Human pose estimation: technology at the heart of Computer Vision

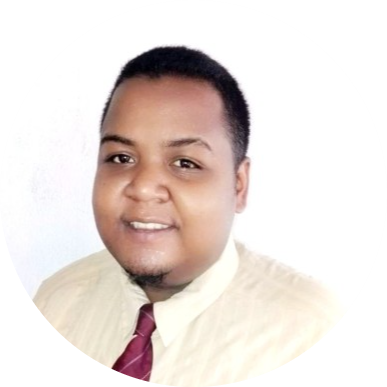
Have you ever heard of the concept of human pose estimation? To tell the truth, human pose estimation is a rather advanced technology in π Computer Vision. It detects and analyzes the position of human body joints from images or π videos. Models play a fundamental role in neural networks for predicting the position of joints in the human body. This technology has a wide range of uses, from video games to healthcare, augmented reality, robotics and, of course, sport! In this article, we've tried to explore the subject of human pose estimation to help you fully understand this important concept in artificial intelligence.
β
To begin with, remember that human pose estimation uses sophisticated algorithms to recognize shapes and movements. These algorithms are often based on deep neural networks and machine learning. They can accurately identify the positions of different body parts, even under complex conditions. Find out more below!
β
β
How does human pose estimation work?
β
As mentioned in our introduction, human pose estimation is a technology that aims to detect and analyze the position of the joints of the human body through one or more images or videos, thus enabling a person's posture to be detected. It identifies key points on the body, such as :
. shoulders ;
. elbows ;
. wrists ;
. hips ;
. knees ;
. ankles.
β
She then connects them to form a virtual skeleton. This process enables us to understand human postures and movements with great precision.
β
Computer Vision and machine learning algorithms are at the heart of human pose estimation. Neural network models are used to predict the position of human body joints.
β
Here are the key steps in the process:
β
Image pre-processing
The image or video is first pre-processed to improve quality and extract relevant features. This can include color normalization, noise filtering, and brightness and contrast adjustment.
β
Detecting key points
Algorithms identify key points on the human body. Detecting human poses in non-cooperative environments, such as occlusion and clothing variations, presents significant challenges. This can be done using π convolutional neural networks (CNN). These are trained on π annotated datasets containing images of people with markers on their joints.
β
Skeleton construction
Once the key points have been detected, they are linked to form a virtual skeleton. This visualizes the human pose in terms of articular segments.
β
Installation analysis
The virtual skeleton is then analyzed to understand postures and movements. Top-down and bottom-up approaches to human pose estimation are often used to address the complexity of the human body and the challenges associated with posture detection. These can include joint angle assessment, gesture detection and action recognition.
β
Post-processing
Pose data can be filtered and smoothed to correct any errors and improve accuracy. The results can then be used in various fields, such as animation, gesture recognition or biomechanical analysis.
β
β
β
β
β
β
β
β
What are the fields of application of human pose estimation?
β
Human pose estimation has found varied applications in many fields, thanks to its ability to analyze and interpret human movements with precision. Specific techniques used in these fields include artificial intelligence, computer vision and various machine learning methods. Here are some of the main areas of use:
β
Augmented reality (AR) and virtual reality (VR)
- Natural interaction: Augmented reality and virtual reality systems use human pose estimation to enable more intuitive and immersive interactions. Users can manipulate virtual objects or navigate virtual environments through body movement.
- Full-body tracking: Advanced virtual reality applications use this technology to track the user's entire body, enhancing the immersive experience.
β
Video games
- Motion capture: Human pose estimation is used to capture player movements in real time, enabling direct interaction with the game without physical controllers.
- Enhanced user experience: Video games can become more engaging and interactive by using gesture recognition to control in-game actions.
β
Health, fitness and sport
- Biomechanical analysis: Healthcare professionals use this technology to analyze patients' body mechanics, helping to diagnose and treat musculoskeletal disorders.
- Posture correction: fitness applications use pose estimation to correct posture and provide real-time feedback during exercise.
- Rehabilitation : It helps monitor patients' progress in physical rehabilitation by analyzing their movements and adjusting treatments accordingly.
- Performance analysis: Athletes and coaches use pose estimation to analyze techniques and performance, identifying areas for improvement.
- Injury prevention: It helps monitor athletes' movements to prevent injury by detecting at-risk postures.
β
Security and surveillance
- Detecting suspicious behavior: Surveillance systems can use pose estimation to identify abnormal or suspicious behavior in public places, helping to prevent incidents.
- Scene analysis: In industrial environments, it is used to monitor workers' postures and prevent accidents linked to incorrect postures.
β
Social and interactive robots
- Human-robot interactions: Social robots use human pose estimation to understand and imitate human gestures, enhancing human-robot interactions.
- Navigation aid: robots can use this technology to avoid collisions with humans by detecting and interpreting their movements.
β
Arts and entertainment
- Animation and film: Animation studios use pose estimation to capture the movements of actors and transfer them to virtual characters.
- Live performances: It enables real-time special effects and innovative interactions in shows and artistic performances.
β
β
π‘Human pose estimation is a versatile technology that continues to evolve, opening up new possibilities in a wide range of sectors thanks to its advanced capabilities for analyzing and interpreting human movements.
β
β
Conclusion
β
Human pose estimation is an innovative technology that is revolutionizing the way computers interact with the physical world. It opens up new perspectives in many sectors, and its adoption can lead to significant innovations and enriched user experiences.
β
Despite certain advances, human pose estimation still has limitations in terms of accuracy, robustness and real-time processing. There are different types of approach to human pose estimation, includingtop-down andbottom-up methods, each with its own advantages and disadvantages. However, rapid progress in the field of artificial intelligence promises continued improvements in the near future, paving the way for diverse new possibilities and applications.