Data quality in Artificial Intelligence: an information-theoretic approach
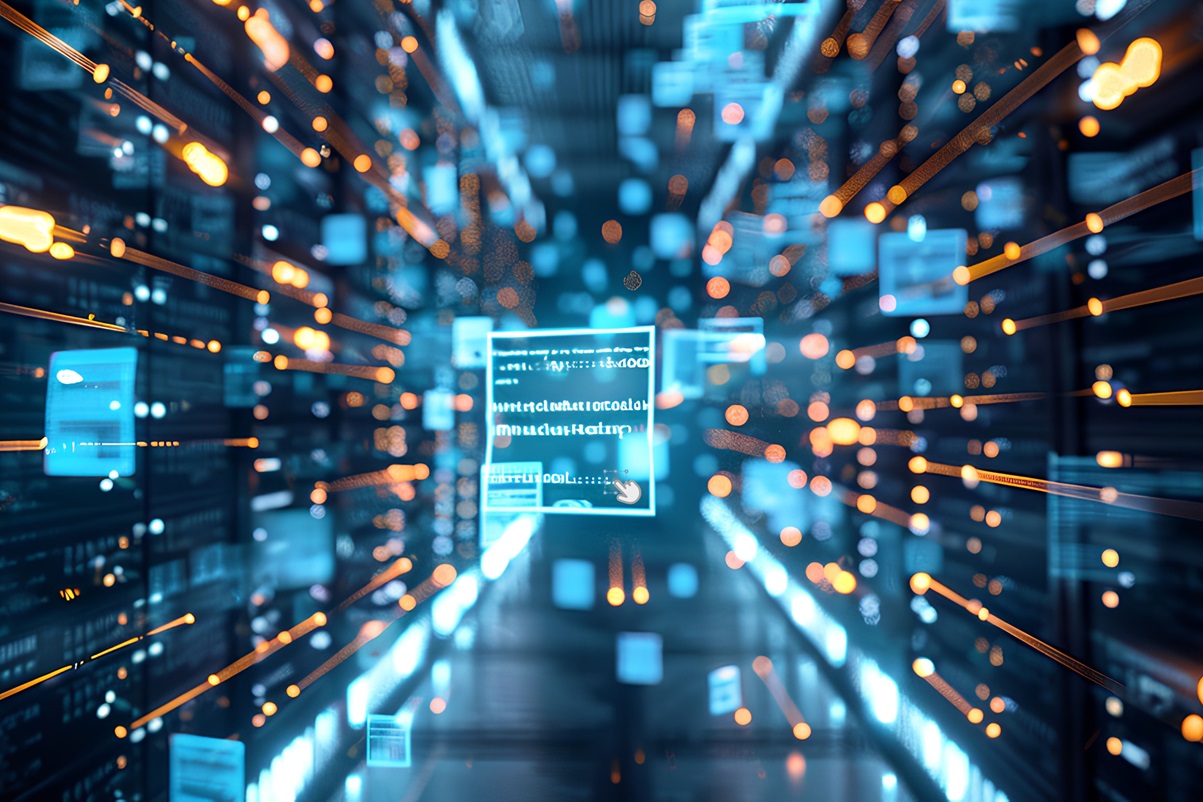
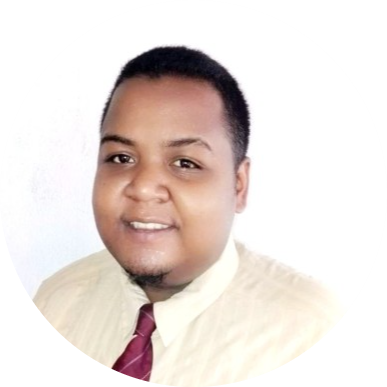
The expression "Garbage In, Garbage Out" is often quoted in Artificial Intelligence (AI), but few understand its theoretical underpinnings.
β
The race for performance in artificial intelligence often focuses onmodel architecture, computing power or optimization techniques.
β
Yet one crucial aspect remains underestimated: the quality of training data. Imagine building a house on an unstable foundation: no matter how sophisticated the architecture, the structure will be compromised.
β
Similarly, an AI model trained on noisy or mislabeled data will inevitably reproduce these defects. This reality is not just empirical; it follows directly from the fundamental principles of information theory. Understanding these principles helps us to understand why investment in data quality is often more important than investment in model complexity.
β
β
Theoretical foundations
β
Shannon's Entropy: the measure of information
Claude Shannon revolutionized our understanding of information by proposing a quantitative measure.Shannon's entropy is given by
β
H = -β p(x) logβ(p(x))
β
β
Where:
- H is entropy (measured in bits)
- p(x) is the probability of occurrence of an event x
- β represents the sum over all possible events
β
This formula tells us something fundamental: information is linked to unpredictability. A certain event (p=1) brings no new information, while a rare event brings a lot of information.
β
β
Application to training data
In a training dataset, the total information can be broken down as follows:
β
H_total = H_usable + H_noise
β
Where:
- H_useful represents information relevant to our task
- H_noise represents imperfections, errors and artifacts
β
This decomposition has a crucial consequence: since an AI model cannot intrinsically distinguish useful information from noise, it will learn both.
This runs the risk of reproducing the model's noise output.
β
β
The principle of information retention
β
The fundamental limit
A fundamental theorem of information theory states that a system cannot create information; it can only transform it. For an AI model, this means:
β
Output_quality β€ Input_quality
β
This inequality is strict: no architecture, no matter how sophisticated, can exceed this limit.
β
β
Case study: image upscaling
β
Let's take the example of photo upscaling, where we want to increase the resolution of an image:
β

The quality chain
For a high-resolution (HR) image generated from a low-resolution (LR) image :
β
PSNR_output β€ PSNR_input - 10*logββ(factor_upscalingΒ²)
β
Where:
- PSNR (Peak Signal-to-Noise Ratio) measures image quality
- upscaling_factor is the ratio between resolutions (e.g. 2 for doubling)
β
Impact of training data
β
Let's consider two training scenarios:
1. High Quality Dataset
- HR images: Uncompressed 4K photos
- Average PSNR: 45dB
- Possible result: ~35dB after upscaling x2
β
β
2. Dataset Poor
- HR images: JPEG-compressed photos
- Average PSNR: 30dB
- Maximum result: ~20dB after upscaling x2
The 15dB difference in the final result is directly linked to the quality of the training data.
β
PSNR in dB is a logarithmic measure that compares the maximum possible signal with the noise (the error).
The higher the number of dB, the better the quality:
β
PSNR (Peak Signal-to-Noise Ratio) is defined as :
β
PSNR = 10 * logββ(MAXΒ²/MSE)
β
Where:
- MAX is the maximum possible pixel value (255 for 8 bits)
- MSE is mean square error
β
For upscaling, when the resolution is increased by a factor of n, MSE tends to increase, which effectively reduces PSNR.
The quality of the result is therefore very sensitive to the level of noise.
β
β
Order of magnitude of PSNR in dB for images
- High-quality JPEG image: ~40-45dB
- Average JPEG compression: ~30-35dB
- A highly compressed image: ~20-25dB
β
dB is a logarithmic scale:
- +3dB = 2x better quality
- +10dB = 10x better quality
- +20dB = 100x better quality
β
So when we say "~35dB after upscaling x2", it means that :
- The resulting image has good quality
- Differences from the "perfect" image are hard to see
- Typical of a good upscaling algorithm
β
β
The cascade effect: the danger of AI-generated data
β
When AI-generated images are used to train other models, degradation follows a geometric progression:
β
Generation_quality_n = Original_quality * (1 - Ο)βΏ
β
Where:
- Ο is the degradation rate per generation
- n is the number of generations
β
This formula explains why using AI-generated images to train other models leads to rapid quality degradation.
β
β
The importance of labelling
β
The quality of the labels is as crucial as that of the data itself. For a supervised model :
β
Maximum_precision = min(Data_Quality, Precision_labels)
β
β
This simple formula shows that even with perfect data, imprecise labels strictly limit possible performance.
β
β
Practical recommendations
β
1. Dataset preparation
Above, our simplistic demonstration illustrates the crucial importance of the quality of the data used for training. We invite you to consult this article to learn more about how to prepare a quality dataset for your artificial intelligence models.
We can't go into detail here, but the informed reader will notice that the definition of "noise" raises philosophical questions. How do you define noise?
β
2. Reflection: the subjective nature of noise
The very definition of "noise" in data raises profound philosophical questions. What is considered noise for one application may be crucial information for another.
β
Let's take the example of a photo:
- For a facial recognition model, lighting variations are "noise".
- For a lighting analysis model, these same variations are the main information.
β
This subjectivity of noise reminds us that data "quality" is intrinsically linked to our objective. Like SchrΓΆdinger's cat, noise exists in a superposition: it is both information and disturbance, until we define our observation context.
β
This duality underlines the importance of a clear, contextual definition of "quality" in our AI projects, challenging the idea of absolute data quality.
β
3. Quality metrics
For each data type, define minimum thresholds, e.g. :
β
Images
β
PSNR > 40dB, SSIM >0.95
β
Labels
β
Accuracy > 98
β
Coherence
β
Crossover tests > 95% of results
β
The 40dB threshold is not arbitrary. In practice :
- 40dB: Virtually imperceptible differences
- 35-40dB: Very good quality, differences only visible to experts
- 30-35dB: Acceptable quality for general use
- <30dB : DΓ©gradation visible
β
SSIM (Structural Similarity Index)
The SSIM complements the PSNR :
β
seuils_SSIM = { Β Β "Excellent": ">0.95", Β Β "Bon": "0.90-0.95", Β Β "Acceptable": "0.85-0.90", Β Β "ProblΓ©matique": "<0.85"}
β
SSIM is closer to human perception, as it considers the structure of the image.
β
Consistency tests
Cross-tests >95% involve :
- k-fold cross-validation
- Internal consistency tests
- Checking outliers
- Distribution analysis
β
Conclusion
β
Information theory provides us with a rigorous framework demonstrating that data quality is not an option , but a strict mathematical limit. An AI model, no matter how sophisticated, cannot exceed the quality of its training data.
β
This understanding must guide our investments: rather than just looking for more complex architectures, our priority must be to ensure the quality of our training data !
β
β
β
Sources
Shannon entropy : https://fr.wikipedia.org/wiki/Entropie_de_Shannon
Illustration: https://replicate.com/philz1337x/clarity-upscaler
β
Academic and technical sources
- Shannon, C.E. (1948). "A Mathematical Theory of Communication". Bell System Technical Journal.
- Wang, Z. et al. (2004). "Image Quality Assessment: From Error Visibility to Structural Similarity". IEEE Transactions on Image Processing.
- Goodfellow, I., Bengio, Y., & Courville, A. (2016). "Deep Learning". MIT Press.
- Zhang, K. et al. (2020). "Deep Learning for Image Super-Resolution: A Survey". IEEE Transactions on Pattern Analysis and Machine Intelligence.
- Dodge, S., & Karam, L. (2016). "Understanding how image quality affects deep neural networks". International Conference on Quality of Multimedia Experience (QoMEX).