Discover the 10 best multimodal datasets for smarter AI models

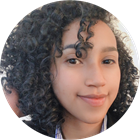
Recent advances in artificial intelligence (AI) are largely based on the integration of data from various modalities, such as images, text, audio and video.
This multimodal approach to datasets enables learning models to exploit complementary information, updating their ability to interpret and understand complex environments.
However, the diversity and richness of this data poses significant challenges, particularly in terms of management and processing. Dimensionality reduction, for example, an essential technique for simplifying these datasets while preserving their information content, plays a central role in optimizing the performance of AI models. But that's a subject for another day... let's start by explaining what a multimodal dataset is!
What is a multimodal dataset?
A multimodal dataset is a data set that contains several types of modalities or data formats, such as images, text, audio or video. A summary of these specific datasets supports multimodal processing research, exploring a variety of contexts.
Unlike datasets which focus on a single modality (for example, images or text alone), multimodal datasets integrate several complementary sources of information, enabling machine learning models to process and analyze a variety of data at the same time.
What are the specific advantages of multimodal datasets for image recognition and language understanding?
Multimodal datasets offer several specific advantages for image recognition and language understanding:
Contextual enrichment
By combining visual (images) and textual (language) information, models can better understand the overall context of a scene or image. For example, an image of a dog can be enriched with a caption that specifies the breed or the action performed by the dog. This gives models a more complete and accurate picture.
Reducing ambiguity
Integrating several modalities helps resolve ambiguities that might exist if one modality were used alone. For example, a simple image can be ambiguous in terms of interpretation, but the addition of explanatory text or questions and answers helps to clarify the visual content.
Improved model accuracy
Models using multimodal data combine the strengths of both types of information. This improves their accuracy in tasks such as image description generation(image captioning) or visual questioning(VQA), where models need to understand both visual content and text.
Deeper thinking skills
Multimodal datasets enable models to develop reasoning capabilities by cross-referencing information from different sources. For example, a model can learn to understand complex spatial relationships between objects in an image, while taking into account the semantic relationships provided by text.
Robustness in the face of data variations
By drawing on several types of data, multimodal models are more robust to variations or disturbances in one modality. For example, if the image is partially obstructed, the associated text can compensate for this lack of information and enable better interpretation.
Intelligent, interactive applications
Multimodal datasets are essential for the development of interactive systems such as virtual assistants or visual question-and-answer systems, which require an understanding of both visual and linguistic elements to effectively answer users' questions or provide detailed descriptions.
Enhanced natural language understanding
By combining images and text, models can better grasp the meaning of words in a visual context. This enables them to learn complex relationships between objects, actions and concepts, improving their understanding of natural languagein applications such as image translation and visual scene analysis.
💡 Ces avantages font des datasets multimodaux des outils puissants pour développer des modèles d'IA capables de mieux comprendre et interpréter le monde visuel et linguistique de manière intégrée. Une modification le jour même des données multimodales garantit que les modèles d'IA reçoivent toujours les informations les plus à jour.
What are the criteria for selecting a quality multimodal dataset?
To select a quality multimodal dataset, several criteria need to be taken into account to guarantee the relevance and efficiency of training artificial intelligence models. Here are the main elements to consider:
Diversity and richness of modalities
A good multimodal dataset should contain a variety of formats (images, text, audio, video) to cover complementary information. This diversity makes it possible to capture different aspects of the same reality and enrich the models' comprehension capacity.
Annotation quality
Files linked to annotations must be precise, consistent and exhaustive for each modality. For example, the use of unique identifiers makes it possible to link different information between modalities, facilitating data analysis and processing by AI models.
This is particularly useful for linking textual content to specific visual entities, improving the consistency of predictions.
Dataset volume and size
Dataset size is a key factor in ensuring optimal performance when training models. A large dataset, well annotated by experts, offers a better representation of real data, helping to minimize bias and improve model generalization.
Data variability and representativeness
A good multimodal dataset needs to cover a wide range of scenarios, environments, and data types to ensure that models can generalize to diverse and varied situations.
Accessibility and standards compliance
The dataset must be easily accessible and comply with ethical and legal standards, particularly in terms of data confidentiality. The availability of licenses and transparency regarding data collection and use are also important criteria.
Documentation and technical support
A quality dataset must be accompanied by a clear, detailed documentation file that explains collection methods, data formats, annotations and access methods. This documentation facilitates the integration of information into Machine Learning pipelines, and the updating of datasets to perfect models.
What are the 10 best multimodal datasets for training AI models?
Here's a list of the top 10 multimodal datasets for training AI models, based on their relevance to different application domains:
1. MS COCO (Common Objects in Context)
Un dataset très utilisé pour les tâches de détection d'objets, de segmentation et de génération de descriptions d'images. Ses auteur s y ont mis plus de 330'000 images avec des annotations détaillées. Il est particulièrement apprécié pour les modèles intégrant vision et langage.
2. Flickr30K Entities
Ce dataset est une extension du Flickr30K, combinant des images avec des descriptions textuelles riches. Il permet d’entraîner des modèles à mieux comprendre les relations entre les objets visuels et les concepts linguistiques.
3. Visual Genome
Ce dataset combine des images avec des descriptions de régions spécifiques, des relations entre objets et des questions-réponses visuelles. Il est utilisé pour des tâches comme le questionnement visuel (VQA) et la compréhension visuelle complexe.
4. YouTube-8M
Un vaste dataset multimodal qui inclut plus de 7 millions de vidéos annotées avec des métadonnées textuelles. Il est couramment utilisé pour la classification de vidéos et la reconnaissance d'actions, combinant vidéo et texte.
5. CLEVR (Compositional Language and Elementary Visual Reasoning)
Conçu pour évaluer les capacités des modèles à raisonner visuellement en utilisant des images synthétiques et des questions en langage naturel. CLEVR est utilisé pour des tâches de raisonnement visuel et spatial.
6. MuSe (Multimodal Sentiment Analysis)
Ce dataset est axé sur l'analyse des émotions, combinant information audio et visuelle. Il est utilisé pour la détection d'émotions et l'analyse des sentiments dans des applications telles que la détection de stress.
7. MovieQA
Spécialisé dans le questionnement visuel pour des vidéos, ce dataset associe des clips de films à des questions-réponses basées sur des dialogues et des actions. Il est particulièrement adapté pour des modèles combinant vision, langage et compréhension contextuelle.
8. MSR-VTT (Microsoft Research Video to Text)
C'est un code de dataset dédié à la génération de descriptions automatiques de vidéos. Il combine des vidéos avec des annotations textuelles, facilitant le développement de modèles pour le captioning vidéo.
9. VQA (Visual Question Answering)
Ce dataset permet de former des modèles capables de répondre à des questions en fonction de l'analyse d'images. Il est utilisé pour le développement de systèmes interactifs et intelligents, notamment dans l'assistance visuelle.
10. Open Images
Un des plus grands datasets d’images annotées, il contient 8 millions d’images avec des annotations pour la classification, la détection d’objets et la segmentation. Il est utilisé pour des tâches de vision par ordinateur, souvent combiné avec une origine fichiers d'annotations textuelles.
We hope you enjoyed this article 😊 ! If you can't find what you're looking for among these datasets, or are looking for a specific dataset, don't hesitate to contact us !