Data Labeling Industry: crowdsourcing for AI, the only model?
.jpg)
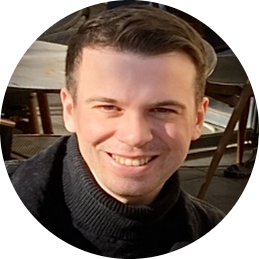
Using data annotation services: a necessity for anyone wanting to develop AI products?
β
Artificial intelligence (AI) has become an increasingly prominent topic of discussion in our society in recent years, highlighting the importance of ethical and responsible sourcing in the IT field. Recently, you've probably tested ChatGPT, from π OpenAIwhich blew your mind. However, according to the article π "AI Isn't Artificial or Intelligent" published by Vice, AI is neither artificial nor intelligent in the sense we usually understand it.
β
It has to be said that AI is actually a tool created by humans to accomplish specific tasks, often thanks to outsourcing and crowdsourcing in fields such as π Data Labeling. Its definition is that it has no consciousness or will of its own, and cannot be considered an "intelligent entity" in its own right. AI is simply programmed to follow the instructions it is given, and cannot think independently or make decisions independently. In short - it's a computer program like any other!
β
The impact of crowdsourcing in the AI industry is undeniable. This concept, which involves calling on a large community to solve problems or carry out tasks, is at the heart of many open innovation initiatives. Crowdsourcing makes it possible to gather ideas, knowledge and resources efficiently, drawing on the contributions of many individuals around the world.
β
Social and ethical issues in outsourcing image annotation tasks?
β
It's important to note that AI can also lead to social and ethical problems. For example, the automation of certain tasks can lead to the elimination of certain jobs, which can have consequences for workers and their way of life. It is therefore important to think about how AI can be used responsibly, fairly and ethically, in order to minimize potential risks to individuals and society. However, we must minimize what we sometimes hear about AI ("artificial intelligence will eliminate our jobs, tomorrow I'll be obsolete!"): with AI, jobs that don't exist today will emerge and create just as many opportunities all over the world.
β
AI can also have significant positive externalities, creating new opportunities in a variety of fields, including in developing countries. One of these positive externalities is the potential for job creation linked to AI (paradoxically). While certain tasks can be automated, new professions are emerging to design, develop, maintain and supervise AI systems. What's more, the massive data needed to feed AI algorithms can be collected, annotated and managed by human workers, creating jobs in data annotation and data quality management.
β
In developing countries, AI offers new economic opportunities. Companies can outsource AI tasks, such as π annotation of data or imagesto workers around the world, providing income opportunities for people with access to the Internet, even in remote areas. π This work shouldn't be seen as thankless : this is a bias of privileged countries, which perceive annotation tasks for AI as "micro-tasks", giving them little importance or credit in the AI development process. Yet it's a necessary job for the AI revolution, and one that few people in the world are prepared to do.
β
It is essential to ensure that these opportunities are equitably accessible, and that the benefits of AI are not concentrated solely in certain regions or among certain populations.
β
What's the difference between Data Labeling Outsourcing and Crowdsourcing?
β
What is Data Labeling?
β
As we've often said on this Blog, you've got it π Data Labeling is a critical process in the field of artificial intelligence (AI). It involves labeling data for use in an AI model. Crowdsourcing is increasingly used to produce such data labeling tasks in short timeframes. This is the dominant trend in the AI market, to produce data that can be exploited by models. If π some believe that Data Labeling is dead with LLMs (Large Language Models), the reality is more complex: try asking GPT-4 to draw a π Bounding Box on a very simple image, you might be surprised...
β
In short, what is crowdsourcing and how can it impact AI?
β
Why crowdsourcing for AI?
β
Crowdsourcing is not a new concept: it's a strategy for π data collection almost as old as the Internet, which involves relying on the contributions of many individuals to solve a problem or complete a task. This can be done online, via dedicated platforms, or using traditional methods such as surveys. Crowdsourcing has been widely popularized by platforms such as Wikipedia, which have enabled thousands of contributors to share their knowledge on a given subject.
β
Crowdsourcing is probably the best way to build an AI encyclopedia
β
The democratization of AI is comparable to the creation of a global encyclopedia through crowdsourcing. Just as Wikipedia revolutionized access to information, crowdsourcing in AI provides access to a diversity of data and perspectives essential to the development of inclusive and equitable technologies.
β
Crowdsourcing, as a key open innovation strategy, is essential for the development of AI products, and has proved particularly effective in the context of the continuous updating of algorithms and systems. The concept of crowdsourcing, by its very definition, invites a collaborative and distributed approach, making it ideal for projects requiring a wide diversity of data and perspectives.
β
β
β
β
β
β
Crowdsourcing can be an effective way of gathering ideas, knowledge and resources to accomplish tasks that would be difficult or costly to carry out traditionally. Applied to Artificial Intelligence, it involves gathering dozens or hundreds of π Data Labelersgenerally untrained and from low-income countries, and inviting them to work on a use case (for example, labeling 5,000 images of vehicles according to precise criteria). This approach has many negative aspects, with a social and ethical impact and precarious working conditions for many people. Here's an overview:
β
An exploitation of workers (Data Labelers or Data Labeling specialists)
β
One of the main problems with crowdsourcing is that it can lead to exploitation of workers, particularly in low-income countries.Some crowdsourcing platforms offer tasks to be carried out in exchange for remuneration, but this remuneration can be very low and does not reflect the real value of the work performed. There can be a real discrepancy between the work carried out by Data Labelers' teams and the low remuneration received. What's more, these platforms may not offer stability, social protection or rights to workers, which can lead to their situation becoming more precarious. While crowdsourcing can reduce costs and speed up production, it is essential to adopt an ethical and responsible approach, ensuring that workers are fairly remunerated and that their working conditions are dignified.
β
A negative impact on diversity and inclusion... and biased AI models
β
Crowdsourcing can also have a negative impact on diversity and inclusion. Indeed, some crowdsourcing platforms may be dominated by certain populations, which can lead to bias in the tasks offered and how they are completed. This can have negative consequences for marginalized or underrepresented populations, who may be excluded from these collaborative processes.
β
The diffusion of fake news
β
Finally, crowdsourcing can be misused to disseminate false information or dangerous ideologies. Indeed, the participation of a large number of people can give the impression that a consensus exists on a given subject, when in fact it may be false information or manipulation. This issue is particularly worrying in the current context, where the rapid spread of fake news can have serious consequences for people's lives, particularly in terms of health and safety.
β
Should we do without data annotation services for AI?
β
The answer is "no"! Even in the face of ethical and social challenges, it is vital torecognize the existence (and importance) of crowdsourcing in the AI product development process. Ethical and responsible solutions exist and must be explored to guarantee a respectful production chain, from sourcing data to feeding models with annotated data.
β
Data Labeling, although tedious, is essential to guarantee the effectiveness of AI. Mislabeled data can lead to erroneous results, underlining the importance of regular updating and careful verification of data. It is important that the Data Labeling process is carried out rigorously, ethically involving all workers in the AI product construction chain.
β
"We need to think seriously about the human workforce in the AI Supply Chain. This workforce deserves to be trained, supported and compensated to be ready to do important work that many might find tedious or too demanding."
β
Quote from Mary L. Gray and Siddharth Suri, authors of the book "Ghost Work: How to Stop Silicon Valley from Building a New Global Underclass," in a 2017 article in the Harvard Business Review.
β
What are the alternatives to crowdsourcing for AI? Why choose specialized service providers?
β
In the rapidly evolving world of artificial intelligence (AI), the quality of training data plays a key role in the success or failure of an AI model. The Data Labeling process, essential for preparing this data, requires precision and expertise that only specialized providers can offer. This is where the importance of partners like π CentaurLabswho specialize in medical annotation, become obvious.
β
Expertise at the heart of AI annotation
β
Data Labeling is much more than just an administrative task; it's an operation that requires an in-depth understanding of the field of application (medicine, finance, heavy industry, fashion, etc.). Specialized service providers bring not only technical expertise in data classification and labeling, but also in-depth knowledge of the sector concerned. In the case of medical annotation, for example, subtle nuances can make all the difference if the tool is used as a decision aid, for diagnosis.
β
CentaurLabs: a specialized model for medical annotation
β
CentaurLabs, a company specializing inmedical data annotation, is a perfect example of the importance of expertise in the field of Data Labeling. By harnessing the skills of medical professionals, CentaurLabs ensures that annotated data is not only accurate, but also relevant and reliable for medical AI applications. This accuracy is essential, as errors in annotated medical data can have direct consequences on patients' lives and health.
β
Why choose specialized service providers?
β
Data accuracy and quality:
Specialist providers guarantee high accuracy in data annotation, which is crucial to the performance of AI models. This is particularly important in sensitive fields such as medicine, where errors can have serious implications.
β
Time-saving :
By outsourcing Data Labeling to experts, companies save valuable time and effort that can be better invested in other aspects of their AI projects.
β
Compliance and ethics :
Specialist providers are often better equipped to navigate complex regulations and ethical considerations, especially in regulated areas such as healthcare.
β
Access to specific expertise:
Providers like CentaurLabs offer access to experts in specific fields, which improves the quality of annotations and, consequently, the performance of AI models.
β
Scalability and flexibility:
Specialized service providers can handle large volumes of data and adapt to changing project needs, giving companies great flexibility.
β
β
In conclusion, outsourcing Data Labeling work in a low-income country is a considerable responsibility: we are well aware of this at Innovatiana. We do our utmost to put people and ethics at the heart of your AI efforts! It is essential to ensure that Data Labelers are fairly remunerated and that processes are inclusive and do not disseminate false information or biased content.