Direct preference optimization (DPO): towards more intelligent AI

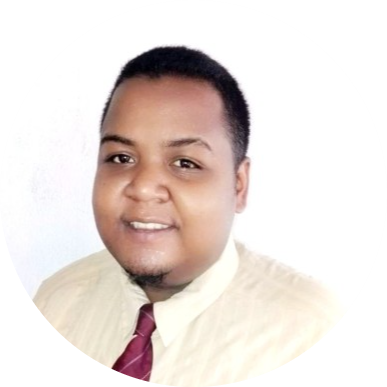
Beyond the new AI products coming to market at a breakneck pace, artificial intelligence and research in this field continue to evolve at an impressive rate, thanks in particular to innovative optimization methods. These include 🔗 Direct Preference Optimization (DPO)stands out as a promising approach.
Unlike traditional learning methods, which rely primarily on maximizing a reward function, DPO seeks to align the decisions of language models (LLMs) with explicit human preferences. Typically, traditional methods often require a complex reward model, which can make the optimization process longer and more complicated.
This technique looks promising for the development of more intelligent AI systems tailored to users' needs.
What is Direct Preference Optimization (DPO)?
Direct Preference Optimization (DPO) is an optimization method applied in the field of artificial intelligence, which aims to directly adjust models according to human preferences. Unlike conventional approaches, which rely on explicit or implicit reward signals, DPO relies on human judgments to guide model behavior.
Visit RLHF (reinforcement learning from human feedback) is a commonly used method for aligning AI models with human preferences, but it requires a complex reward model. In other words, instead of maximizing a pre-defined reward function, RLHF seeks to align model decisions with the preferences expressed by users. This makes it possible to create AI systems that are more intuitive and more in line with human expectations, particularly in contexts where preferences are not always easily quantifiable.
This method is particularly useful in scenarios where standard performance criteria are difficult to define, or where it is important to prioritize the user experience, such as in text generation, content recommendation, or interface personalization. OPD therefore stands out for its ability to bring AI models closer to users' subjective expectations, offering better adaptation to specific preferences.
How does DPO differ from other optimization methods, mainly reinforcement learning?
Direct Preference Optimization (DPO) differs primarily from Reinforcement Learning (RL) in the way preferences and rewards are used to tune AI models. Reinforcement learning (RL) presents challenges, including the difficulty of obtaining annotated datasets and the need for complex reward models.
Using rewards
In reinforcement learning, an agent interacts with an environment by taking actions and receiving rewards in return. These rewards, whether positive or negative, guide the agent to learn how to maximize long-term gain.
AR therefore relies on a predefined reward model, which needs to be well understood and defined to achieve optimal results. However, in some situations, human preferences are not easily quantifiable in terms of explicit rewards, which limits the flexibility of AR.
OPD, on the other hand, gets around this limitation by relying directly on human preferences. Rather than trying to define an objective reward function, OPD takes into account explicit human judgments between different options or outcomes. Users directly compare several model outputs, and their preferences guide model optimization without having to go through an intermediate step of quantified reward.
Complexity of human preferences
While reinforcement learning can work well in environments where rewards are easy to formalize (for example, in games or robotic tasks), it becomes more complex in contexts where preferences are subjective or difficult to model.
DPO, on the other hand, is designed to better capture these subtle, unquantifiable preferences, making it better suited to tasks such as personalization, recommendation, or content generation, where expectations vary considerably from one user to another.
Optimization approach
Reinforcement learning seeks to optimize the agent's actions through a process of trial and error, maximizing a long-term reward function. Fine-tuning of language models is necessary to ensure that model results match human preferences. OPD takes a more direct approach, aligning the model with human preferences through pairwise comparisons or rankings, without going through a step of simulating interaction with the environment.
Human preferences in AI
Human preferences play a key role in the development of artificial intelligence (AI). Indeed, for AI systems to be truly effective, they must be able to understand and respond to users' needs and expectations. This is where Direct Preference Optimization (DPO) comes in, by aligning the decisions of AI models with explicit human preferences.
The DPO approach is distinguished by its ability to integrate human judgments directly into the optimization process. Unlike traditional methods based on often abstract reward functions, DPO uses human preferences to guide model learning. This makes it possible to create AI systems that are more intuitive and more in line with user expectations, particularly in contexts where preferences are not easily quantifiable.
By incorporating human preferences, OPD enables the development of AI models that are not only more accurate, but also more tailored to users' specific needs. This approach is particularly useful in areas such as service personalization, content recommendation and text generation, where expectations vary considerably from one user to another.
What are the advantages of DPO for training AI models?
Direct Preference Optimization (DPO) offers several notable advantages for training artificial intelligence models, not least in terms of aligning models with finer, more nuanced human preferences. Here are its main benefits:
Direct alignment with human preferences
Unlike traditional methods, which depend on reward functions that are often difficult to define or unsuited to subjective criteria, OPD enables user preferences to be captured directly. The fine-tuning of hyperparameters and 🔗 tagged data is essential to ensure that model results match human preferences. By incorporating these preferences into the training process, the model becomes more capable of meeting real user expectations.
Better management of subjective preferences
In areas where performance criteria cannot be easily quantified (such as user satisfaction, content generation or product recommendation), OPD makes it possible to better manage these subjective preferences, which are often overlooked in conventional approaches. This enables AI models to make more nuanced decisions, in line with individual user needs.
Reducing bias induced by performance metrics
Reward functions or performance metrics can introduce unwanted biases into the training of language models (LLMs). OPD, by allowing users to provide direct judgments, helps to limit these biases by moving away from optimization based solely on numbers and incorporating more flexible subjective criteria.
Improving the quality of decisions
OPD enables AI models to make decisions that are better aligned with human preferences in complex or ambiguous situations. This is particularly useful in applications such as text generation, content recommendation and service personalization, where user experience is paramount.
Adaptation to changing scenarios
Human preferences can evolve over time, and rigid reward functions don't always capture these changes. DPO makes it possible to adapt models more fluidly by constantly reassessing human preferences through new data or continuous feedback.
Use in non-stationary environments
In environments where conditions change rapidly (e.g., recommendation platforms or virtual assistants), OPD enables greater flexibility by adjusting AI models based on direct user feedback, without the need to constantly redefine reward functions.
DPO methodology and applications
The OPD methodology is based on the collection and use of human preference data to optimize the parameters of AI systems. In concrete terms, this involves collecting explicit judgments from users on different model outputs, and using these judgments to adjust models to better meet human expectations.
This approach can be applied to a multitude of fields. For example, in the healthcare sector, OPD can improve AI systems responsible for diagnosing diseases or suggesting personalized treatments. In finance, it can optimize AI systems involved in investment decision-making, taking into account the specific preferences of investors.
OPD is also at the heart of numerous academic research projects. At Stanford University, researchers such as Stefano Ermon, Archit Sharma and Chelsea Finn are exploring the potential of this approach to improve the accuracy and efficiency of AI systems. Their work shows that OPD can revolutionize the way AI models are trained.
In short, OPD is an innovative approach that uses human preferences to optimize the performance of AI systems. Its applications are vast and varied, ranging from healthcare and finance to technology and academic research. Thanks to DPO, AI models can become smarter, more intuitive and better adapted to users' needs.
How important is data annotation in the DPO?
Data annotation is essential in OPD, as it enables human preferences to be captured directly in small or massive datasets. By providing explicit judgments on model outputs, annotation helps to tailor results to users' expectations.
It also improves the quality of training data, reduces the biases associated with traditional methods (on the assumption that annotators working on the dataset have been rigorously selected), and enables continuous adaptation of models to evolving preferences. In short, data annotation ensures that AI models remain aligned with real user needs!
In conclusion
Direct preference optimization (DPO) could represent a major advance in training artificial intelligence models, enabling more precise alignment with human preferences. By incorporating explicit judgments and focusing on users' subjective needs, this method promises AI systems that are more powerful, intuitive and adapted to complex contexts.
In this context, data annotation plays a central role, ensuring that models remain in tune with changing user expectations. As AI applications multiply, DPO is emerging as a key approach to creating truly intelligent models!