Inter-Annotator Agreement or how to control the reliability of data evaluated for AI?
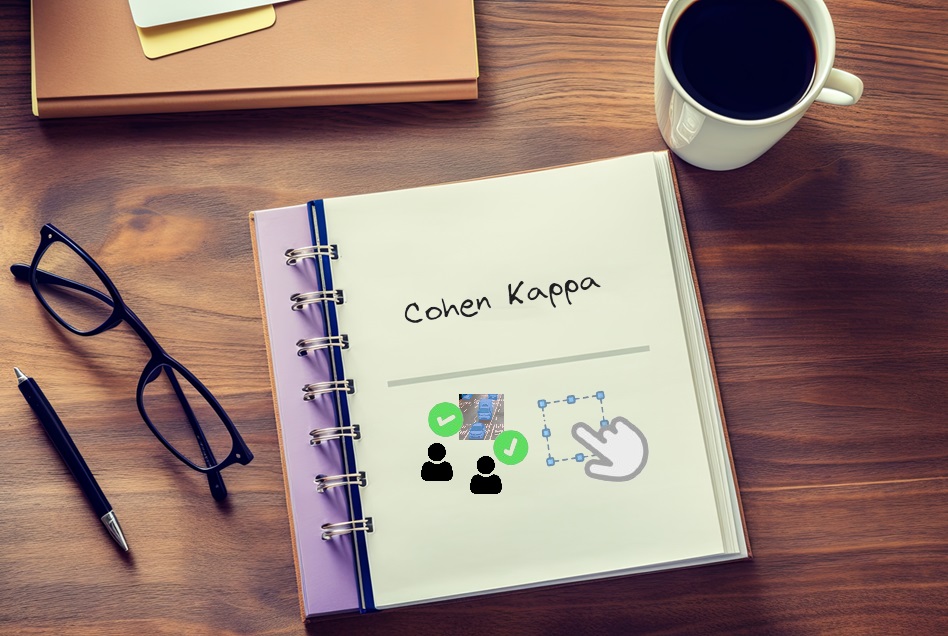
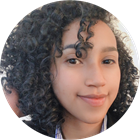
β
β
What is the Inter Annotator Agreement (IAA) and why is it important?
β
An Inter-Annotator Agreement (IAA) is a measure of the agreement or consistency between each annotation produced by different annotators working on the same task or dataset, as part of the preparation of a training dataset for AI. Inter-Annotator Agreement assesses the extent to which annotators agree on the annotations assigned to a dataset (or π dataset).
β
The importance of Inter-Annotator Agreement lies in its ability to provide a scientific and accurate indication of evaluations. In the fields previously mentioned, especially the development of AI products relying on big data, decisions and conclusions are often based on every annotation provided by π human annotators. Without a means of measuring and guaranteeing the consistency of these annotations, the results obtained can be biased or unreliable!
β
β
IAA makes it possible to quantify and control the consistency of each annotation. This helps to improve the quality of annotated data and the robustness of the resulting analyses, and of course of the results produced by your AI models. By identifying discrepancies between annotators, Inter-Annotator Agreement also helps to target points of disagreement and clarify annotation criteria. This can improve the consistency of any annotations subsequently produced, during the data preparation cycle for AI.
β
β
How does the Inter Annotator Agreement help ensure the reliability of annotations in AI?
β
Inter-Annotator Agreement is a metric that contributes to the reliability of evaluations in several ways:
β
Measuring annotation consistency
The IAA provides a quantitative measure of the agreement between each annotation assigned by different annotators. By evaluating this concordance, we can determine the reliability of evaluations and identify areas where there are discrepancies between annotators.
β
Identifying errors and ambiguities
By comparing each annotation, i.e. the metadata produced by different annotators on a specific dataset, Inter-Annotator Agreement helps identify potential errors. And by the same token, ambiguities in annotation instructions (or annotation manuals) and gaps in annotator training. Correcting these errors improves the quality of the metadata, the datasets produced and, ultimately, the AI!
β
Clarification of annotation criteria
Inter-Annotator Agreement can help clarify annotation criteria by identifying points of disagreement between annotators. By examining these points of disagreement, it is possible to clarify annotation instructions and then provide additional training for annotators. This is a good practice for improving the consistency of assessments!
β
Optimizing the annotation process
By regularly monitoring the Inter Annotator Agreement, it is possible to identify recurring trends and problems in evaluations, in datasets under construction. This helps optimize the annotation process, whether for images or π videos in particular, by implementing corrective measures along the way to improve the reliability of dataset evaluations over the long term.
β
β
β
β
β
β
β
β
β
What are the common methods used to assess the reliability of an annotation?
β
Several methods are commonly used to assess the reliability of each annotation. Here are some of the most widely used methods:
β
β
Cohen's Kappa coefficient
Cohen's Kappa coefficient is a statistical measure of agreement between two annotators, corrected for the possibility of random agreement. It is calculated by comparing the observed frequency of agreement between annotators with the expected frequency of agreement by chance. This coefficient ranges from -1 to 1, where 1 indicates perfect agreement, 0 indicates agreement equivalent to that obtained by chance, and -1 indicates perfect disagreement. This measure is widely used to assess the reliability of binary or categorical annotations, such as presence/absence annotations, or annotations for classification into predefined categories (e.g. dog, cat, turtle, etc.).
β
β
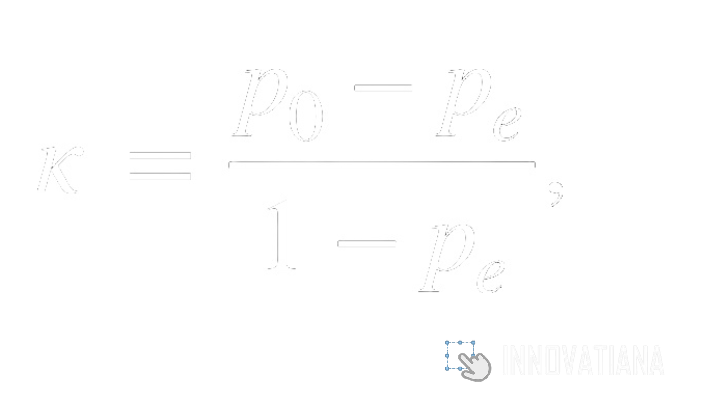
β
β
Krippendorff alpha coefficient
Krippendorff's alpha coefficient is a measure of inter-annotator reliability that evaluates the agreement between several annotators for categorical, ordinal or nominal data. Unlike Cohen's Kappa coefficient, it can be applied to data sets with more than two annotators. Krippendorff's alpha coefficient takes into account sample size, category diversity and the possibility of agreement by chance. It ranges from 0 to 1, where 1 indicates perfect agreement and 0 indicates complete disagreement. This measure is particularly useful for assessing the reliability of annotations in situations where several annotators are involved, as in inter-annotator studies.
β
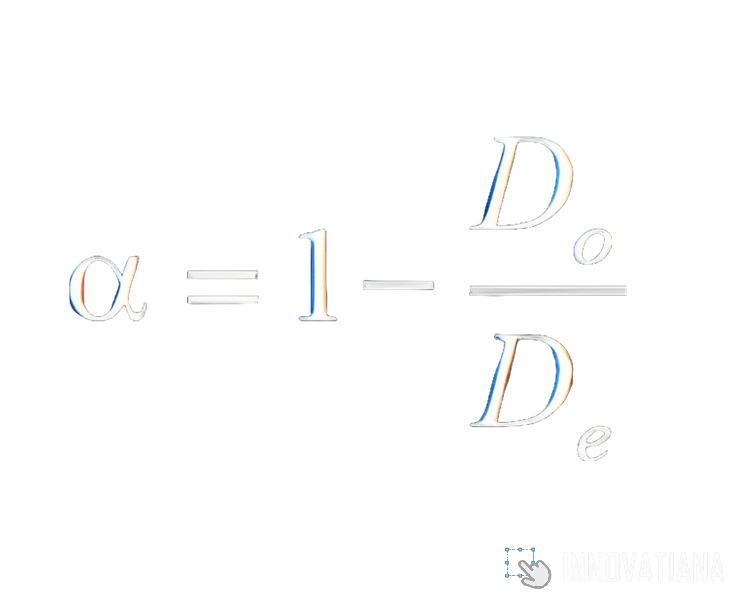
β
β
Intra-class correlation coefficient (ICC)
The intra-class correlation coefficient is a reliability measure used to assess the agreement between the continuous or ordinal annotations of several annotators. It is calculated by comparing the variance between annotators' annotations with the total variance. This gives an estimate of the proportion of variance attributable to agreement between annotators. CCI ranges from 0 to 1, where 1 indicates perfect agreement and 0 indicates complete disagreement. This measure is particularly useful for assessing the reliability of quantitative or ordinal measures, such as performance evaluations or quality assessments.
β
β
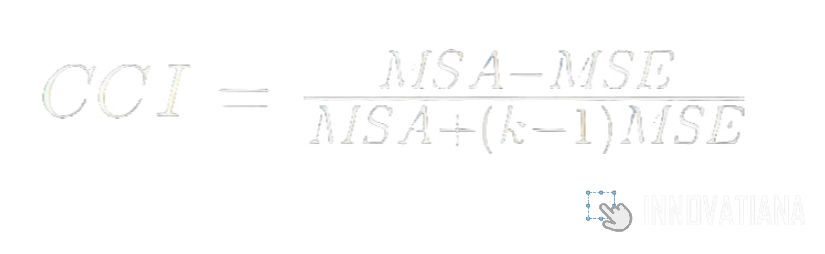
β
β
Discordance analysis
Discordance analysis involves examining cases where annotators differ in their annotations, to identify potential sources of disagreement. This may include examining cases where annotators interpreted instructions differently, cases where instructions were ambiguous, or cases where annotators lacked training on the annotation task. This analysis enables us to understand the reasons for discrepancies between annotators, and to identify ways of improving annotation consistency in the future.
β
β
Internal reliability analysis
Internal reliability analysis evaluates the internal consistency of annotations by examining the concordance between different annotations from the same annotator. This can include measures such as intra-annotator consistency, which assesses the stability of an annotator's annotations over several evaluations of the same task. This analysis determines whether an annotator's annotations are consistent and reliable over time.
β
β
Margin of error analysis
Margin of error analysis evaluates the variability of annotations by examining the discrepancies between the same annotator's annotations on similar elements. This can include examining cases where an annotator has assigned different annotations to elements that should be similar according to annotation guidelines. This analysis quantifies the accuracy of annotations and identifies the most error-prone elements. This can provide valuable pointers for improving annotation instructions or training annotators.
β
β
How to use Inter-Annotator Agreement effectively in annotation processes for AI?
β
To set up an efficient AI annotation process, Inter-Annotator Agreement can be used as a quality control metric. To implement this metric, several key steps need to be followed. First of all, it's important to clearly define annotation guidelines, specifying the criteria to be followed when annotating data. These instructions must be precise, complete and easy for the annotators (or Data Labelers) to understand. For greater efficiency, it's best to provide them with in-depth training on annotation and the task in hand. It's vital that Data Labelers fully understand the instructions and are able to apply them consistently!
β
Before launching the annotation process on a large scale, it is advisable to carry out a pilot, i.e. a test with a small data set and several annotators. This helps to identify and correct any problems in the annotation instructions or in the annotators' understanding. Continuous monitoring of the annotation process is also necessary to detect any problems or inconsistencies. This can be achieved by periodically examining a random sample of annotations produced by annotators.
β
If problems or inconsistencies are identified, annotation instructions should be revised and clarified based on feedback from annotators. The use of appropriate annotation tools can also facilitate the process and ensure consistent annotations. These tools can include online platforms specialized in data annotation or customized software developed in-house.
β
Once the annotations are complete, it is necessary to assess inter-annotator reliability using methods such as Cohen's Kappa or Krippendorff's alpha. This will quantify agreement between annotators and identify possible sources of disagreement. Finally, the results of the inter-annotator reliability assessment should be analyzed to identify potential errors and inconsistencies in the annotations. These should then be corrected by revising the annotations concerned and clarifying annotation instructions where necessary.
β
π‘ Want to find out more and learn how to build quality datasets? π Discover our article !
β
β
How is Inter-Annotator Agreement used in Artificial Intelligence?
β
In the field of Artificial Intelligence (AI), Inter-Annotator Agreement plays a key role in guaranteeing the quality and reliability of the annotated datasets used to train and evaluate AI models.
β
Training AI models
AI models require annotated datasets for training and effective machine learning. This is the case for deep neural networks, machine learning algorithms and natural language processing systems. Inter-Annotator Agreement is used to guarantee the reliability and quality of annotations in these datasets. This results in more accurate and reliable models.
β
Model performance evaluation
Once the AI models have been trained, they need to be evaluated on test data sets to measure their performance. Inter-Annotator Agreement is also used in this context to ensure that annotations in test sets are reliable and consistent. This guarantees accurate evaluation of model performance.
β
Correction of modeling errors
When analyzing the results of AI models, it is often necessary to identify and correct modeling errors. Inter-Annotator Agreement can be used to assess the quality of annotations in annotated datasets and identify areas where models produce incorrect results. This helps to understand the shortcomings of models and improve their accuracy.
β
Development of a specific data set
In some cases, it may be necessary to create a specific dataset for particular AI tasks. Inter-Annotator Agreement is then used to guarantee the quality and consistency of annotations in this dataset. This makes it possible to develop AI models tailored to specific domains or applications.
β
β
β
β
β
β
β
β
What are the advantages and disadvantages of using LPN?
β
Using the Inter-Annotator Agreement has both advantages and disadvantages in a number of areas.
β
Benefits
By proactively using Inter-Annotator Agreement, AI specialists or Data Scientists can ensure the quality and consistency of evaluations in various domains, strengthening the validity of analyses and, potentially, model performance. Here are just a few of the benefits:
β
1. Valuation reliability
Inter-Annotator Agreement enables the concordance between the annotations of different annotators to be measured, thus boosting confidence in the assessments made. For example, in academic research, where studies are often based on the analysis of manual annotations, IAA ensures that results are based on reliable and consistent data. Similarly, in the development of AI systems, reliably annotated datasets are essential for training accurate models.
β
2. Error identification
By comparing the annotations of several annotators, Inter-Annotator Agreement can identify inconsistencies and errors in annotated data. For example, in data analysis, it can reveal discrepancies in the interpretation of information. This allows errors to be identified and corrected. At the same time, it helps to improve data quality and avoid potential biases in subsequent analyses.
β
3. Clarification of annotation instructions
When annotators produce divergent annotations, this can signal ambiguities in annotation instructions. By identifying points of disagreement, IAA helps to clarify and refine instructions, improving the consistency of annotations in the future. For example, in the field of π image classificationdiscrepancies in the assignment of certain classes may indicate a need to revise guidelines for better interpretation.
β
4. Optimizing the annotation process
By regularly monitoring the IAA, it is possible to identify trends and recurring problems in data evaluations of all types. This enables continuous improvements to be made to the annotation process, by implementing corrective measures to enhance the quality of evaluations over the long term. For example, if the IAA reveals a sudden drop in agreement between annotators, this may indicate a need for revised instructions or additional training for annotators.
β
β
Disadvantages
Although the IAA offers many advantages for guaranteeing the quality and reliability of assessments in different fields, this metric also has its drawbacks.
β
Cost in time and resources
Setting up a labeling process and associated metrics such as IAA can be a time-consuming and resource-intensive task. Qualified annotators need to be recruited and trained, the annotation process supervised, annotated data collected and processed, and metrics analyzed on a regular basis to optimize data and metadata production. This process can be time-consuming and require a significant financial investment, especially in fields where data is numerous or complex.
β
Analysis complexity
The analysis of metrics such as IAA can be complex, especially when several annotators are involved or when the annotated data is difficult to interpret. It is often necessary to use advanced statistical methods to assess the concordance between annotations and interpret the results appropriately. This may require specialized skills in statistics or data analysis, which can be a challenge for some Data Labeling teams.
β
Sensitivity to human bias
Data labeling processes can be influenced by annotators' individual biases, such as personal preferences, subjective interpretations of annotation instructions or human errors. For example, an annotator may be more inclined to assign a certain annotation due to his or her own opinions or experiences, which can bias AI models. It is important to take steps to minimize these biases, such as training annotators and clarifying annotation instructions.
β
Limitations in certain contexts
In certain domains or for certain tasks, the use of a metric like IAA may be limited by the nature of the annotated data. For example, in domains where data is scarce or difficult to obtain, it may be difficult to build a reliable annotated dataset. Similarly, in domains where annotation tasks are complex or subjective, it may be difficult to recruit experienced annotators capable of producing high-quality annotations.
β
Possibility of persistent disagreements
Despite efforts to clarify annotation instructions and harmonize practices, annotators may continue to have differing opinions on certain annotations. This can lead to persistent disagreements between annotators, making it difficult to resolve differences. In some cases, this can compromise the overall quality of evaluations and therefore of datasets!
β
β
With these drawbacks in mind, it's important to put measures in place to mitigate their effects and maximize the benefits of using an indicator like AI in different applications. This can include thorough training of annotators, regular clarification of annotation instructions, close monitoring of the annotation process, and above all careful analysis of AI results to identify and correct potential problems.
β
β
In conclusion
β
In conclusion, Inter-Annotator Agreement (IAA) is an essential tool for guaranteeing the quality and reliability of annotated data used in artificial intelligence. It's a metric that is becoming increasingly popular with the most mature data-labeling teams.
β
By measuring consistency between annotators, IAA ensures that datasets are reliable and free from bias, thus contributing to the effectiveness of the AI models developed. Despite challenges such as cost and complexity, the importance of IAA lies in its usefulness as a metric for continuously improving the annotation process.
β
By using IAA wisely, teams of data scientists and AI specialists can optimize annotation processes, thereby enhancing the quality of the datasets produced. The role of IAA in the development of training data and the evaluation of AI models is therefore undeniable, making this indicator a real pillar in the preparation of high-quality data for future technologies.