Understanding the importance of Data Curation for AI models

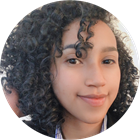
Data Curation today occupies a central place in the development of artificial intelligence (AI) models, and in data preparation pipelines for AI in particular. Expanded access to data poses management and control challenges, requiring curation solutions to ensure the accuracy and correct use of data by business users. Indeed, the quality of the data used to train these models directly influences their performance and reliability!
β
Data Curation goes far beyond simple data cleansing: it includes the selection, organization and annotation of π datasetsto ensure that models can learn efficiently and accurately. When it comes to managing complex datasets, it's important to address the challenges associated with data governance and ensure a proper framework for curation operations. With growing volumes of often imperfect data, curation becomes essential to avoid bias, improve data representativeness and guarantee the robustness of AI systems.
β
β
π‘ At a time when automated decisions and algorithms are influencing many sectors, careful data curation is essential to unlock the full potential of machine learning models. That's what this article is all about: without going into too much technical detail, we explain what Data Curation is in practice!
β
β
What is Data Curation and why is it essential in AI?
β
Data Curation is the process of managing and optimizing data sets throughout their lifecycle, with the aim of guaranteeing their quality, relevance and usefulness for a specific purpose. It is indeed necessary to gather and share information within a company to establish curation policies tailored to the needs of its members, in line with the organization's data governance.
β
This process includes several key stages such as data collection, organization, documentation, annotation, cleansing and enrichment. A coordinated service is needed to harmonize data curation and management activities, including digital libraries and archives, to guarantee data access and preservation.
β
Unlike simple cleansing, Data Curation aims to structure data so that it can be effectively used to train artificial intelligence (AI) models.
β
Data curation is essential in AI for several reasons:
β
Improving data quality
An AI model can only be as good as the data it is trained on. Curation meets user demand for high-quality data. Rigorous curation ensures that data is free of errors, duplicates or biases, resulting in more reliable and accurate models.
β
Bias reduction
Unsorted or poorly annotated data can introduce biases into AI models, leading to discriminating or incorrect results. Curation helps to detect and correct these potential biases, ensuring that data is representative and balanced.
β
Easier integration of multiple data sets
Curation helps to merge data from different sources, making them compatible and usable in the same project. It also plays an important role in aggregating links from different sources to create an enriching user experience. This enables AI models to take advantage of a greater diversity of data to generate more robust results.
β
Optimizing model performance
Well-organized and annotated data enables machine learning algorithms to train more efficiently. This improves model performance, reducing training time and increasing prediction accuracy.
β
β
The challenges of data management
β
Data management is a complex process that requires careful attention to ensure the quality and reliability of information. The challenges of data management can be many, but here are some of the most common:
β
Complexity of data sources
Data sources can be highly varied and complex, making data management and curation difficult. Data can come from internal sources, such as company databases, or external sources, such as social networks or websites. The complexity of data sources can make it difficult to collect, select and prepare data for analysis.
β
Volume and variety of data
The volume and variety of data can also present a challenge for data management. Companies can generate massive amounts of data every day, which can make it difficult to manage and curate. What's more, data can be in a variety of formats, such as images, videos or text documents.
β
β
How is data curation different from data cleansing?
β
Data curation and data cleansing are often confused, but they differ in scope and purpose.
β
Process scope
Data cleansing is a subset of curation. It mainly involves eliminating errors, duplicates, missing or inconsistent values from a dataset. The aim is to make the data cleaner and ready for use without false information that could compromise the performance of AI models.
β
Data curation, on the other hand, encompasses the entire data management process. It includes not only cleansing, but also broader steps such as collecting, organizing, annotating, and sometimes even creating additional data (e.g., via data augmentation) or correcting biases. Curation also includes the π selecting and organizing content to improve visibility and SEO. It aims to optimize the entire data lifecycle, ensuring that data is not only clean, but also relevant, complete, well-documented, and correctly structured for its end use.
β
Objectives
The main aim of data cleansing is to guarantee data integrity and quality by removing anomalies or errors.
β
Data Curation, in addition to guaranteeing data quality, seeks to maximize its value by making it exploitable in a specific context (such as training an AI model). It ensures that data is well contextualized, documented, and can be used efficiently and reproducibly.
β
Enrichment process
Cleansing does not usually deal with data enrichment. Conversely, curation can include enrichment, for example by adding annotations or metadata, making the data more informative and useful for specific algorithms.
β
Bias management and information diversity
Cleaning focuses on correcting immediate errors, but does not necessarily take into account more complex issues such as data diversity or bias.
β
Data Curation pays particular attention to these aspects, ensuring that data is balanced, representative and unbiased. This is essential to guarantee fair and ethical results in AI models.
β
β
Creating and curating datasets: what's the difference?
β
Dataset creation and curation are two distinct but complementary processes that play a key role in training artificial intelligence (AI) models. Together, they ensure that the data used is not only available, but also high-quality, well-organized and relevant to model training. Here's how these two processes complement each other:
β
Dataset creation
The creation of datasets involves gathering raw data from a variety of sources. It is necessary to contextualize and unify information around a subject to create added value and facilitate web users' access to relevant content. This can include images, text, audio or video recordings, or structured data.
β
This process aims to provide sufficient data to train AI models, and is often the first step in the data pipeline. It can be carried out manually or using automated techniques, such as web scraping or data collection via sensors.
β
Dataset curation
Once the data has been collected, curation steps in to ensure that it is ready for use by AI models. This includes cleaning, annotating, structuring and enriching the data.
β
Curation is essential to ensure that data is of high quality, free from errors, and representative of the model's use cases. This process also helps to improve data diversity and correct potential biases, which is essential to ensure reliable and accurate results.
β
Why do dataset creation and curation complement each other?
β
Data qualityβ
Creation enables large quantities of data to be generated or collected. Curation, on the other hand, ensures that this data is usable by cleaning up errors and improving overall quality, enabling AI models to learn more effectively.
β
Annotation and enrichmentβ
The creation of datasets provides raw data, but this data often needs to be annotated to be exploitable. For example, in an image recognition project, it's not enough to have photos; you also need to π annotate to indicate what each image contains (e.g. "dog", "car", "pedestrian"). This is where curation comes in, adding annotations and metadata that make it easier to learn the model.
β
Eliminating bias and improving diversityβ
The creation of datasets can introduce biases due to the nature of the data collected (for example, cultural or geographical biases). Curation helps to detect and correct these biases by rebalancing the data and ensuring that it is representative of reality. This is crucial to prevent AI models from reproducing pre-existing biases.
β
Learning optimizationβ
The datasets created are not always optimized for training AI models, due to format or structure problems. Curation restructures and formats data so that it can be efficiently processed by algorithms, reducing processing time and improving prediction accuracy.
β
β
Conclusion
β
In conclusion, data curation is a central and indispensable element in the development of artificial intelligence models. In addition to the creation of datasets, this practice transforms raw data sets into quality resources, ready to be exploited by learning algorithms.
β
By guaranteeing the cleanliness, relevance, annotation and balance of data, curation not only helps improve model skills, but also minimizes bias and ensures the reliability of results. In a context where data is increasingly voluminous and varied, curation is becoming a strategic asset for any organization seeking to make the most of AI.
β
It plays a key role not only in optimizing model performance, but also in creating ethical and robust AI solutions. So, combining dataset creation and curation is essential for your future AI developments!
β