Discover the FineWeb Dataset: Optimizing AI with superior data
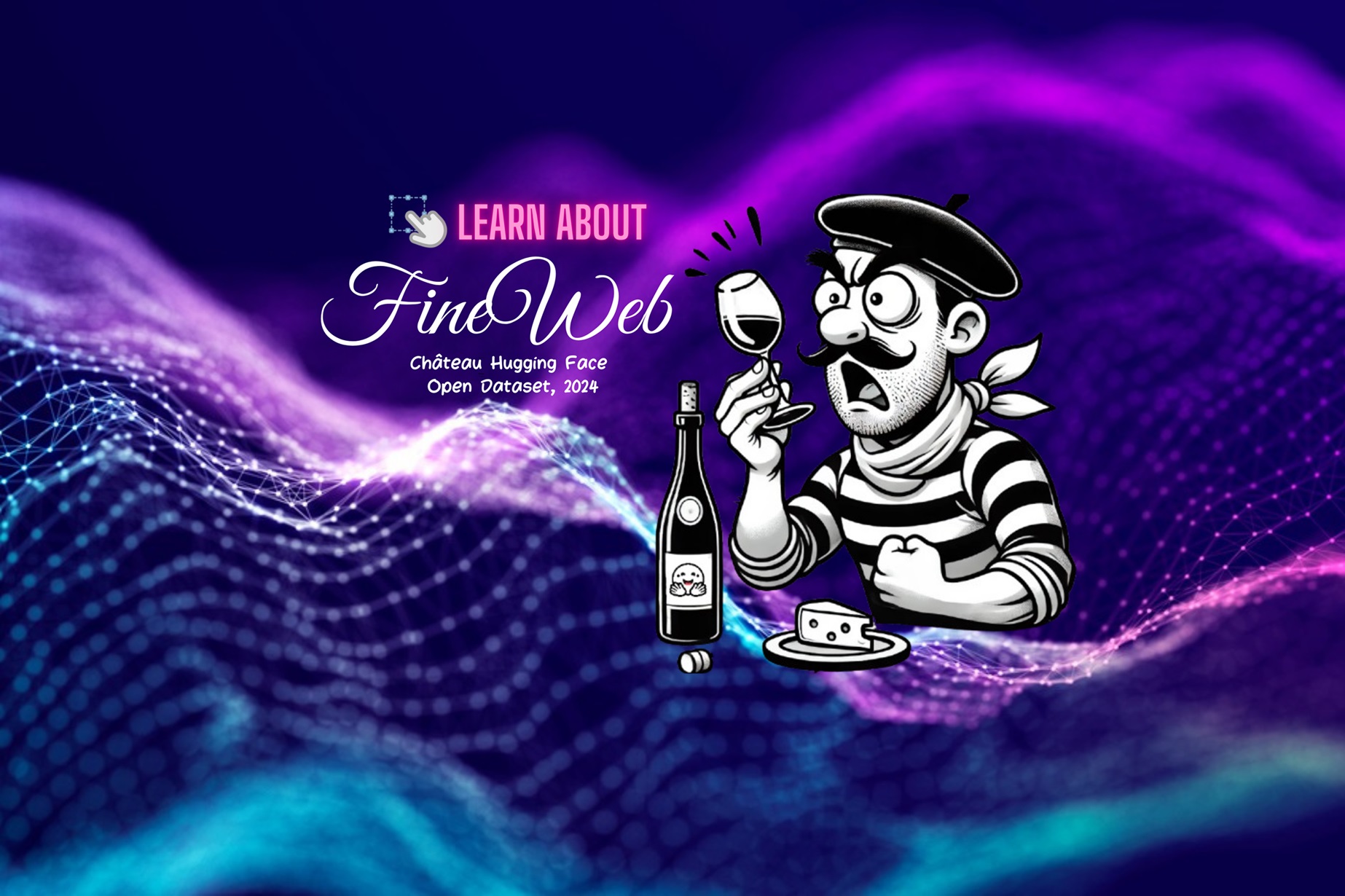
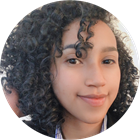
In artificial intelligence, data quality is a decisive factor in the performance of machine learning models. The FineWeb Dataset, developed by 🔗 Hugging Facerepresents a significant advance in this field.
Designed to enrich language models, this dataset is distinguished by its meticulous structure and substantial volume of prepared, sorted and annotated web data. By harnessing diverse, well-organized data, the FineWeb Dataset aims to improve the accuracy and efficiency of AI algorithms. Wondering why this dataset is important, and especially how it was constructed? We tell you more in this article!

What is the FineWeb Dataset and why is it important?
The FineWeb Dataset is a dataset developed by Hugging Face, designed to improve the training of Large Language Models (LLMs), LLM).
This dataset consists of data extracted from the Internet, carefully filtered and annotated to guarantee high quality and increased relevance for artificial intelligence applications. The collection of web pages and the importance of filtering URLs to avoid inappropriate content, personal or sensitive data, and ensuring effective deduplication at URL level are important aspects of maintaining data quality.
Its importance lies in its ability to provide diverse and accurate data, which is essential for the development of robust, high-performance AI models. By optimizing the quality of the data used for training, the FineWeb Dataset helps improve the accuracy, consistency and efficiency of language models. This makes it an invaluable resource for AI developers and enthusiasts working on applications requiring a fine understanding of natural language!
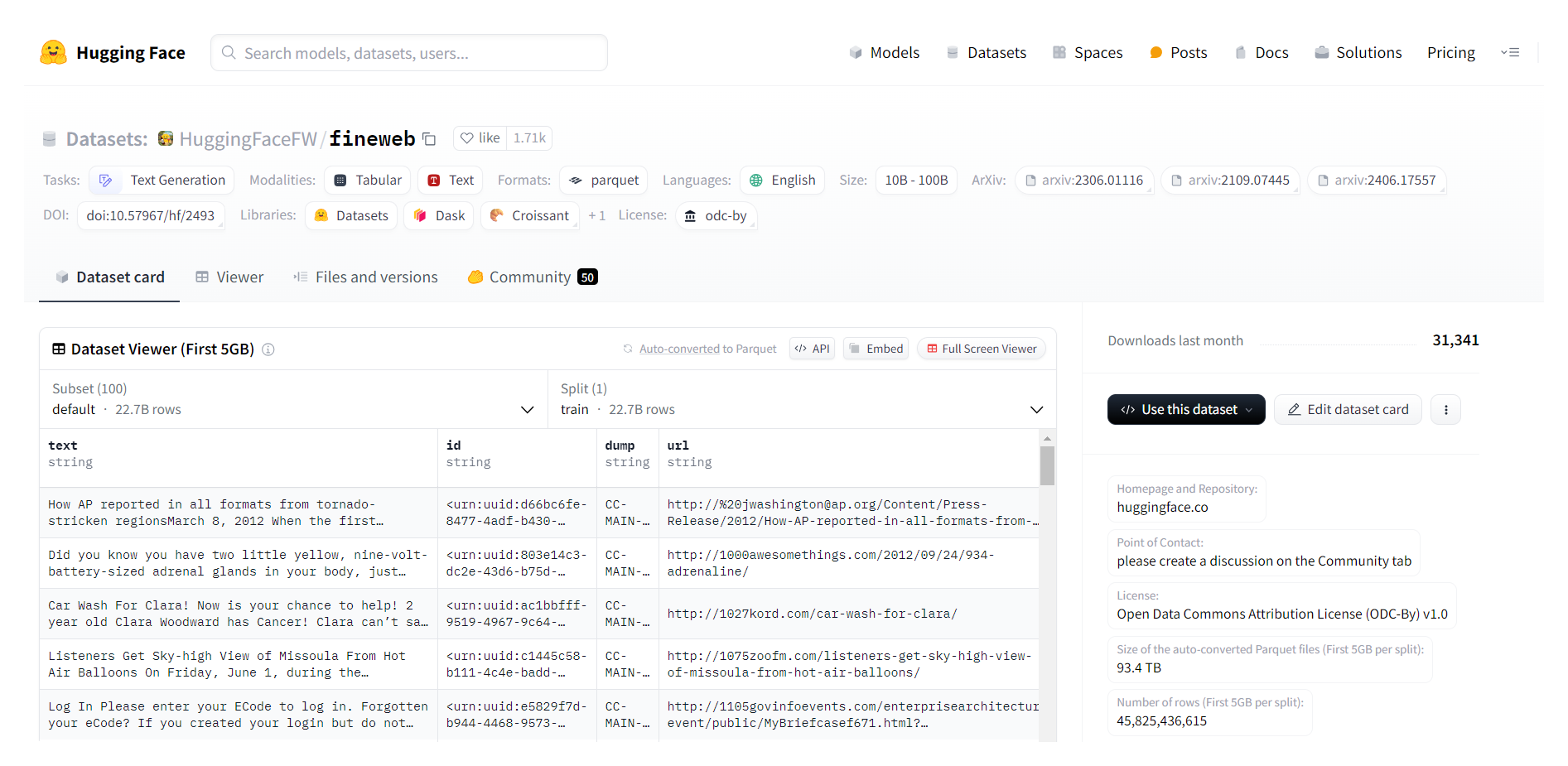
How does the FineWeb Dataset differ from other AI datasets?
The 🔗 FineWeb Dataset differs from other datasets for AI in several key respects:
1. Data quality
Unlike many datasets that contain raw, unfiltered data, the FineWeb Dataset is made up of carefully selected and annotated data to ensure high quality and maximum relevance. This selection process reduces noise and bias in the data, improving model performance.
2. Structure and diversity
The dataset is made up of a wide range of web data, covering different domains and types of content. This diversity enables language models to train on a variety of information, promoting better generalization and greater adaptability to complex tasks. What's more, the FineWeb Dataset contains millions of tokens, contributing to the diversity and richness of the data.
3. Continuous updating and maintenance
Hugging Face regularly updates the FineWeb Dataset to include new data and correct existing errors. This ongoing maintenance ensures that AI models remain up-to-date with the latest information and natural language trends.
4. Compatibility with large models (LLMs)
FineWeb Dataset has been specially designed to meet the needs of large-scale language models, optimizing data structure and format for easy integration into training processes.
5. Ethical approach and respect for privacy
In the current context of growing concerns about data confidentiality, FineWeb Dataset stands out for its adherence to ethical standards in the collection and use of web data, ensuring responsible use as part of the adoption of artificial intelligence tools and techniques.
These features make the FineWeb Dataset a unique and valuable resource for training artificial intelligence models, positioning it as a benchmark in the field of datasets for improving language models.
How does FineWeb EDU contribute to the training and improvement of artificial intelligence models?
A variant of FineWeb, the 🔗 FineWeb EDUcontributes to the training and improvement of artificial intelligence models by offering a dataset specifically designed for educational and research contexts. FineWeb EDU aims to transform the educational world by providing high-quality data for learning and research.
This version of the dataset aims to provide researchers, students and academic institutions with access to high-quality data, while being structured to facilitate learning and experimentation.
Here are some of the ways FineWeb EDU plays a key role in improving AI models:
1. Increased accessibility
FineWeb EDU is often made available for non-commercial or academic use, allowing researchers and students to explore and develop their own models without the financial or legal constraints that might be associated with other datasets.
2. Pre-processed data and quality annotations
The dataset includes rigorous, well-structured annotations, which are essential for accurate training of artificial intelligence models. These annotations enable models to learn from well-labeled data, reducing errors and improving the quality of predictions.
3. Encouraging innovation
By making data available to academic communities, FineWeb EDU encourages the development of new approaches and techniques for 🔗 natural language processing and machine learning. Researchers can experiment freely with these data, stimulating innovation and technological advances.
4. Update and adaptation
As with the standard FineWeb Dataset, the FineWeb EDU benefits from regular updates to include the latest relevant web data. This ensures that AI models trained with this data are based on the most up-to-date information and are able to respond to evolutions in natural language.
5.Practical training
By enabling users to experiment directly with real data, the FineWeb EDU helps to develop practical skills in using datasets, improving these datasets and, above all, modeling and optimizing the performance of AI models.
💡 Thanks to these features, FineWeb EDU plays a leading role in the education and development of artificial intelligence skills, while contributing to the continuous improvement of language models and research in the field of AI!
Is the FineWeb Dataset available as open source, and how does this impact AI research?
The FineWeb Dataset is largely available as Open Source, which means that its data is publicly accessible and can be used, modified and shared by the community. This open source approach offers maximum benefits for both the open source community and artificial intelligence research:
1. Open access and collaboration
The fact that the FineWeb Dataset is available as open source makes it easier for researchers, developers and academic institutions to collaborate. They can share their experiences, improvements and discoveries, accelerating innovation and the creation of new techniques in the field of natural language processing and machine learning.
2. Reducing barriers to entry
By being accessible to all, the FineWeb Dataset eliminates the costs often associated with acquiring high-quality data. This enables independent researchers, startups and universities to work on ambitious projects without the financial constraints, stimulating a diversity of contributions and perspectives in the field of AI. It's also crucial to share achievements and connect with experts on LinkedIn to enhance visibility and collaboration.
3. Transparency and reproducibility
The open source availability of the FineWeb Dataset promotes transparency in research processes. Thanks to the URLs included in the FineWeb Dataset, researchers can trace the origin of content and reproduce experiments carried out by other teams to validate results. This enhances the credibility and reliability of training studies for each AI model.
4. Continuous improvement of data
Open source allows the community to contribute to the continuous improvement of the dataset by reporting errors, adding new data or optimizing existing annotations. This active collaboration ensures that the FineWeb Dataset evolves and remains relevant to the changing needs of language models.
5. Rapid innovation
By making its data accessible, the FineWeb Dataset stimulates the rapid development of new AI architectures and techniques. Researchers can test and refine their models on a variety of data, leading to faster technological progress and more effective applications.
🪄 The impact of making a dataset like FineWeb available in Open Source is immense: it democratizes access to the resources needed to develop increasingly sophisticated models, while fostering a culture of sharing and collaboration within the scientific community!
Conclusion
The FineWeb Dataset represents a major advance in the field of artificial intelligence: it provides a solid basis for training language models, not only improving the accuracy and performance of algorithms, but also stimulating research and innovation within the scientific community. Its educational version, FineWeb EDU, further strengthens its impact by facilitating access to learning and experimentation for researchers and students.
Thanks to its features, the FineWeb Dataset is positioned as an essential resource for anyone aspiring to push the boundaries of what AI models can achieve. And if it's not enough for you, you can always contact us... our team of Data Labelers and data processing specialists can help you enrich this dataset, for example. 🔗 We look forward to hearing from you!