Data labeling: a call for ethical regulation of AI in Europe
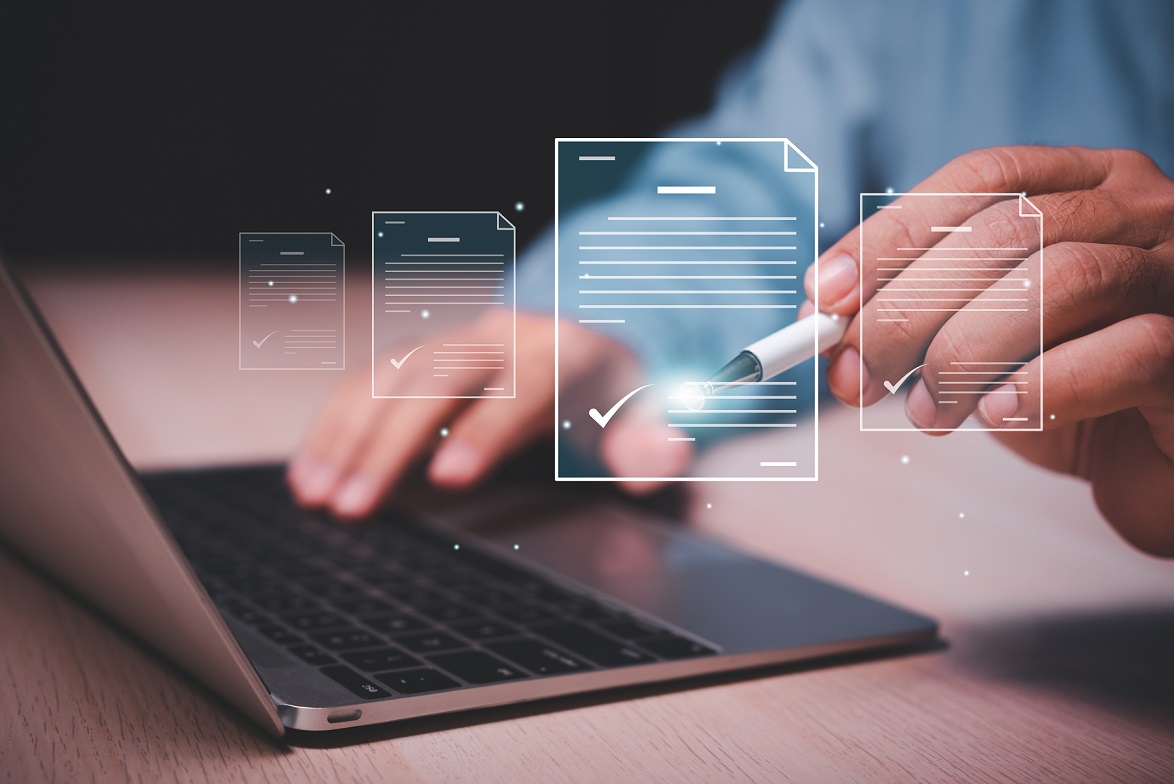
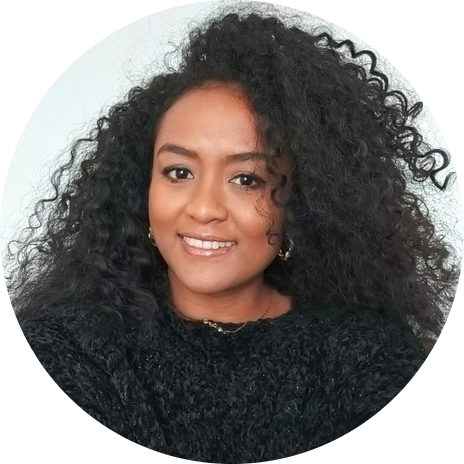
π‘ Artificial intelligence (AI) is revolutionizing our world, but to ensure its ethical and responsible use, proper regulation is essential.
β
β
In this article, we wanted to address the importance of the data labeling process in the construction of AI products, data annotation, crowdsourcing and ethical labeling. We call on the European Union (EU) to adopt theEU AI Act while highlighting the shortcomings of the current text with regard to the AI Supply Chain and data management.
β
β
Data labeling for AI
β
Data labeling is a key step in the development of AI. It involves assigning tags or labels todatasets, enabling machine learning algorithms to understand and interpret the information. However, it is imperative to ensure that this labeling is carried out with care, precision and under ethical conditions, in order to avoid bias and prejudice.
β
β
β
Ethical data annotation
β
Data annotation requires human expertise. It involves adding additional information to the data, i.e. a semantic layer associated with images, videos, etc. videos or text, such as metadata or detailed descriptions. In the context of AI, it is essential that data annotation is carried out ethically. This means that annotators (or Data Labelers) must follow strict guidelines to ensure the integrity and objectivity of annotated data, avoiding stereotyping, discrimination and value judgments. They must also work in good conditions (decent working hours, stability, career prospects) and be accompanied (training and support) to produce quality data.
β
β
β
The importance of crowdsourcing in labeling processes
β
Crowdsourcing is an effective method for labeling and annotating large quantities of data. By calling on a community of contributors, it is possible to obtain fast, accurate results. However, it is crucial to put in place rigorous quality control mechanisms to guarantee the reliability of the data produced by crowdsourcing . It's also important to remember that this is not the only way to label large quantities of data: it's often more effective to call on a panel of functional specialists to annotate data, and to accept their gradual increase in competence, rather than demanding an immediate maximum level of quality (which is often the case in crowdsourcing-based labeling processes). Data Labeling is an important job, and the people willing to invest themselves in it, the Data Labelers, must be treated with dignity and regarded as AI specialists in the same way as a Data Scientist.
β
β
β
Ethical labelling: a fundamental requirement
β
Ethical labeling is a fundamental aspect of responsible AI. It aims to ensure that the data used to train AI models is collected, labeled and annotated in an ethical and human-friendly way. Transparency and fairness are key principles of ethical labeling, helping to avoid bias and discrimination in automated decision-making.
β
β
β
Weaknesses of the EU AI Act: Supply Chain AI and data management
β
Despite the progress made by the EU AI Act project, it still has certain weaknesses with regard to the AI Supply Chain and data management. It is essential to put in place clear measures to ensure transparency and ethics throughout the lifecycle of AI systems, from data collection to use. Accountability and control mechanisms must be put in place to ensure proper data management and avoid abuse.
β
β
β
Data Labeling for ethical AI: conclusion
β
It is imperative that the European Union adopts sound ethical regulations to frame the development and use of AI. Regulation is necessary and must not hold back innovation. Data labeling and ethical sourcing are key to ensuring responsible AI and an AI data supply chain that respects human life and fundamental rights. However, it is also important to take into account the current weaknesses of the EU AI Act in terms of AI Supply Chain and data management, in order to strengthen the protection of these rights.