All you need to know about audio annotation for AI (or how to annotate audio files for AI)

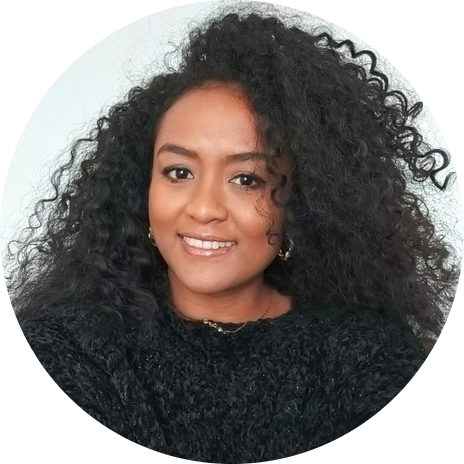
In the process of creating today's AI models and tools, the use of audio annotation is significant. Just as each individual tries to improve and is able to answer questions more naturally and accurately with practice and experience, an AI model develops this ability with the right training, which is often based on a complex process of preparing audio data for the AI. In everyday life, we ask today's AI models a variety of questions in the form of voice commands. In the case of Siri or Alexafor example:"Hey Siri, can you find an address for a Vietnamese restaurant? I'm hungry". Audio annotation helps the AI transcription tool understand our voice and interpret our questions.
β
This article will help you understand the full details of the audio annotation processΒ and audio annotation tools used by Data Scientists to prepare the training data used by Siri or Alexa, and many other applications. Let's read on and find out how it works!
β
β

β
β
β
How do I define audio annotation?
β
Before going any further, let's try to understand and defineaudio annotation with a slightly clearer concept! Audio annotation is the process of adding notes ortags to audio recordings. Annotating audio files is like putting stickers on different parts of a recording to say what it is, like"This part is a barking dog" or"This is a car horn". This helps computers understand and recognize different sounds more easily.
β
Audio annotation is a milestone in the field of machine learning and artificial intelligence. As these technologies continue to advance, the need for accurate and comprehensive audio annotation becomes more important.
β
Why do we need audio annotation?
β
Audio annotation is essential fortraining computers to understand sound as humans do. Imagine teaching a child to recognize animal sounds; we have to repeat and associate each sound with a picture, for example, with illustrated books and simple rules. Audio annotation does this for computers.
β
β

β
β
With over 500 hours of video uploaded every minute on platforms like YouTube, there's a huge amount of audio for computers to analyze. Without audio annotation, computers wouldn't know whether a sound in a video was a doorbell ringing or a phone notification. This is the basis of services like voice-activated GPS, which helps us navigate by recognizing our voice commands, which over 77% of smartphone users have tried out. Also, for the hearing impaired, audio annotation is essential for creating reliable software that translates spoken words into text in real time, making content more accessible. Audio annotation is the answer to today's accessibility challenges!
β
β
β
β
β
β
β
β
What are the different types of audio annotation?
β
Audio annotation is a powerful tool available in many different forms. Here are some of the most famous ones you should know about!
β
Sound event detection
Sound event detection involves marking specific audio events in a recording. This can range from identifying the sound of glass breaking, to the melody of a bird singing. The audio data annotators listen carefully to isolate these events and mark them so that the machines can learn what each event sounds like.
β
Transcription of speech into text
This involves converting spoken words or recorded speech into written text. Transcribing speech into text is essential for creating subtitles or transcribing meetings. Speech recognition software relies heavily on large datasets of transcribed speech to correctly understand different accents and dialects, in all languages.
β
Emotion recognition
Here, annotators label parts of an audio recording by the emotion conveyed. Is the speaker happy, sad or angry? This is increasingly used in customer service to assess callers' emotions, and in mental health applications to monitor users' well-being.
β
Diarization
Diarization is the labeling process used to identify who is speaking in an audio sequence, when several speakers are present in an audio recording. This helps to transcribe interviews or court proceedings by attributing the text to the correct speaker in the given recording.
β
Classification of environmental sounds (or CSE)
Environmental Sound Classification (ESC) is a process in which annotators create and label audio extracts of unspoken, non-musical sounds from our environment. Whether it's the hustle and bustle of city traffic, the peaceful chirping of birds in a forest, or the subtle sound of water running in a stream, annotators categorize these environmental sounds to help AI systems recognize and respond to them.
β
CSE is particularly useful in applications for smart cities, security systems and environmental monitoring, where differentiating (and sometimes ignoring) a multitude of background noises is critical.
β
Classification of natural language utterances (NLU) in audio classification
Natural Language Utterance (NLU) classification in audio annotation goes a step further by recognizing not only words, but also understanding the intention behind them. This involves analyzing sentences in audio and categorizing them by speaker intent, such as a command, a question or a request.
β
A common example of NLU can be seen in voice-activated virtual assistants, which interpret and respond to user queries. This powerful aspect of audio classification enables AI to process and interact using a human-like understanding of natural language, transforming voice interfaces into intelligent conversational agents. With NLU, we're moving closer to a world where communication between man and machine becomes fluid and intuitive, and dispenses with complex interfaces.
β
β
How to create the perfect audio annotation for AI?
β
Creating a reliable audio annotation is no easy task. But it can be done with expert help. Here are a few best practices for annotating high-quality audio data that can be used by your models.
β
Choosing the right tools
Selecting the right software and hardware is paramount to quality audio annotation. From a software point of view, you'll needaudio editing software that allows you to label audio accurately. As for your annotators, you'll need to equip them with quality headphones to enable them to capture and interpret every nuance of the sound.
β
β
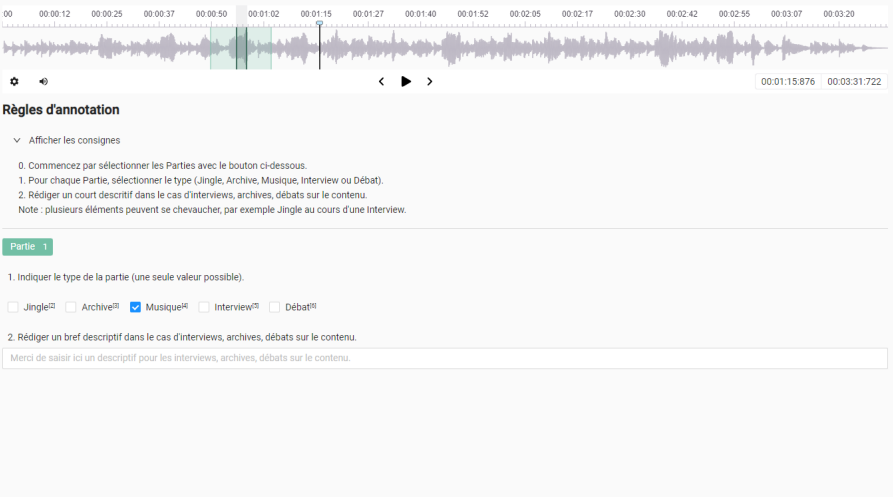
β
β
Create a detailed annotation guide
Having a clear and comprehensive guide (defining the principles for creating your audio metadata) also helps to ensure consistency throughout the annotation process. This document should define all audio categories and the criteria for each.
β
Employ trained and experienced annotators
Make sure your annotators are properly trained. They need to understand the annotation guide and be able to recognize and categorize different sounds accurately.
β
Carry out quality controls
Regular quality assessments are necessary. Listen to a random selection of annotated audio files and check that the sounds have been labeled according to the guidelines.
β
Working in an iterative process
Audio annotation is an iterative process. Gather feedback, refine your guidelines and re-train annotators as necessary to improve the quality of project audio annotation over time.
β
Use diversified data
To train a model that works well in different scenarios, use a diverse data set from different environments, dialects and audio recording qualities.
β
β
How to use an audio annotation tool effectively?
β
To use an audio annotation tool effectively:
- Start with a clear goal: Define what you want your AI system to do with the entire audio file. Whether it's recognizing specific sounds or understanding speech, your goal will guide the annotation process.
- Choose an annotation platform with an intuitive interface : Choose annotation tools that are easy to use and learn, so that annotators can concentrate on the content. They don't have to waste their time struggling with the interface!
- Invest in quality equipment: Use high-fidelity headphones and microphones to ensure that every audio nuance is accurately captured and annotated.
- Provide training and resources: Offer tutorials and examples to annotators so they understand how to use the system and what is expected in the annotation process.
- Regularly check accuracy: periodically review annotated audio to ensure that labels are being applied correctly, and make adjustments if necessary.
- Iterate for improvement: Continuously improve the system by re-training annotators with updated guidelines based on feedback from accuracy checks.
- Diversify your data sets: Use audio samples from different sources to make your AI robust and accurate in different situations.
- Stay up-to-date: Keep abreast of the latest developments in annotation tools and techniques to continually improve the efficiency of your system.
β
β
Main applications and use cases for audio annotation in today's world
β
Examples of audio annotation are very common, and we find them in our everyday lives. Let's take a look at some of the most common applications or cases of these annotations, in different fields!
β
Voice assistants and smart homes
Voice-enabled virtual assistants, such as Amazon Alexa, Google Assistant and Apple Siri, are prime examples of audio annotation applications. These AI-powered voice recognition tools recognize and process human speech, enabling users to operate smart home devices, search the Internet and manage personal calendars through voice commands.
β
Health monitoring
In the healthcare sector, audio annotation is used to develop systems capable of monitoring patients with conditions such as sleep apnea and asthma. These AI systems are trained to listen for wheezing, coughing and other abnormal sounds that signal distress, often enabling preventive healthcare interventions.
β
Automotive industry
Modern vehicles are increasingly equipped with voice-activated controls and safety features that depend on audio annotation. Annotators classify sounds inside and outside the car to improve driver assistance systems. This audio data helps develop features such as emergency braking systems that can instantly detect the sound of other cars or pedestrians.
β
Security and surveillance
Audio annotation enhances security systems by enabling them to detect specific sounds, such as glass breaking, alarms or unauthorized entry. By 2025, the global video surveillance market is expected to reach $75.6 billion, with audio surveillance accounting for a significant share.
β
Wildlife conservation
Conservationists use audio annotation tools to monitor animal populations. By training AI to identify and classify animal calls, researchers can track the presence and movements of species in a particular area, which is essential for species conservation efforts.
β
Language translation services
Language translation services improve real-time communication between speakers of different languages. Audio annotation improves the accuracy of machine translation, making international business and travel smoother. The market for AI translation services is expected to grow, with projected sales of $1.5 billion by 2024.
β
β
What are some common challenges with audio annotation and how can they be overcome?
β
When it comes to difficulties with audio annotations, here are some common challenges and their solutions:
β
Interference from ambient noise
One of the biggest challenges in audio annotation is differentiating desired audio signals from background noise. This interference can lead to inaccurate annotations if the AI system has difficulty isolating the target sound.
β
Solution : Use noise reduction algorithms and high-quality recordings to reduce the effect of ambient noise. In addition, the training data should include samples with different levels of background noise so that the AI learns to recognize the target sound in different settings.
β
Speaker variability
Humans have diverse voice tones, accents and speech rates, creating variability in speech recognition that can confuse AI systems.
β
Solution : To overcome speaker variability, collect and annotate audio samples from a wide range of speakers with different characteristics. This variety helps AI systems to become more adaptable and accurate in real-life scenarios.
β
Inconsistent annotations
Inconsistency in audio labeling can also occur when multiple annotators interpret audio differently, which can lead to a less efficient AI model.
β
Solution : Establish clear guidelines and provide thorough training to ensure that all annotators apply labels consistently. Regular accuracy checks and feedback are also important to maintain consistent annotations.
β
Lack of high-quality data
High-quality, diverse data sets are essential for training effective audio recognition systems, but obtaining such data can be time-consuming and often difficult.
β
Solution : Form partnerships with organizations that can provide or help collect diverse audio samples. Use synthetic data generation techniques if real-world data is scarce, taking care to represent a variety of scenarios.
β
Data security and confidentiality
Audio datasets can contain sensitive information, presenting potential privacy concerns and requiring secure handling.
β
Solution : Implement strict data security protocols and, where possible, ensure that all personally identifiable information is anonymized before annotation begins. Transparency about data handling can also promote trust and compliance.
β
β
In brief
β
An efficient audio annotation process is key to advancing AI and ML technologies. As you work with AI, overcoming the challenges associated with annotation tasks is necessary to build robust AI systems. By adopting clear strategies and technologies, we improve AI's ability to understand and process audio data. As AI continues to evolve, so will approaches to audio annotation, always with the aim of improving accuracy and reliability in AI sound and speech recognition models.
β