Harnessing clinical data and imaging in medicine: a real-world application of multimodal AI

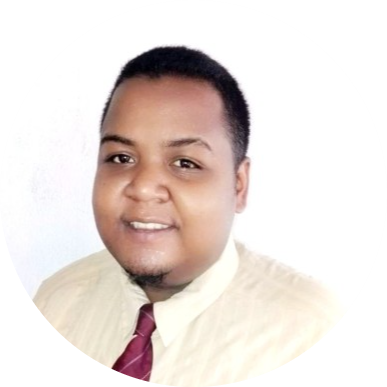
Artificial intelligence is making spectacular advances, andmultimodal AI stands out as a major innovation, particularly in the medical field. By combining different sources and types of data, such as medical images, clinical data and biological analyses, this technology offers an integrated and enriched vision of patients!
â
This approach overcomes the limitations of traditional analyses by exploiting the complementarity of data for a finer understanding of pathologies. On the rise, multimodal AI promises more effective precision medicine, where diagnoses and treatments can be personalized with unrivalled accuracy. In this article, we explain how multimodal AI works, and why it could well revolutionize medicine!
â
â
What is multimodal AI?
â
Multimodal AI refers to an advanced form of artificial intelligence capable of processing and interpreting several types of data from different, often heterogeneous, sources.
â
Unlike traditional AI systems that focus on one type of data (text, image or audio, for example), multimodal AI combines a variety of data, such as medical images, clinical reports, biological signals or genetic sequences. This integration enables a richer, more comprehensive understanding of information, particularly in complex contexts such as medicine.
â
â
How does Multimodal Artificial Intelligence work?
â
â
â
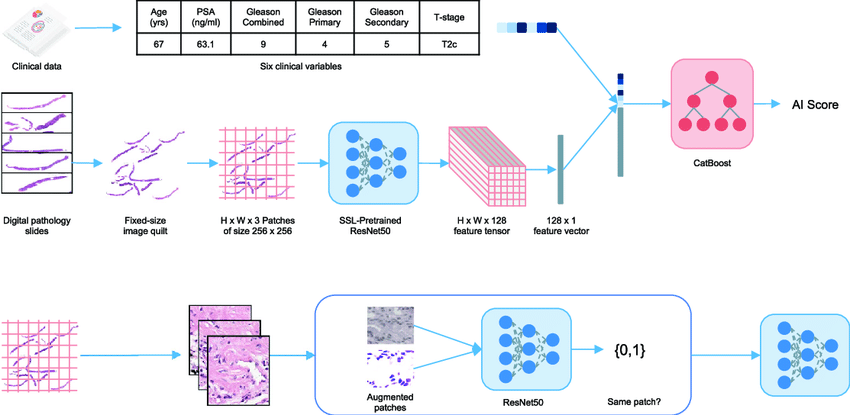
â
â
Multimodal AI relies on advanced algorithms, often based on DeepLearning neural networks, which are trained to interpret each type of data individually while learning to make connections between them. For example, a model can analyze an MRI image for abnormalities while taking into account associated clinical data, such as the patient's medical history or biological results. Thanks to this ability to establish correlations between distinct data sources, multimodal AI provides insights that conventional approaches, limited to a single modality, cannot offer.
â
This technology is based on key steps such as data fusion, which involves harmonizing the different modalities so that they are processed in a coherent way, and multimodal learning, which enables the model to capitalize on the complementary nature of the information. Multimodal AI thus offers a powerful approach to solving complex problems in fields where multidimensional understanding is essential, such as precision medicine.
â
â
How does multimodal AI improve medical diagnostics?
â
â
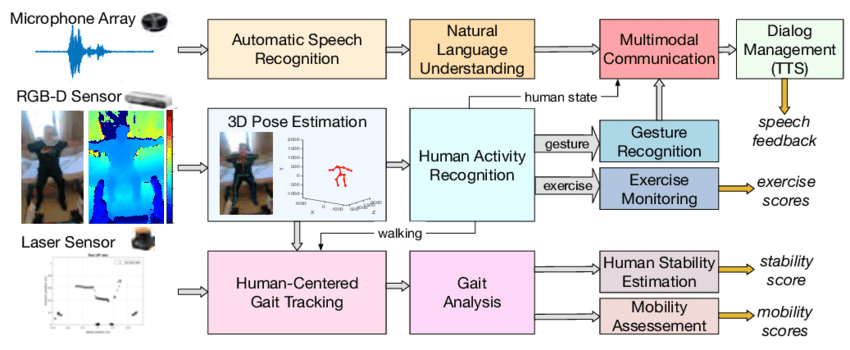
â
â
Multimodal AI improves medical diagnoses by exploiting the complementarity of data from different sources, enabling a more complete and accurate analysis of clinical cases. Unlike traditional methods, which often focus on a single type of data, such as an MRI image or a laboratory report, multimodal AI simultaneously integrates varied information, such as imaging data, electronic clinical records, biological results and even genetic history. This multidimensional approach enriches medical decision-making.
â
A global view for greater precision
By combining a variety of data, multimodal AI can detect subtle relationships between different types of information. For example, a model can identify correlations between a visible abnormality on an X-ray image and specific biomarkers present in blood results. This enhances diagnostic accuracy by reducing the risk of errors or isolated interpretations.
â
Early detection of disease
Thanks to its ability to analyze multiple signals simultaneously, multimodal AI excels in the early detection of disease. For example, in cancer screening, it can combine mammographic images with genetic data to more accurately assess risk and propose early detection, even at an asymptomatic stage.
â
Personalized care
Multimodal AI plays a key role in precision medicine, where treatments are tailored to each patient's unique characteristics. By integrating clinical and biological data specific to an individual, this technology can provide more tailored and effective treatment recommendations, improving clinical outcomes.
â
Shorter diagnosis times
Manual analysis of large quantities of medical data is often time-consuming and prone to human error. Multimodal AI automates these processes while maintaining consistency in data interpretation. This significantly reduces the time needed to make a diagnosis, which is particularly critical in emergency situations.
â
â
đĄ By integrating multidimensional data and exploiting their synergy, multimodal AI is redefining the way medical diagnoses are made. It contributes to more informed decision-making, faster disease detection and greater personalization of treatments, transforming healthcare in depth.
â
â
What types of data are used in multimodal AI in medicine?
â
Multimodal AI in medicine relies on a diversity of data from different sources to provide a comprehensive view of a patient's state of health. This data, which covers clinical, biological and environmental aspects, enables information to be cross-referenced for in-depth analysis and precise diagnosis. Here are the main types of data used
â
1. Medical imaging data
- X-rays, MRIs and CT scans: visualize the body's internal structures to identify abnormalities or lesions.
- Functional imaging (such as positron emission tomography): Provides information on tissue metabolic activity.
â
2. Clinical and demographic data
- Electronic medical records (EMR): Include medical history, diagnosis, treatment and allergies.
- Demographic information: Age, gender, weight, and other factors that influence health status and associated risks.
â
3. Biological results and laboratory analyses
- Biomarkers: Specific indicators, such as glucose levels, lipids or enzymes, that help assess pathologies.
- Genetic analysis: Data derived from DNA sequencing to identify genetic predispositions or specific mutations.
â
4. Text data
- Clinical reports: Notes written by doctors describing symptoms, diagnoses and recommendations.
- Radiology or pathology reports: Summaries of medical observations resulting from examinations.
â
5. Physiological signals
- ECG (electrocardiogram) data: Measures the heart's electrical activity.
- EEG (electroencephalogram) signals: Record electrical activity in the brain.
- Data on vital functions: heart rate, blood pressure, oxygen saturation, etc.
â
6. Environmental and behavioral data
- Physical activity tracking: Captured by wearable devices (such as connected watches).
- Environmental factors: exposure to pollution, air quality and climatic conditions.
â
7. Data from clinical trials
- Study protocol: Data detailing treatments administered and their observed effects.
- Clinical test results: Information collected on cohorts of patients to validate medical hypotheses.
â
â
đĄ En croisant ces donnĂ©es, lâIA multimodale permet de crĂ©er des modĂšles sophistiquĂ©s capables dâidentifier des patterns invisibles Ă lâĆil humain et de fournir des recommandations mĂ©dicales personnalisĂ©es. Ce mĂ©lange de donnĂ©es hĂ©tĂ©rogĂšnes est ce qui fait de lâIA multimodale un outil rĂ©volutionnaire dans le domaine mĂ©dical. Vous voulez en savoir plus sur l'annotation mĂ©dicale ? N'hĂ©sitez pas Ă nous contacter !
â
â
What are the current use cases for multimodal AI in healthcare?
â
Multimodal AI is currently transforming the healthcare field by tackling complex problems requiring the analysis of data from multiple sources. Here are the main current use cases that demonstrate its potential in medical practice:
â
1. Diagnosis of complex diseases
- Cancer: Multimodal AI combines radiological images, biopsies and genetic analysis to detect cancers at an early stage or assess their progression.
- Heart disease: Imaging data (cardiac ultrasound), electrocardiograms (ECGs) and clinical history enable us to identify cardiovascular risks with greater precision.
â
2. Precision medicine
By cross-referencing genomic, clinical and biological data, multimodal AI helps to personalize treatments according to the patient's specific characteristics. For example, it can recommend a drug treatment based on the patient's genetic profile and medical history.
â
3. Chronic disease management
- Diabetes: Multimodal models analyze blood glucose monitoring data, dietary habits and physical activity levels to help patients better manage their condition.
- Asthma: environmental sensors combined with clinical data can anticipate attacks by identifying triggers.
â
4. Assisted surgery
Multimodal AI systems provide surgeons with tools by combining pre-operative images (MRI, CT) with real-time data from sensors. This improves the precision of surgical interventions, particularly for complex operations such as those on the brain or heart.
â
5. Clinical research and therapeutic trials
Multimodal AI is used to analyze patient cohorts by integrating heterogeneous data from clinical trials, enabling the discovery of biomarkers or the identification of target populations for new treatments.
â
6. Early detection of epidemics
By combining clinical, demographic and environmental data, multimodal AI can anticipate epidemics by identifying symptom clusters in specific regions.
â
7. Analysis of rare diseases
Rare pathologies often require the combined analysis of a wide range of data. Multimodal AI helps reduce diagnosis time by cross-referencing genetic data, specific imaging, and medical history.
â
8. Post-treatment follow-up and rehabilitation
Data from connected devices, coupled with medical records, can be used to track patients' progress after treatment or surgery. This promotes personalized rehabilitation.
â
9. Training and decision support for healthcare professionals
Multimodal AI models serve as educational tools to train practitioners, simulating complex cases where different modalities need to be interpreted simultaneously.
â
10. Risk prevention and prediction
Multimodal platforms analyze medical history, lifestyle habits and environmental factors to predict the risk of diseases such as diabetes or heart disease, enabling targeted preventive interventions.
â
Conclusion
â
Multimodal AI represents a significant advance in the medical field, enabling the integration and exploitation of diverse data to transform the way healthcare is delivered.
â
By combining the analysis of images, clinical data and biological signals, this technology paves the way for more precise diagnostics, personalized treatments and truly precision medicine.
â
However, despite its promise, multimodal AI raises challenges, particularly in terms of ethics, data management and system interoperability. By overcoming these obstacles, it could become an essential pillar of connected healthcare and revolutionize medical practices, offering unprecedented prospects for patients and healthcare professionals alike.