Discover Small Language Models (SLMs): towards lighter, more powerful Artificial Intelligence

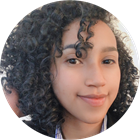
Rapid progress in the field of artificial intelligence has given rise to increasingly complex language models, capable of processing massive amounts of data and carrying out varied tasks with greater precision.
β
However, these large-scale language models, while efficient, pose challenges in terms of computing costs, energy consumption and ability to be deployed on limited infrastructures. It is in this context that "small language models" (SLMs) are emerging as a promising alternative.
β
By reducing model size while maintaining competitive performance, these lighter models offer a solution for resource-constrained environments, while meeting growing demands for flexibility and efficiency. What's more, SLMs deliver greater long-term value thanks to their increased accessibility and versatility.
β
β
A quick reminder: how are language models developed?
β
A) Pre-trained models
Language models are trained on self-supervised tasks based on large corpora of unlabeled text. For example, in the language masking task, a fraction of the tokens in the original text are randomly masked, and the language model attempts to predict the original text.
β
B) Fine-tuned models
(Pre-)trained language models are often tuned to specific tasks with labeled text, via a standard supervised learning approach. Fitting is generally much faster and offers better performance than training a model from scratch, especially when labeled data is scarce.
β
β

β
β
What is a Small Language Model (SLM)?
β
A Small Language Model (SLM) is an artificial intelligence (AI) model designed to process π natural language in English and other languages, similar to large language models (LLMs), but with a smaller, optimized architecture.
β
Unlike LLMs, which can require billions of parameters to operate, SLMs are designed with fewer parameters while maintaining acceptable performance for a variety of linguistic tasks.
β
This reduction in size makes SLMs more resource-efficient, faster to train and deploy, and better suited to environments where computing power and memory are limited.
β
Although their processing capacity is reduced compared to large models, SLMs remain powerful for specific tasks, especially when optimized with π high-quality annotated data and advanced training techniques. What's more, compact, high-performance tools make these models easy to access and use, making their adoption more accessible for businesses without requiring in-depth technical expertise.
β
β
β
β
β
β
β
What are the advantages of SLMs over larger models?
β
Small Language Models (SLMs) offer several advantages over large language models (LLMs), particularly in contexts where resources are limited or speed is essential. Here are the main advantages of SLMs:
β
Less resource-hungry
SLMs require less computing power, memory and storage space, making them easier to deploy on resource-constrained devices such as smartphones or embedded systems.
β
In addition, small language models show notable effectiveness in learning scenarios π zero-shotlearning scenarios, achieving comparable or even better results in some text classification tasks.
β
Reduced training costs
Thanks to their smaller size, SLMs can be driven faster and more cost-effectively, reducing energy and IT infrastructure costs.
β
Processing speed
A lighter architecture enables SLMs to perform tasks faster, which is essential for applications requiring real-time response (e.g. a chatbot).
β
Flexible deployment
SLMs are better suited to diverse environments, including mobile platforms and embedded systems, where large-scale models are not viable due to their high resource requirements.
β
Durability
Driving large language models is associated with high energy consumption and a significant carbon footprint. SLMs, with their reduced need for resources, contribute to greener solutions.
β
Optimization on specific tasks
Although smaller, SLMs can be extremely powerful for specific tasks or specialized fields, thanks to fine-tuning techniques enabled by quality datasets assembled through π quality data annotation processes.
β
In addition, the performance of small language models for text classification without examples shows that they can rival or even outperform large models in certain tasks.
β
β
What role does data annotation play in the effectiveness of Small Language Models?
β
Data annotation plays an essential role in the efficiency of Small Language Models (SLMs). As SLMs have a smaller architecture than large models, they rely heavily on the quality of the data they are trained on to compensate for their limited size. Accurate, well-structured annotation enables SLMs to learn more efficiently and improve their performance on specific tasks, such as classification tasks.
β
Data annotation also helps to better target learning to particular domains or applications, enabling SLMs to specialize and excel in specific contexts. This reduces the need to process vast quantities of raw data, and maximizes model capabilities with high-quality annotated data. In short, data annotation optimizes the training of SLMs, enabling the construction of very high-quality training datasets to achieve increased accuracy and performance despite their reduced size.
β
Challenges and limits of Small Language Models
β
Small Language Models (SLMs), while innovative and promising, are not without their challenges and limitations. Understanding these aspects is key to evaluating their use and impact in various contexts. We'll tell you more in an upcoming article!
β
Future prospects for Small Language Models
Small Language Models (SLMs) pave the way for many future innovations and applications. Their potential for evolution and integration in various domains is immense, offering promising prospects for artificial intelligence.
β
Conclusion
β
Small Language Models (SLMs) represent a major step forward in the evolution of artificial intelligence, offering lighter, faster and more accessible solutions, while maintaining competitive performance. Thanks to their flexibility and reduced resource requirements, SLMs open up new perspectives for a wide range of applications, from resource-constrained environments to sustainability-conscious industries. As technologies evolve, these models promise to play a central role in the future of AI.