How are AI and massive data annotation reinventing medicine?
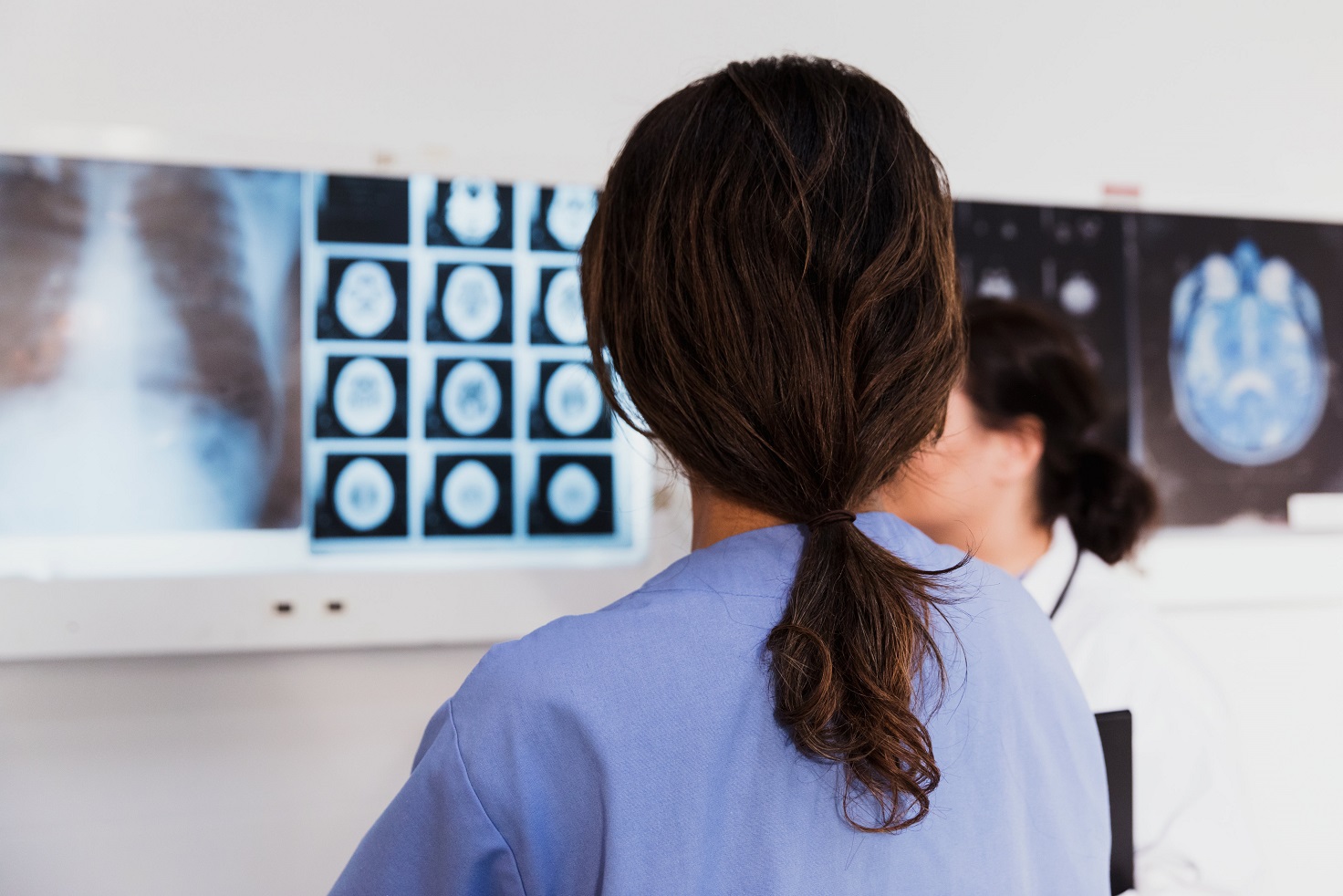
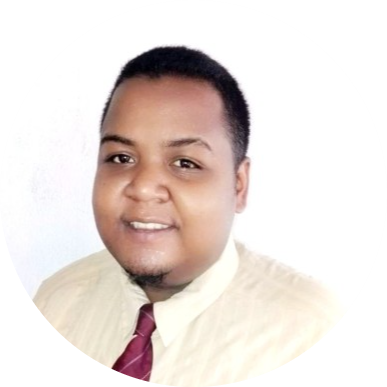
Artificial intelligence (AI) has gradually conquered many sectors, transforming the way we work, communicate and interact with our environment.
β
In the medical field, it is playing a growing role in improving diagnosis, treatment and research. An essential component of AI in medicine is Data Labelingwhich consists in annotating voluminous medical data (mainly images, such as X-rays or scans, but also videos of medical operations, for example) to train machine learning algorithms.
β
β
π‘ In this article, we explore the importance of Data Labeling and the place ofAI in the medical fieldusing real-life examples.
β
β
β
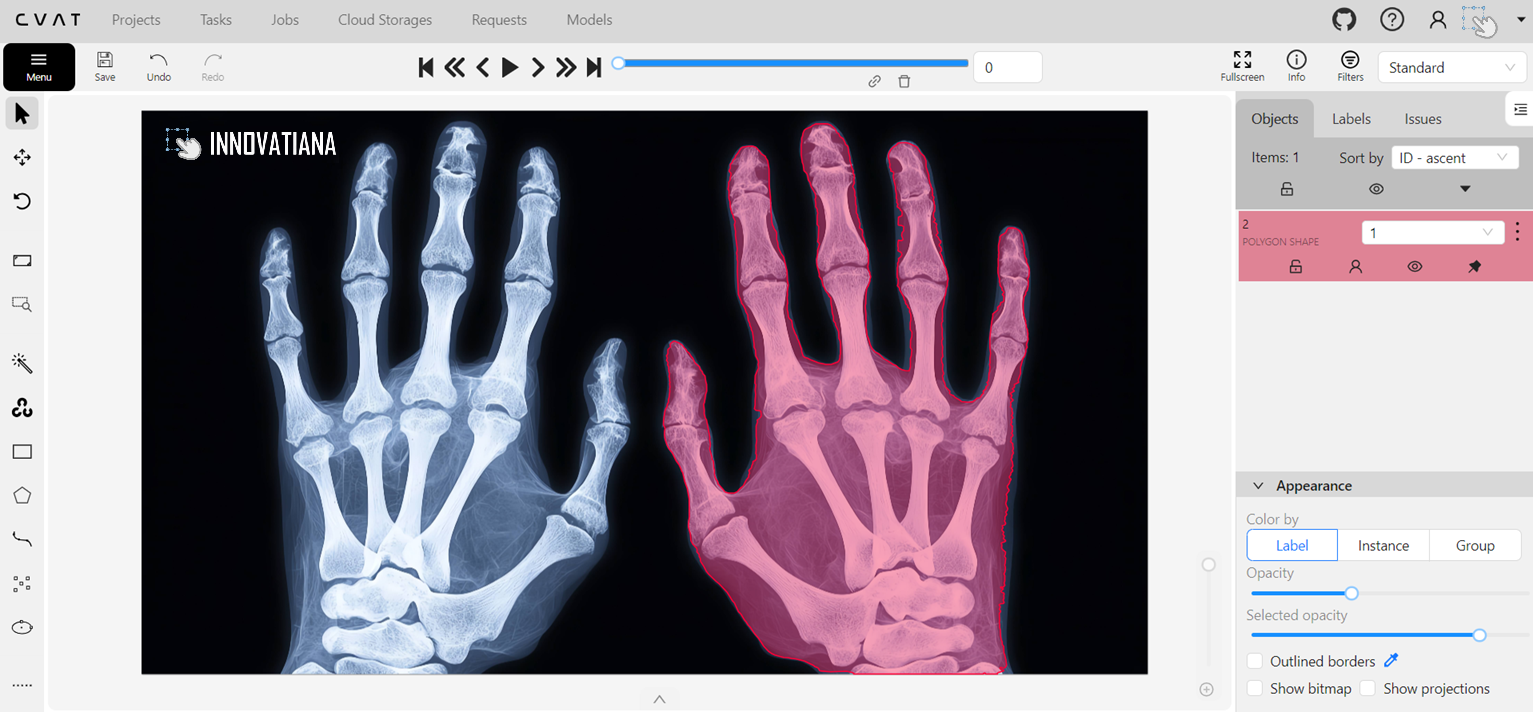
β
β
The role of the medical annotator in Data Labeling: the key to AI models for medicine
β
As you know if you read us, Data Labeling is the process of annotating data - in this case medical data - to make sense of it for AI algorithms. Data annotation is a necessary component of the medical AI development cycle, as it helps prepare the high-quality training data required for the models.
β
Data annotation is essential for improving AI models, particularly in the healthcare sector and in the analysis of clinical data. Understanding the cause of symptoms is necessary in any medical decision-making process. It is almost impossible for an AI model, and especially dangerous, to make a medical decision autonomously.
β
Also, a medical annotator plays an important role in the manual annotation of data for medical use cases, helping to overcome the challenges associated with the large quantities of data that need to be annotated for AI models to generalize and become operational.
β
For example, in the case of medical images, one of the main challenges in medical AI is to build up voluminous data sets in which regions of interest, instruments, anomalies, etc. are identified, in order to exploit the full value offered by medical imaging and, sometimes, detect weak trends to anticipate diagnoses.
β
Below, we present some of the contributions of medical annotation and AI to medicine:
β
Annotation of unused medical data: improving diagnosis
β
AI enabled by supervised learning models and the use of labeled data sets demonstrates excellent results and high accuracy in medical diagnosis and medical records management.
β
Image annotation helps create high-quality annotated medical datasets for machine learning.
β
Data annotation helps improve diagnostics by providing the precise, structured information needed to train machine learning models. Different types of data, such as medical images and patient records, are used to improve diagnostics.
β
Studies have shown that AI algorithms can detect specific pathologies, such as breast cancer and eye disease, with comparable or even higher accuracy than human experts. For example, a study by researchers at Stanford University showed that AI was as accurate as dermatologists in detecting skin cancers.
β
β
AI products to facilitate access to quality healthcare
β
AI is also helping to improve access to healthcare, particularly in remote or disadvantaged areas. Thanks to mobile apps and connected devices, patients can benefit from preliminary diagnoses, medical advice and treatment recommendations, even when there are no doctors on site.
β
In this way, AI makes it possible to bridge care gaps and provide medical services to more people, especially in medical deserts!
β
β
Medical research and development of drugs or treatments
β
AI also facilitates medical research by enabling the analysis of large datasets. Medical imaging enables the creation of visualizations of specific organs, which is useful in diagnosing unusual "defects" in the human body.
β
AI is also used to evaluate the results of medical treatments, or the evolution of a disease. For example, AI has been used to develop predictive models of the evolution of Alzheimer's disease, enabling earlier intervention and improved clinical outcomes.
β
β
AI and the training of healthcare professionals: an innovative pedagogical tool
β
Artificial intelligence also plays an important role in the training of healthcare professionals. AI-powered virtual simulations and learning platforms enable medical students and healthcare professionals to practice medical procedures in a controlled, safe environment.
β
For example, AI-assisted surgical simulations offer hands-on training without risk to patients, while enabling students to receive instant, personalized feedback on their performance. These pedagogical tools are revolutionizing the way future doctors are trained.
β
β
Managing patient flows and hospital resources optimized by AI
β
AI is also helping to improve the operational efficiency of hospitals by optimizing the management of patient flows and resources. AI algorithms can predict peak demand in emergency departments, enabling better allocation of human and material resources.
β
What's more, AI helps to plan surgical procedures and manage hospital beds more efficiently, reducing waiting times and improving the patient experience. This optimization is essential to ensure that healthcare facilities run smoothly and efficiently, especially in times of high demand.
β
β
Ethical challenges surrounding medical annotation and the regulation of AI in medicine
β
As artificial intelligence continues to transform the medical field, it's important to consider the ethical and regulatory implications associated with its use. Patient data confidentiality, informed consent and equity of access to AI technologies are major concerns.
β
Robust regulatory frameworks are essential to ensure that AI is used ethically and securely. Initiatives such as the GDPR (General Data Protection Regulation) in Europe aim to protect personal data and build trust in the use of AI in medicine.
β
β
Future prospects for AI in personalized medicine
β
One of the most promising areas for AI in medicine is personalized medicine. By analyzing patients' genomic data, medical histories and lifestyles, AI can help develop tailor-made treatments that are better suited to individual needs.
β
For example, targeted therapies can be designed to treat specific cancers based on the patient's genetic mutations. This personalized approach promises to dramatically improve treatment efficacy and reduce side effects, offering patients a better quality of life.
β
β
Medical annotator: its role, and how the use of AI is a revolution for medicine...
β
AI and Data Labeling are playing an increasingly important role in the medical field, offering promising opportunities to improve diagnosis, treatment and research.
β
Using a specialized medical annotator, often a doctor himself or at least a healthcare professional, medical imaging data can be labeled to train machine learning models and improve the quality of medical datasets for various healthcare applications.
β
Image annotation plays a critical role in the creation of medical datasets for machine learning, enabling the development of more accurate models. It involves associating metadata with the raw data available, to extract the maximum amount of information.
β
Thanks to the analysis of large quantities of data, AI enables more personalized, faster and more accessible medicine. However, it is essential to guarantee the quality of the data used to train AI models, paying particular attention to ethics, medical confidentiality and data security.
β
β
β
β
β
β
An overview of Innovatiana's medical data labeling services
β
At Innovatianawe offer medical data labeling services designed to enhance the accuracy and efficiency of AI models in the healthcare field. We use data annotation to support our customers in the healthcare and pharmaceutical industries in the development of innovative products.
β
We specialize in the meticulous annotation of medical images, videos, text and audio data, drawing on our in-depth expertise to train state-of-the-art machine learning algorithms. Our team is made up of doctors, both specialists and generalists, who work at our customers' request on medical use cases, such as annotating X-rays, prescriptions or videos of medical operations.
β
By intervening early in AI development cycles, our annotations help create products to improve diagnostics, treatment planning and patient care, ensuring reliable, high-quality data for advanced technology applications in medicine. Put your trust in Innovatiana to develop your medical AI, and get better results for your patients!
β
β
π‘ Here are just a few examples of the medical annotations we've produced for our services. Follow the guide!
β
β
How are medical data and associated metadata used? A few examples
β
Text data annotation for medical research
The annotation of textual data plays an essential role in medical research and the improvement of health information systems. At Innovatiana, we specialize in the annotation of medical documents such as pathology reports, clinical notes and scientific publications.
β
By labeling these texts, we enable AI models to understand and extract key information, facilitating research and the discovery of new treatments. For example, analysis of large text databases can reveal correlations between symptoms and rare diseases, opening up new avenues for diagnosis and treatment.
β
Image annotation for early disease detection
Medical image annotation is a tool for developing AI for early disease identification. Innovatiana works with medical experts to annotate X-ray images, CT scans and MRI scans, identifying subtle features of diseases in their early stages.
β
For example, in the early detection of lung cancer, our precise annotations enable AI models to spot lung nodules as soon as they appear, well before they become symptomatic. This early detection is vital for increasing the chances of cure and improving patient outcomes.
β
Video annotation for surgical training
Innovatiana's annotated surgical videos are a valuable resource for training surgeons. We segment and annotate every step of surgical procedures, providing detailed datasets for training AI models.
β
These models can then be used to create realistic simulations and interactive training tools for medical students and surgeons in training. For example, a video of a coronary bypass operation can be annotated to show the different stages of the procedure, the instruments used and the techniques employed, providing in-depth, hands-on training.
β
Audio data annotation for medical voice assistants
Annotating audio data is essential for the development of medical voice assistants. At Innovatiana, we annotate audio recordings of medical consultations, doctors' dictations and discussions between patients and healthcare professionals.
β
These annotations enable AI models to understand and process medical language, facilitating the development of accurate and reliable speech recognition systems. For example, a medical voice assistant can transcribe and analyze discussions during a consultation, offering valuable support to doctors by generating summaries and alerting them to key points to check.
β
Data annotation for clinical trial management
For example, in a clinical trial of a new diabetes treatment, our accurate annotations of blood glucose data and side effects enable AI models to detect trends and anomalies more quickly, facilitating real-time adjustments and improving treatment safety and efficacy.
β
DICOM image annotation for enhanced diagnostic accuracy
Images DICOM (Digital Imaging and Communications in Medicine) images are essential in the medical field for the storage and transmission of radiological images. At Innovatiana, we offer specialized services for annotating DICOM images, enabling AI models to be trained with high-quality data.
β
By meticulously labeling the various features of DICOM images, such as organ contours, abnormalities and anatomical structures, we help improve diagnostic accuracy. For example, in lung tumor detection, annotating DICOM images enables AI algorithms to accurately differentiate between healthy and pathological tissue, increasing the reliability of diagnoses and treatment plans.
β
Multimodal data annotation for a holistic view of patients
At Innovatiana, we understand the importance of combining different data modalities to gain a holistic view of patient health. Our services include multimodal data annotation, integrating images, text and audio data to create comprehensive datasets.
β
This approach enables AI models to analyze information more coherently and contextually. For example, when monitoring patients with chronic diseases, the integration of imaging data, clinical notes and voice recordings can provide a comprehensive overview, facilitating more personalized and efficient care management.
β
β
Other use cases for AI in medicine, enabled by medical data annotation
β
AI-assisted diagnosis in radiology
Take the case of a hospital using AI to diagnose bone fractures from X-rays. Image annotation helps train AI algorithms to diagnose bone fractures by labeling medical imaging data.
β
Thanks to our annotation service, each radiograph is carefully labeled to identify fractures, fracture lines and areas of calcification. AI algorithms, trained with this precise data, can then analyze new radiographs and detect fractures with an accuracy comparable to that of experienced radiologists.
β
For example, when it comes to detecting complex fractures such as those of the wrist (Colles' fracture), AI can spot subtle details and provide fast, reliable diagnoses, which is crucial for fast, effective patient care.
β
Optimizing surgical planning
In the field of neurosurgery, AI is used to plan complex surgical procedures. Using our video annotation service, videos of previous surgeries are segmented and classified according to the different stages of the operation. This enables AI models to understand surgical procedures and provide recommendations on the best surgical approach.
β
For example, when removing brain tumors, AI can analyze videos and suggest optimal paths to minimize damage to healthy brain tissue, thereby improving post-operative outcomes and reducing complications.
β
Improved cardiological diagnosis
For cardiologists, AI can transform the analysis of echocardiographic images. Thanks to our specialized cardiology annotation service, each image is tagged by experts to identify cardiac structures and abnormalities such as valvular insufficiency or cardiomyopathy.
β
AI algorithms trained with this data can detect cardiac abnormalities with increased accuracy, enabling cardiologists to diagnose and treat heart disease more effectively. For example, in the case of hypertrophic cardiomyopathy, AI can identify asymmetric thickening of the heart muscle, facilitating early intervention.
β
Automated transcription of medical consultations
AI makes it easier to automate the transcription of medical consultations, improving the efficiency and accuracy of medical documentation. Thanks to advanced speech recognition systems, conversations between doctors and patients can be automatically transcribed in real time, reducing the administrative burden on healthcare professionals.
β
For example, during a consultation, AI can capture and transcribe discussions about symptoms, diagnoses and treatment plans, enabling doctors to focus more on patient care.
β
Diagnosis of dermatological diseases
AI helps diagnose dermatological diseases by analyzing skin images to detect conditions such as melanoma, eczema and psoriasis. AI-enabled mobile apps enable patients to take photos of their skin lesions and receive a preliminary assessment.
β
For example, AI can compare images with a database of known dermatological conditions and provide recommendations to dermatologists, improving diagnostic accuracy and speed of treatment.
β
β
In conclusion
β
Innovatiana's medical data annotation services cover a wide range of healthcare needs, from medical research to surgical training. By collaborating with medical experts, we guarantee accurate and reliable annotations, essential for the development of high-performance AI models.
β
Whether it's for the early detection of disease, the improvement of speech recognition systems or the creation of innovative training tools, our annotation services play an important role in the advancement of medical technologies and the creation of tomorrow's medical technologies!
β
Put your trust in Innovatiana to transform your medical data into actionable insights for AI, and thus improve the quality of healthcare.
β
To go further and understand the importance of medical annotation in AI
β
The future of medicine is inextricably linked to its application of AI, and it is imperative to continue research and collaborations to fully exploit its potential for the benefit of everyone's health!
β
Impact companies such as SDSC (https://www.surgicalvideo.io/), whose aim is to build a global medical data platform to provide knowledge to surgeons at all levels of training, worldwide.
β
To find out more about our image and video annotation services for medical use cases, please visit our page dedicated toAI in the healthcare industry !