Top 15 medical datasets essential for AI
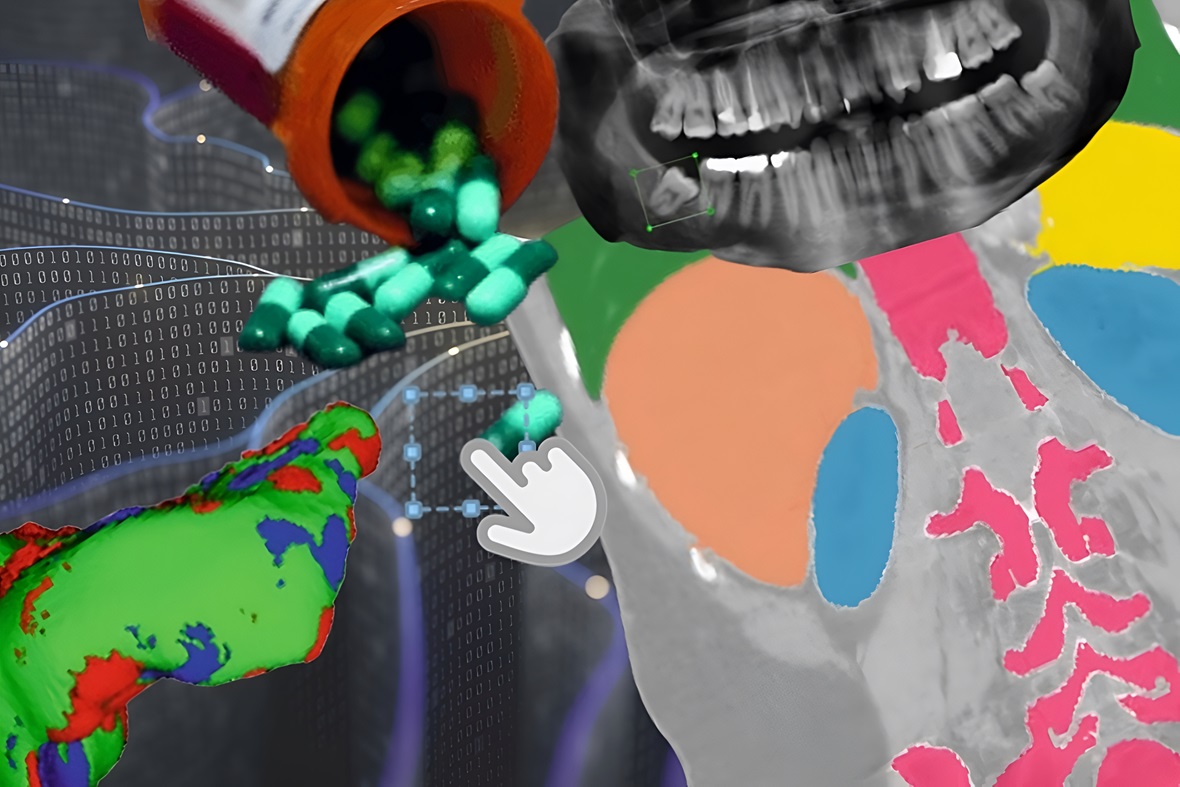
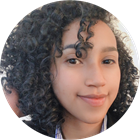
Artificial intelligence (AI) is rapidly transforming the medical field, in particular through the use of π specialized datasets for training predictive models. Advances in medical image analysis, automated diagnosis, or even patient record management rely heavily on the quality of available data.
β
Medical datasets play a big role in providing a solid foundation for training and refining these algorithms, enabling the accuracy of AI-based healthcare tools to be improved.
β
With this in mind, medical datasets offer a unique opportunity to advance AI research and development, while respecting the ethical and regulatory challenges inherent in the healthcare sector. Access to structured, reliable data is essential to guarantee results that are relevant and applicable to real clinical environments.
β
In this article, we tell you more about medical datasets, and suggest you discover 10 free medical datasets that will enable you to initiate your work developing AI products for healthcare. Follow the guide!
β
β
What is a medical dataset and why is it important for training AI models?
β
A medical dataset is a collection of healthcare data, such as medical images, diagnoses, or patient records. This data is essential for training AI models, as it enables algorithms to learn to identify patterns, make predictions, or propose diagnoses.
β
Datasets can therefore be used to improve the accuracy of AI tools in areas such as diagnostics, disease progression prediction and the automation of medical analyses.
β
β
Introduction to the use of medical data for AI
β
The use of medical data for artificial intelligence (AI) is a fast-growing field, offering unprecedented opportunities to improve medical research, healthcare and public health. Medical data, also known as health data, is information collected about patients, treatments, health outcomes and experiences. This data can be used to train AI models, which can then be used to predict treatment outcomes, identify disease risk factors and improve the quality of care.
β
Health data comes from a variety of sources, such as electronic medical records, public health databases, clinical studies and therapeutic trials. By analyzing this information, researchers can uncover trends and correlations that were previously invisible, paving the way for significant advances in the medical field. For example, AI can help identify patterns in health data that indicate an increased risk of certain diseases, enabling earlier intervention and more effective treatments.
β
In short, the integration of medical data into AI models represents a revolution in the way we approach health and care. It not only improves the accuracy of diagnosis and treatment, but also enables care to be tailored to the specific needs of each patient. This data-driven approach is essential for advancing medical research and optimizing public health systems.
β
The importance of data for medical research
Medical data is essential for medical research, enabling researchers to understand the underlying mechanisms of disease, develop new treatments and test their efficacy. Medical data can be collected from a variety of sources, including medical records, health databases, clinical studies and therapeutic trials. This information is important for answering specific questions, such as the prevalence of a disease, the effectiveness of a treatment or the risk factors associated with a pathology.
β
Using healthcare databases, researchers can develop AI models capable of predicting treatment outcomes, identifying disease risk factors and improving quality of care. For example, an AI model trained on healthcare data can help anticipate post-operative complications or optimize treatment protocols for chronic diseases. These models can analyze large amounts of data in real time, enabling healthcare professionals to make informed decisions and deliver high-quality care.
β
In short, medical data plays a key role in medical research and improving public health. They enable the development of AI models that can predict treatment outcomes, identify disease risk factors and improve the quality of care. By exploiting this data, researchers can not only answer specific questions but also improve our understanding of the underlying mechanisms of disease, paving the way for significant medical innovations.
β
β
β
β
β
β
β
What are the main use cases for open data medical datasets in the development of AI models?
β
Open data medical datasets are used in several use cases for the development of artificial intelligence (AI) models:
β
AI-assisted diagnosis
One of the most common uses is to train models capable of detecting diseases from series of medical images, such as X-rays, MRIs or CT scans. For example, algorithms are trained to identify cancer, heart disease or lung pathologies.
β
Predicting disease progression
Datasets containing clinical information can be used to develop predictive models to estimate the evolution of a disease in a patient. These algorithms help anticipate complications or risks associated with certain pathologies.
β
Genomic data analysis
Genomic data, such as that provided by databases like TCGA (The Cancer Genome Atlas), enable AI models to identify genetic mutations associated with disease, facilitating personalized treatment in oncology.
β
Treatment optimization
By analyzing data relating to medical prescriptions and treatment effects, AI models can suggest optimized therapeutic protocols, thereby reducing prescription errors or adverse reactions.
β
Public health research
Datasets like those in France's Système National des Données de Santé (SNDS) are used to study epidemiological trends, improve care planning and optimize the management of healthcare systems.
β
These use cases show how open data datasets, including tables representing data for public health analysis, are transforming AI in healthcare, enabling faster, more accurate and personalized decision-making.
β
β
How important is data diversity in medical datasets for AI?
β
Data diversity in medical datasets is essential to guarantee the reliability and fairness of artificial intelligence models. It enables algorithms to better generalize their results to different groups of patients, minimizing biases linked to age, ethnic origin or medical conditions.
β
This ensures that diagnoses and predictions are applicable to a wider population. In addition, diversified data reinforce the robustness of models, making them more suitable for a variety of situations and reducing the risk of medical errors in real-life contexts.
β
β
What are the best data sets for medical research?
β
Here is a selection of 15 of the most useful medical datasets for training artificial intelligence models in the healthcare field. They cover various aspects of medicine, from medical imaging to chronic disease data and prescriptions.
β
#1 - MIMIC-III
This is a hospital database containing anonymized information on intensive care patient admissions, including vital signals, prescriptions and clinical notes.
β
#2 - Chest X-ray Dataset
This is a large set of over 100,000 annotated chest X-ray images, used for automatic detection of lung diseases.
β
#3 - Open Access Series of Imaging Studies (OASIS)
It includes brain imaging datasets for studies of dementia and Alzheimer's disease, including MRI (magnetic resonance imaging) data.
β
#4 - UK Biobank
It is a vast biomedical database containing health data and biological samples from 500,000 UK participants, used for research into many diseases.
β
#5 - TCGA (The Cancer Genome Atlas)
This is a collection of genomic and clinical data on over 20 types of cancer, used for oncology research and personalized medicine.
β
#6 - PhysioNet
It's a collection of databases on physiological signals such as the electrocardiogram (ECG), enabling studies of heart disease and other conditions.
β
#7 - eICU Collaborative Research Database
It's an anonymized dataset from intensive care units (ICUs) across the U.S., for critical care studies and clinical trends.
β
#8 - MedNIST Dataset
This is a data set of medical images in radiology (MRI, CT, ultrasound), used for image classification algorithms.
β
#9 - CheXpert
This is another database of chest X-rays, with over 200,000 annotated images and diagnoses for several lung diseases.
β
#10 - Cancer Imaging Archive (TCIA)
This is an open resource containing medical images of patients with different types of cancer, for training cancer detection algorithms.
β
#11 - Open Bio
This is data on medical biology, covering millions of reimbursements for medical biology procedures, providing valuable information on trends in biological diagnosis and treatment in France.
β
#12 - Open Medic
Data on drug expenditure reimbursed in France, including detailed information on medical prescriptions.
β
#13 - Human Connectome Project (HCP)
This is data on human neuronal connections collected via MRI, enabling the study of π neural networks and their links with different cognitive functions.
β
#14 - PAD-UFES-20
This is a dataset for skin disease detection based on clinical images, used for the analysis of dermatological disorders.
β
#15 - SNDS (National Health Data System)
It is a French database covering a wide range of health data, including hospitalizations, prescriptions and consultations, widely used in epidemiological research and public health management.
β
These datasets provide a solid basis for training artificial intelligence models capable of diagnosing, predicting and managing various medical conditions.
β
β
Conclusion
β
In conclusion, the use of medical datasets in the development of artificial intelligence models paves the way for major advances in the healthcare field. These datasets, whether they relate to medical imaging, prescriptions or genomic data, enable us to improve the accuracy of diagnoses, personalize treatments and better understand the evolution of diseases.
β
Thanks to access to open data sources (available to the general public), the scientific community can train more efficient models, while respecting ethical and regulatory issues. Artificial intelligence, fed by this high-quality data, is thus an essential lever for making healthcare more effective and accessible.