Large Action Model: How to redefine AI beyond verbal interactions
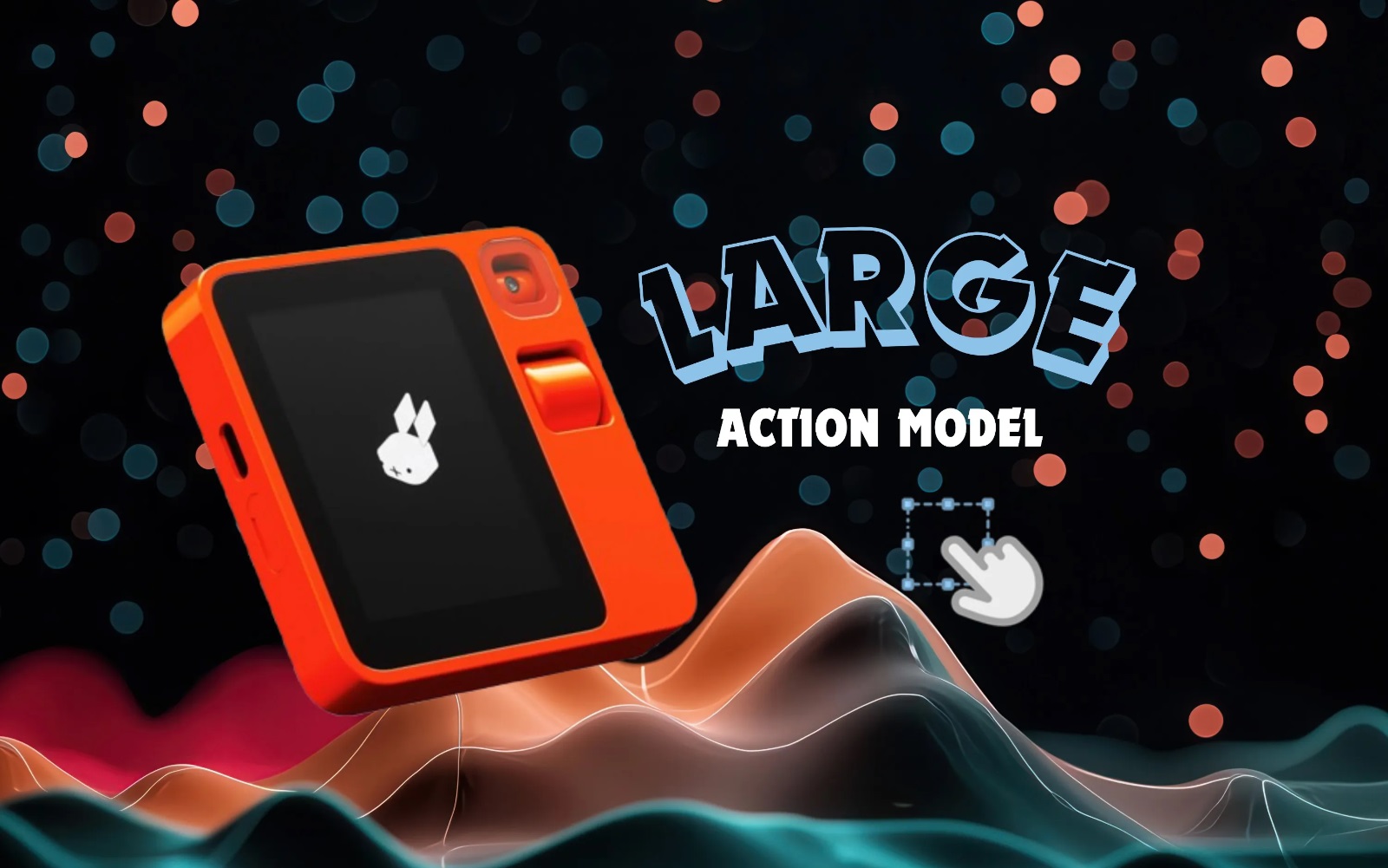
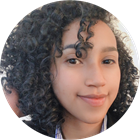
Recent advances in the field of artificial intelligence (AI) have led to an important milestone with the emergence of Large Action Models (LAMs). Unlike traditional models, which are mainly limited to language or image processing, these models aim to extend AI capabilities to more complex and practical actions.
β
Relying on complete and precise datasets (which bring together massive volumes of pre-processed/annotated data), LAMs enable machines to understand their immediate environment, to make autonomous decisions and to execute physical (in robotics) or virtual tasks with greater precision.
β
This approach, which transcends simple verbal interactions, redefines the way AI models are trained and used, opening up new perspectives in fields as diverse as robotics, autonomous driving and industrial process automation, by simplifying human interactions through a simple interface.
β
β
π‘ In short, LAM makes AI proactive. With LAM, it understands queries and responds with actions! We explain how it works in this article.
β
β
What is a Large Action Model?
β
A Large Action Model or LAM is an advanced type of artificial intelligence model designed to perform tasks that go beyond language processing or simple prediction. Unlike traditional models, which often specialize in analyzing textual or visual data, LAMs are capable of interpreting and acting on complex instructions in real or simulated environments.
β
They combine various data modalities - including text, images, movements and actions - to enable AI to interact autonomously with its environment, make decisions in real time and perform concrete tasks, whether manipulating physical objects or carrying out operations in a virtual context.
β
The training of these models relies on the annotation of large, complex data sets, integrating both human actions and specific contexts, to enable them to understand not only what to do, but also how to do it. These capabilities open up new perspectives in sectors such as robotics, autonomous vehicles and industrial process automation. What's more, an operating system based on LAM technology, such as the Rabbit OS, offers a unique user experience without the need for conventional applications.
β
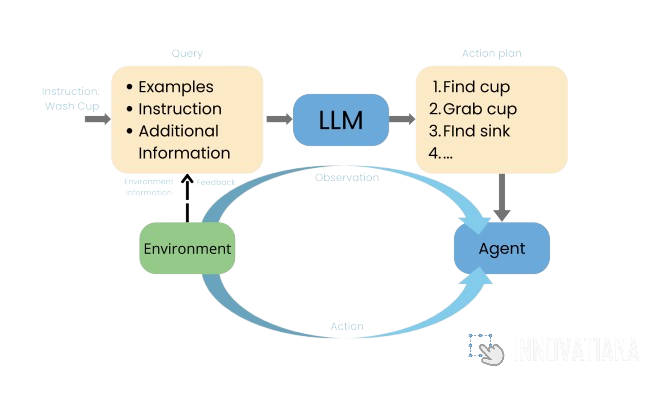
β
β
β
How does it differ from traditional artificial intelligence models?
β
Large Action Models differ from traditional artificial intelligence models in a number of ways, including their objectives, their complexity and their ability to interact with dynamic environments.
β
Scope of actions
While traditional AI models, such as those for natural language processing (NLP) or image recognition, focus primarily on analyzing and understanding static data (text, images, etc.), LAMs are designed to execute physical or virtual actions in response to complex contexts. They don't just process data, but actively interact with the environment.
β
Multi-modality
Unlike traditional models, which often process a single type of data (text, images or audio), Large Action Models are capable of combining several data modalities - for example, visual, textual and kinaesthetic (movement and action) data. This enables a more complete and contextual understanding, necessary to carry out complex actions, notably thanks to an optimized operating system.
β
Autonomous decision-making
Large Action Models are equipped with mechanisms enabling them to make decisions in real time and adjust their actions according to the results. Traditional models, on the other hand, focus more on predictions based on training data, and often require human intervention for final decision-making.
β
Task complexity
While traditional models are often limited to specific tasks (such as image image classification orsentiment analysis), Large Action Models are designed to handle much more complex and practical tasks, such as object manipulation in robotics, or navigation in physical and digital environments.
β
β
AI evolution with Large Action Models
β
Large Action Models (LAMs) represent a major advance in the field of artificial intelligence (AI). These innovative models are designed to understand and execute actions based on human intentions, revolutionizing the way we interact with technology.
β
Unlike traditional models, which focus primarily on analyzing static data, LAMs are capable of processing multi-modal information and making decisions in real time. This ability to integrate textual, visual and kinaesthetic data enables LAMs to perform complex actions and adapt to dynamic environments.
β
The evolution of LAMs has been made possible by significant advances in data processing and machine learning. Based on massive volumes of annotated data, these models can learn to perform tasks autonomously, without human intervention. This opens up new perspectives in a wide range of fields, from robotics and autonomous driving to healthcare and logistics.
β
LAMs are also redefining the way operating systems are designed, by integrating more intuitive and interactive interfaces. For example, projects such as the Rabbit R1 demonstrate how LAMs can be used to create robots capable of understanding and executing complex commands, improving efficiency and task precision.
β
β
πͺ In short, Large Action Models represent a key step in the evolution of artificial intelligence, enabling more natural and efficient interaction between humans and machines. These technological advances promise to transform many industrial sectors, automating ever more complex tasks!
β
β
β
How can Large Action Models be applied in industry?
β
Large Action Models have many applications in various industrial sectors, thanks to their ability to perform complex actions and interact autonomously with dynamic environments. We have put together a list of some of the most relevant application areas:
β
Industrial robotics
LAMs are used to automate complex tasks in production environments. They enable robots to manipulate objects, assemble components, or navigate workspaces without human intervention, while adapting to changes in real time.
β
Autonomous driving
In the automotive sector, these models play a key role in the design of autonomous vehicles. Thanks to their ability to interpret multiple data sources (cameras, sensors, radar), LAMs enable cars to make complex decisions in real time, such as traffic management, obstacle detection and navigation in urban environments.
β
Health and medical care
In medicine, Large Action Models can be used for surgical assistance by robots, where precise, coordinated actions are required. They are also applied in assistive robotics to help the elderly or disabled perform everyday tasks.
β
Logistics and supply chain
In the logistics sector, LAMs help to automate warehouse management, notably by enabling robots to move and organize goods, pack products or manage inventory with greater efficiency. They also optimize transport planning and management.
β
Manufacturing industry
These models facilitate the automation of production lines in factories, enabling real-time monitoring, maintenance and management of machines. They can adjust manufacturing processes according to variations in materials or production parameters.
β
Security and surveillance
In the security sector, Large Action Models can be used for real-time video analysis and proactive intervention when suspicious behavior is detected. They can also be integrated into autonomous surveillance systems to anticipate and react to potential threats, thanks to a user-friendly interface that simplifies interaction with these systems.
β
Entertainment and video games
In the video game industry, LAMs enable the creation of more intelligent non-player characters (NPCs), capable of reacting realistically to players' actions, thus enhancing interaction and immersion.
β
Agriculture
In agriculture, these models are used to automate repetitive tasks such as harvesting, planting and crop monitoring. Agricultural robots equipped with Large Action Models can assess the condition of plants and adjust their actions accordingly.
β
β
β
The importance of datasets in LAM training
β
Datasets are essential for training Large Action Models (LAMs). To date, two datasets can be used for this purpose: WorkArena (source) and WebLinx (sourceHowever, these datasets remain relatively limited in size. Although they include telemetry data, it is conceivable to train LAMs solely from video recordings, like a human following a YouTube tutorial to reproduce an action. This process is reminiscent of the method potentially employed by Tesla to train its autonomous driving systems from videos, without resorting to more complex technologies such as LiDAR.
β
β
β
β
β
β
β
Conclusion
β
Large Action Models represent a significant advance in technology and artificial intelligence, extending the capabilities of traditional models to include concrete, autonomous actions.
β
Thanks to their ability to integrate multi-modal data and make decisions in real time, these models are redefining the field of possibilities in the world of artificial intelligence, enabling applications in sectors as varied as robotics, healthcare and logistics.
β
As these technologies continue to develop, they offer promising prospects for the automation of increasingly complex tasks, and could transform many industries in a sustainable way. However, their large-scale deployment still requires overcoming technical, ethical and regulatory challenges, to maximize their impact in a responsible manner.