied by screenshots, can help annotators quickly familiarize themselves with the interface. For example, if you use a specific annotation software, explain how to create, modify and save annotations. Including step-by-step instructions for common tasks can also reduce the time needed to train annotators and increase their efficiency.
Annotated examples
Annotated examples are extremely useful for illustrating annotation guidelines in action. They allow annotators to see how rules and criteria are applied in real-life situations. Including annotated examples for each category and type of situation, including ambiguous or difficult cases, can greatly enhance annotators' understanding. For example, for a sentiment annotation project, show examples of text annotated as positive, negative and neutral, with explanations of the decisions made.
Quality management
Ensuring the quality of annotations is crucial to the success of the project. The manual should include quality control procedures, such as regular reviews of annotations by supervisors or double annotation where two independent annotators annotate the same data. Define quality metrics, such as accuracy and inter-annotator inter-annotator consistencyenable annotation quality to be measured and continuously improved. Instructions on how to resolve disagreements between annotators should also be included to ensure that the final annotations are as accurate and reliable as possible.
Revisions and updates
Finally, a good annotation manual should be a living document, subject to regular revision and updating. To modify annotations, it's important to know how to switch to edit mode, which allows you to modify, delete or link annotations, as well as change the type of annotation. Defining a clear process for collecting feedback from annotators and incorporating this feedback into updates to the manual is essential to ensure that the document remains relevant and useful. Announcing major revisions and training annotators on the changes can also help maintain a high level of quality and consistency in annotations throughout the project.
💡 En intégrant ces éléments, un manuel d’annotation devient un guide complet et efficace pour les annotateurs, assurant la qualité et la cohérence des données annotées et contribuant au succès global du projet.
How does an annotation manual improve the quality of a data corpus?
An annotation manual improves the quality of a data corpus in several essential ways:
Standardizing annotations
An annotation manual provides clear, uniform guidelines on how each item in the corpus should be annotated. This reduces subjective variations between different annotators, ensuring that annotations are consistent and conform to defined standards. This standardization is crucial to ensuring data reliability, as it minimizes errors and inconsistencies that might otherwise compromise the integrity of the corpus.
Error reduction
By defining precise rules and concrete examples, an annotation manual helps reduce common mistakes that annotators might make. Detailed instructions and specific use cases enable annotators to understand exactly how to deal with ambiguous situations, which improves the accuracy of annotations and, consequently, the overall quality of the corpus.
Bias management
Biases in the data can have a negative impact on machine learning models and subsequent analyses. A well-designed annotation manual can help identify and mitigate these biases by providing objective criteria and making annotators aware of the types of bias possible. This helps to create a more balanced and representative corpus.
Annotator training and efficiency
An annotation manual also serves as a training tool for new annotators. By providing clear instructions and practical examples, it makes annotation tasks easier to learn and understand. This enables annotators to work more efficiently and produce high-quality annotations right from the start, improving the quality of the data corpus.
Documentation and traceability
The annotation manual acts as a formal documentation record of annotation decisions and processes. It allows you to trace the steps taken and understand the choices made during annotation. This traceability is essential for assessing data quality and making adjustments or corrections if necessary.
Facilitating collaboration
In large-scale projects, several annotators or teams may be involved in data annotation. An annotation manual ensures that all participants follow the same guidelines, facilitating collaboration and communication. This coordinated, consistent approach improves the quality and cohesion of the data corpus.
What strategies should you adopt for a successful annotation campaign?
To run a successful annotation campaignThere are several strategies you can adopt. Here are some of the most important:
1. Define clear objectives
Before starting the annotation campaign, it's important to clearly define the objectives. What data and metadata are required? What types of annotation are expected? These questions need to be addressed to guide the project.
2. Create a detailed annotation manual
A well-developed annotation manual is essential. It must contain precise instructions, concrete examples and use cases to help annotators understand and correctly apply annotation rules. The manual must also be regularly updated in line with feedback from annotators.
3. Training annotators
Proper training of annotators is essential. They need to understand the annotation manual, the campaign objectives, and the tools they will be using. Hands-on training sessions with exercises and concrete examples can greatly improve the quality of annotations.
4. Use appropriate tools
Choosing the right annotation tools for your project is crucial. On-line annotation guides can greatly facilitate this process. These tools must be user-friendly, enable efficient annotation, and offer annotation management and tracking functionalities. Collaborative annotation platforms can also facilitate teamwork.
5. Set up a quality control process
To guarantee the quality of annotations, it is important to implement a quality control process. This can include peer reviews, regular sampling of annotations, and the use of quality metrics to assess annotation accuracy and consistency.
6. Manage returns and adjustments
It's important to regularly gather feedback from annotators and use it to adjust and improve the annotation manual and annotation processes. Annotators should have a channel to ask questions and report problems, and this feedback should be taken into account to improve the campaign.
7. Plan milestones and intermediate objectives
Breaking down the annotation campaign into stages, and setting intermediate objectives, makes it easier to manage the project and ensure that everything goes according to plan. It also enables any problems to be detected and corrected quickly.
8. Encourage communication and collaboration
Fostering good communication and effective collaboration between annotators and project managers is essential. Regular meetings, updates on project progress, and open discussion of challenges encountered can help maintain a positive and productive dynamic. A single communication channel (e.g. Discord) is important to enable teams to collaborate and support each other.
9. Use reference data
Having reference data or "golden setsgolden sets"can be very useful for evaluating annotator performance and calibrating annotations. These data should be annotated in an exemplary manner and serve as a standard for annotators.
10. Evaluate and adjust regularly
Finally, it is useful to regularly evaluate the progress of the annotation campaign and adjust strategies according to the results obtained and feedback received. This ongoing evaluation helps to maintain the high quality of annotations and achieve project objectives.
💡 En adoptant ces stratégies, une campagne d'annotation peut être menée de manière efficace et aboutir à un corpus de données de haute qualité, essentiel pour les projets de science des données et de Machine Learning.
Conclusion
Annotation manuals play an indispensable role in the success of Data Labeling projects, ensuring the quality, consistency and reliability of annotated corpora. By establishing clear guidelines and standardizing annotation processes, these documents help minimize errors, reduce bias, and significantly improve the accuracy of Machine Learning models. They also serve as an essential reference for the training of annotators and the efficient management of annotated data.
Beyond their technical aspects, annotation manuals facilitate team collaboration, ensuring a uniform, coordinated approach throughout the project. They are also invaluable documentation tools, recording decisions taken and ensuring the traceability needed for auditing and ongoing assessment of data quality.
To optimize the effectiveness of annotation campaigns, it is critical to design manuals adapted to the specificities of each project, and to invest in the ongoing training of annotators. By following these best practices and incorporating feedback, teams can not only improve the quality of their annotated data, but also maximize the impact of their data science projects.
Ultimately, annotation manuals represent not only the basis of a successful Data Labeling project, but also an essential pillar of evolution and innovation in the field of artificial intelligence and data analysis.