Our selection of the best datasets for developing artificial intelligence for agriculture
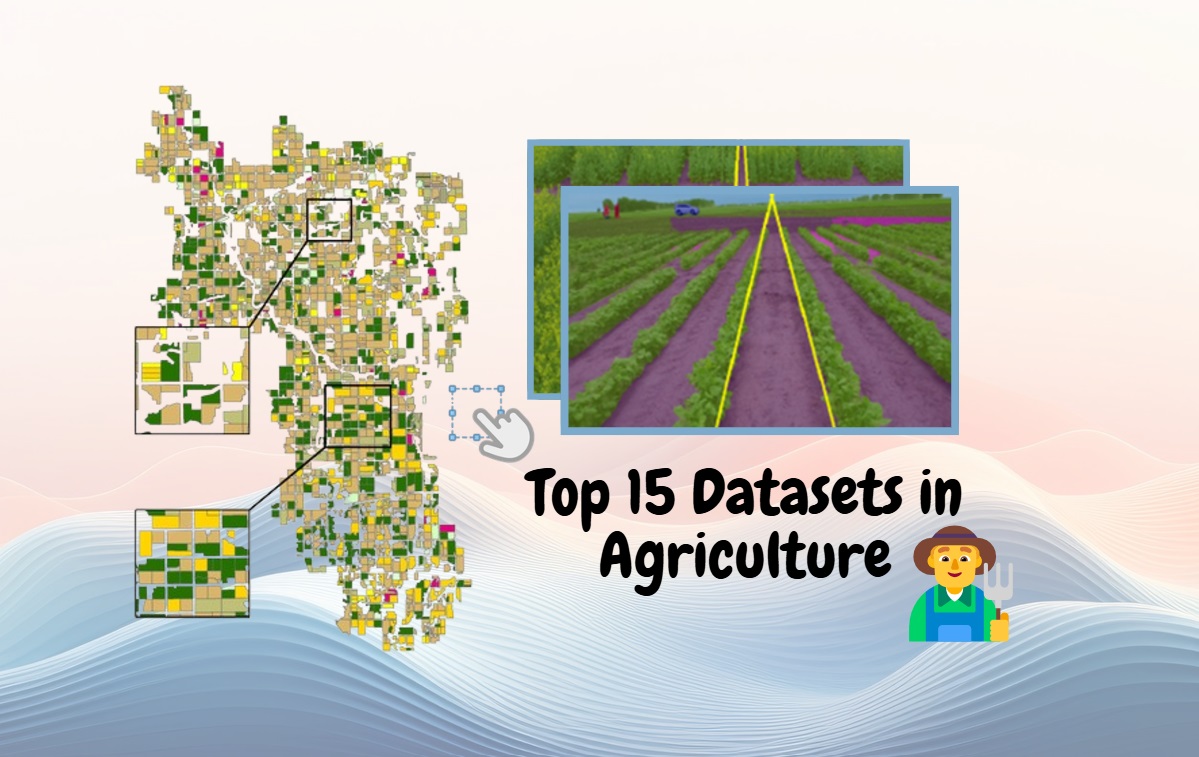
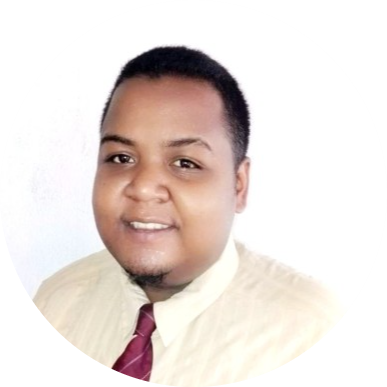
Modern agriculture is being transformed by the integration of technologies such as artificial intelligence and computer vision. At the heart of this revolution are agricultural datasets, essential for training models and developing innovative solutions. Here's a selection of the 15 best datasets that can help advance research and technological application in the agricultural field.
Dataset types in Agriculture
1. Images of crops and diseases
These datasets contain images of healthy and diseased plants, enabling computer vision models to detect and diagnose crop diseases. For example, the PlantVillage Dataset offers a vast collection of images for various crops and diseases.
2. Soil data
Including information on soil composition, texture and fertility, these datasets help optimize fertilizer use and improve land management.
3. Meteorological data
Real-time and historical climate data are important because they can be used to forecast crop yields and plan farming activities.
4. Satellite imagery
The 🔗 satellite images provide an overview of fields, helping to monitor crop health, vegetation cover and anomaly detection.
5. Performance data
These data enable us to analyze crop productivity as a function of factors such as farming practices, soil conditions and climate.
Our top 15 best datasets for agriculture
1. PlantVillage Dataset
Description : One of the largest datasets of leaf images of healthy and diseased plants, covering 38 disease classes on 14 different crops.
Applications : Detection and classification of plant diseases using computer vision.
Link : 🔗 PlantVillage
2. Agriculture-Vision Dataset
Description : Includes over 94,000 annotated aerial images of agricultural fields with various anomalies such as weeds, dry areas and insect damage.
Use : Detection of crop anomalies to improve agricultural monitoring.
Link : 🔗 Agriculture-Vision
3. Open Images Dataset for Agriculture
Description : A subset of the Open Images Dataset specially annotated for agricultural objects, including machinery, farm animals and crops.
Application : Recognition and 🔗 detection of agricultural objects in images.
Link : 🔗 Open Images
4. Sentinel-2 Satellite Imagery
Description : High-resolution multispectral satellite imagery dataset provided by the European Space Agency, covering the entire globe.
Applications : Crop monitoring, plant health analysis, creation of a map for thematic soil and crop mapping.
Link : 🔗 ESA Sentinel-2
5. Soil Moisture Active Passive (SMAP) Dataset
Description : Satellite data providing accurate soil moisture measurements worldwide.
Applications : Irrigation management, drought forecasting, climate modeling.
Link : 🔗 NASA SMAP
6. FAO Statistical Database (FAOSTAT)
Description : FAO's global statistical database on agriculture, including production, trade, prices and land use.
Applications : Analysis of global agricultural trends, economic research, and assessment of the impact of the Common Agricultural Policy on farming practices and land use.
Link : 🔗 FAOSTAT
7. USDA National Agricultural Statistics Service (NASS)
Description : Detailed data on agriculture in the United States, including yields, cropping practices and economic statistics. USDA NASS provides essential services by collecting and disseminating these agricultural data.
Applications : Market research, agricultural planning, policy analysis.
Link: 🔗 USDA NASS
8. DeepWeeds Dataset
Description : A dataset of over 17,000 annotated images of common weeds in Australian agricultural environments.
Applications : Development of automatic weed detection systems.
Link : 🔗 DeepWeeds
9. European Soil Database (ESDB)
Description : A detailed database of European soil characteristics, including texture, chemical composition and physical properties.
Use : Sustainable soil management, land use planning.
Link : 🔗 ESDAC
10. Radiant MLHub Agriculture Datasets
Description : A platform offering open datasets for machine learning in Earth observation, with a focus on agriculture. Radiant MLHub offers open data for machine learning applications, particularly in agriculture.
Applications : Crop classification, change detection, plant health analysis.
Link : 🔗 Radiant MLHub
11. Crop Yield Prediction Dataset (Kaggle)
Description : Data including historical crop yields, weather conditions and soil characteristics.
Applications : Modeling and prediction of crop yields.
Link : 🔗 Kaggle Crop Yield Prediction
12. Global Food Prices Database
Description : Data on world food prices, collected by the World Food Program. The dataset includes data on the share of different food items in world food prices.
Applications : Economic analysis, food safety studies.
Link : 🔗 WFP Data
13. CropDeep Dataset
Description : A collection of images for crop recognition and disease detection, covering several plant species.
Applications : Crop classification, disease diagnosis.
Link : 🔗 CropDeep
14. CGIAR Big Data Platform
Description : Open datasets on agriculture in developing countries, covering crops, climate, soil and more.
Applications : Research into sustainable agriculture, adaptation to climate change.
Link : 🔗 CGIAR Platform
15. UCI Machine Learning Repository - Mushroom Dataset
Description : Detailed data on the physical characteristics of various mushroom species.
Use : species classification, toxicological studies.
Link : 🔗 UCI Mushroom Dataset
Where to find Datasets for agriculture?
Finding the right dataset for your agricultural projects can be a challenge. Here are some sources and platforms where you can access a variety of agriculture datasets:
Specialized Platforms
- Kaggle: An online platform that hosts a multitude of public datasets, including those related to agriculture. You can find data for computer vision, predictive analysis and more.
Link: 🔗 Kaggle datasets
- Radiant MLHub: Provides open datasets for machine learning in Earth observation, focusing on agriculture and environmental sustainability.
Link : 🔗 Radiant MLHub
Research institutions and universities
- CGIAR: The International Consortium for Agricultural Research offers an open data platform that facilitates access to a multitude of agricultural datasets, particularly in developing countries.
Link : 🔗 CGIAR Big Data Platform
- INRAE: France's National Research Institute for Agriculture, Food and the Environment publishes data and resources for agricultural research.
Link : 🔗 INRAE Data
Governmental and international organizations
- FAO: The Food and Agriculture Organization of the United Nations provides a variety of global statistical datasets on agriculture via its FAOSTAT database.
Link : 🔗 FAOSTAT
- USDA: The United States Department of Agriculture provides detailed data sets on American agriculture, useful for comparative analysis and market research.
Link: 🔗 USDA NASS
Online communities and social networks
- GitHub: Many researchers and developers share their datasets and source code on GitHub, which can be an excellent resource for finding specific data.
Link: 🔗 GitHub
- Specialized forums: Platforms like Stack Overflow, Reddit or LinkedIn groups can be useful for asking for dataset recommendations or sharing resources with the community.
Conclusion
Access to high-quality datasets is key to driving innovation in agriculture. The agricultural datasets presented in this article offer valuable resources for the research and development of technological solutions aimed at improving productivity, sustainability and efficiency in the agricultural sector. By harnessing this data, agri-food leaders and innovative startups are helping to meet the global challenges of food security and climate change.