Edge AI: The key to smarter, faster, more efficient models
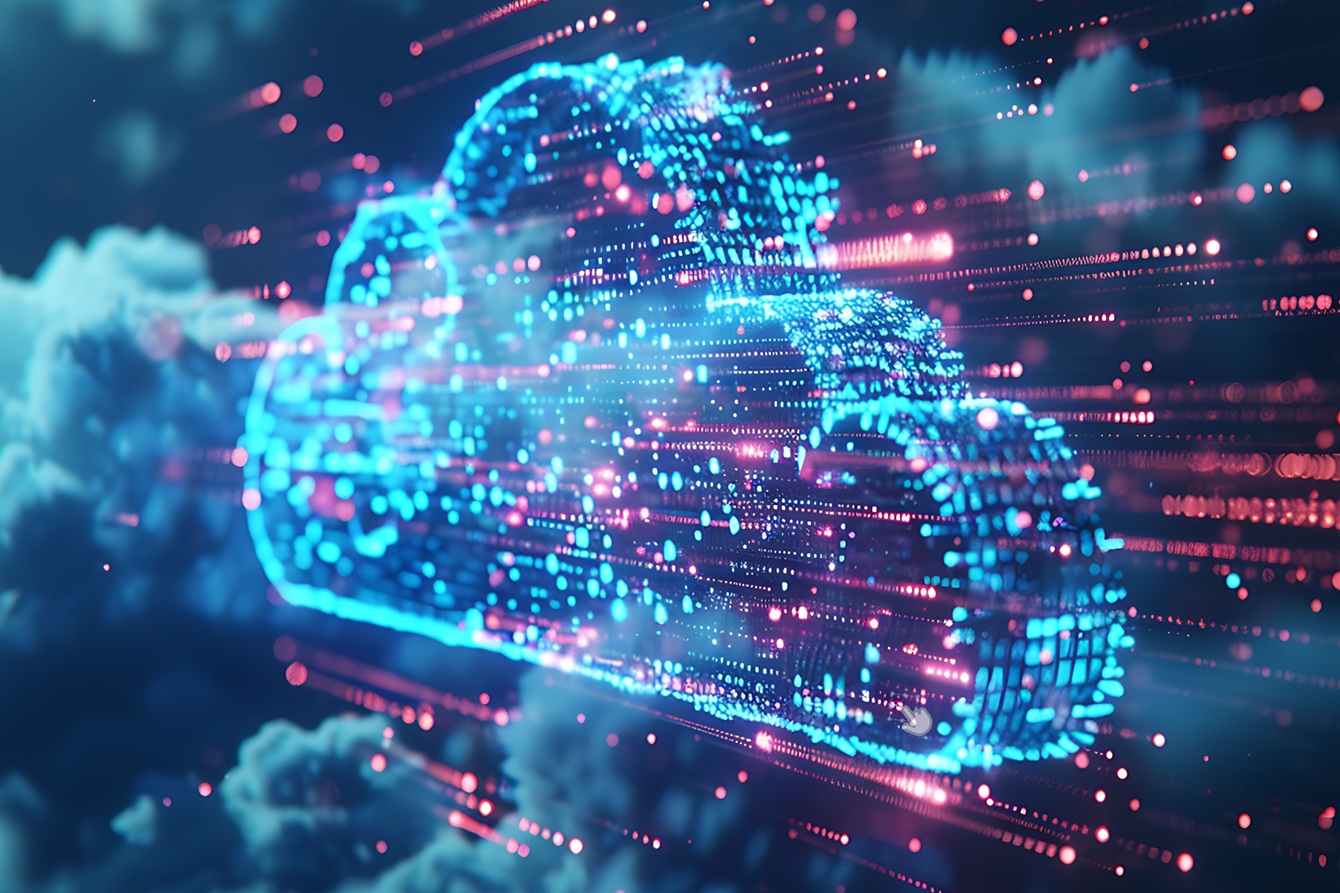
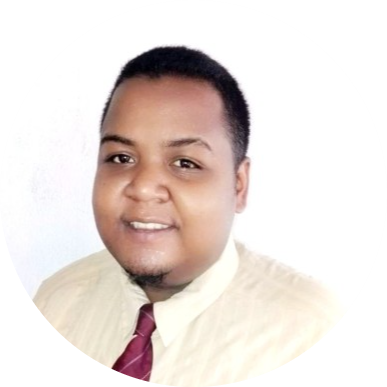
Artificial intelligence (AI) continues to revolutionize many areas of technology, and one of the most promising advances is the emergence of Edge AI. Unlike traditional AI, which relies on centralized data centers to carry out intensive calculations (often datacenters accessible from the cloud), Edge AI moves these operations directly to the devices where the data is generated. It's not so far from local computing after all.
β
This approach offers numerous advantages in terms of latency, security and performance. In a world where data is produced continuously by connected devices such as smartphones, IoT sensors and cameras, Edge AI is emerging as an essential solution for processing these massive flows of information quickly and efficiently.
β
β
What is Edge AI, and how does it differ from traditional AI?
β
Edge AI, or artificial intelligence at the edge, refers to the process of running AI algorithms directly on devices close to where the data is generated, such as sensors, cameras or mobile devices.
β
Unlike traditional AI, which relies on centralized data centers or the cloud to process large quantities of data, Edge AI moves computation to the edge of the network. In short, it enables information to be processed in real time, without the need to send data to a remote server for analysis.
β
Edge AI differs from traditional AI (as seen and consumed in recent years) in several key respects:
- Reduced latency: By performing calculations locally, Edge AI significantly reduces data processing times, which is essential for applications requiring real-time responses, such as π image recognition or π autonomous driving
- Data security: As data does not need to be sent to a remote data center, Edge AI limits the risk of leakage or attack during transfer. This enhances confidentiality, particularly for sensitive sectors such as healthcare or finance.
- Energy efficiency: Edge AI reduces the amount of data that needs to be transferred to remote computing centers, which in turn reduces the energy consumption associated with data transfer and processing.
- Stand-alone operation: Edge AI enables devices to operate even without an Internet connection, making it particularly useful in environments where network connectivity is limited or non-existent.
β
Edge AI thus offers faster, more secure and more resource-efficient solutions, while remaining close to the source of the data, strongly differentiating it from traditional AI in its approach.
β
β
β
β
β
β
β
How does Edge AI improve the efficiency of artificial intelligence models?
β
Edge AI improves the efficiency of artificial intelligence models in several ways. By processing data in real time, it reduces latency and improves model responsiveness. Data no longer needs to be transmitted to a remote server for processing, reducing transmission and processing times. What's more, Edge AI processes data close to the devices, reducing the amount of data to be transmitted and stored, and therefore the associated costs.
β
In addition, Edge AI enables artificial intelligence models to be customized to specific user needs. Real-time data processing allows user preferences and behaviors to be taken into account, enhancing the user experience. Finally, Edge AI reduces dependency on Cloud infrastructures, which can be advantageous for companies looking to cut costs and boost security.
β
β
How does Edge AI improve the efficiency of artificial intelligence models?
β
Edge AI improves the efficiency of artificial intelligence models in several ways, optimizing both data processing and the overall performance of AI systems:
Real-time processing
One of the main advantages of Edge AI is its ability to run AI models directly on the devices where the data is generated, such as sensors or cameras.
β
This enables instantaneous processing of information, with no latency caused by data transfer to a remote server. Models can thus deliver results in real time, improving system responsiveness, as in object recognition applications or anomaly detection.
β
Reduced bandwidth and storage costs
By processing data locally, Edge AI reduces the volume of data to be transferred, and lessens the load on Cloud computing infrastructure (which hosts GPUs / HPUs), thus lightening the load on data centers.
β
Enhanced robustness and resilience
Edge AI-equipped devices can operate autonomously, even in the absence of an Internet connection. This improves system resilience, especially in environments where connectivity is limited or unstable. This autonomous capability also increases the availability of AI models, particularly in critical situations.
β
Optimizing material resources
Edge AI takes advantage of specialized hardware, such as chips designed for artificial intelligence (ASICs, GPUs, FPGAs), which optimize calculations while consuming less energy. This hardware optimization results in more efficient systems, capable of running complex models on low-power devices such as smartphones or IoT devices, without compromising performance.
β
Customization and local adaptation
By performing calculations directly at device level, Edge AI enables models to be better adapted to local conditions. For example, models can be optimized for specific environmental conditions, such as image recognition in low-light environments. This means that models can be optimized for specific contexts, such as particular environmental conditions or demographic characteristics, increasing their accuracy and relevance.
β
Enhanced safety
By keeping data local, Edge AI reduces the risks associated with transmitting sensitive data to remote servers. This approach helps to improve confidentiality and security, which is particularly important for sensitive sectors such as healthcare, transport or finance.
β
β
How does Edge AI influence machine learning?
β
Edge AI has a significant influence on Machine Learning, bringing improvements both in the training phase of models and in their deployment in the field. Edge AI also enables real-time online decision making, improving the efficiency of machine learning models. Here are the main ways in which Edge AI impacts machine learning:
β
Decentralized and federated training
Edge AI enables machine learning to be performed directly on edge devices, avoiding the need to send all the data to a central server. Thanks to π techniques such as federated learningmodels can be trained locally on multiple devices, while combining the results to create a global model.
β
In particular, this preserves data confidentiality while exploiting local resources for training, as in smartphones or IoT devices.
β
Distributed data processing
Rather than processing data in centralized computing centers, Edge AI enables processing to be distributed to several points on the edge of the network. This reduces the need to transmit large amounts of data to the cloud, and enables models to be trained directly where the data is generated.
β
This local processing improves the efficiency of the learning process, particularly in latency-critical environments such as autonomous vehicles or real-time surveillance.
β
Acceleration of pre-trained models
Pre-trained machine learning models can be deployed directly on edge devices to perform specific tasks. Edge AI then improves model inference speed by eliminating the latency associated with transferring data to remote servers.
β
These models, optimized to operate in constrained environments, provide immediate results for applications such as π facial recognition or object detection.
β
Optimizing training resources
Edge AI makes it possible to take advantage of low-power devices to run machine learning algorithms. By optimizing models to run on specialized chips (such as mobile TPUs or GPUs), it becomes possible to perform local learning on resource-constrained devices, while minimizing power consumption.
β
Continuous learning and local updates
Edge AI enables continuous learning or model updates to be performed directly on peripheral devices. This means that models can adapt to new data generated locally, without having to wait for a centralized update.
β
This approach is particularly useful in dynamic environments, such as factories or predictive maintenance systems, where conditions change rapidly and models need to be constantly adjusted.
β
Edge AI use cases and examples
β
Edge AI has a wide range of applications. Here are a few concrete examples:
- Video surveillance: Edge AI can analyze videos in real time to detect anomalies, such as suspicious movements or abandoned objects, enhancing security.
- Autonomous cars: By processing sensor data in real time, Edge AI enables vehicles to make instantaneous decisions, increasing the safety and efficiency of autonomous driving.
- Medical devices: Edge AI can analyze patient data in real time, enabling rapid decision-making to improve patient care and safety.
- Navigation systems: By analyzing navigation data in real time, Edge AI improves the user experience and trip safety.
β
β
Challenges and limits of Edge AI
β
Despite its many advantages, Edge AI also presents challenges and limitations:
- Model complexity: Artificial intelligence models can be complex and difficult to deploy on Edge devices, requiring specific optimizations.
- Amount of data: Processing large amounts of data can be resource-intensive, posing challenges for Edge devices in terms of capacity and performance.
- Security: The security of data and Edge devices is paramount, as data is processed in real time and can be sensitive. Ensuring protection against cyber-attacks is a major challenge.
- Standardization: standardization of protocols and data formats is essential to ensure interoperability between Edge devices and cloud systems, facilitating seamless integration.
β
β
π‘ By addressing these challenges, Edge AI can continue to evolve and offer innovative solutions in a variety of sectors, while maximizing the benefits of decentralized artificial intelligence.
β
β
Conclusion
β
Edge AI represents a major step forward in artificial intelligence, bringing data processing capabilities closer to their source. This approach not only reduces latency and improves the performance of AI models, but also enhances data security while optimizing energy efficiency.
β
By facilitating real-time inference and enabling decentralized learning, Edge AI opens up new opportunities in sectors as varied as industry, healthcare and smart infrastructure.
β
As the demand for faster, smarter systems continues to grow, Edge AI is emerging as a key solution to tomorrow's technological challenges.