How can annotated datasets be evaluated to guarantee the reliability of AI models?
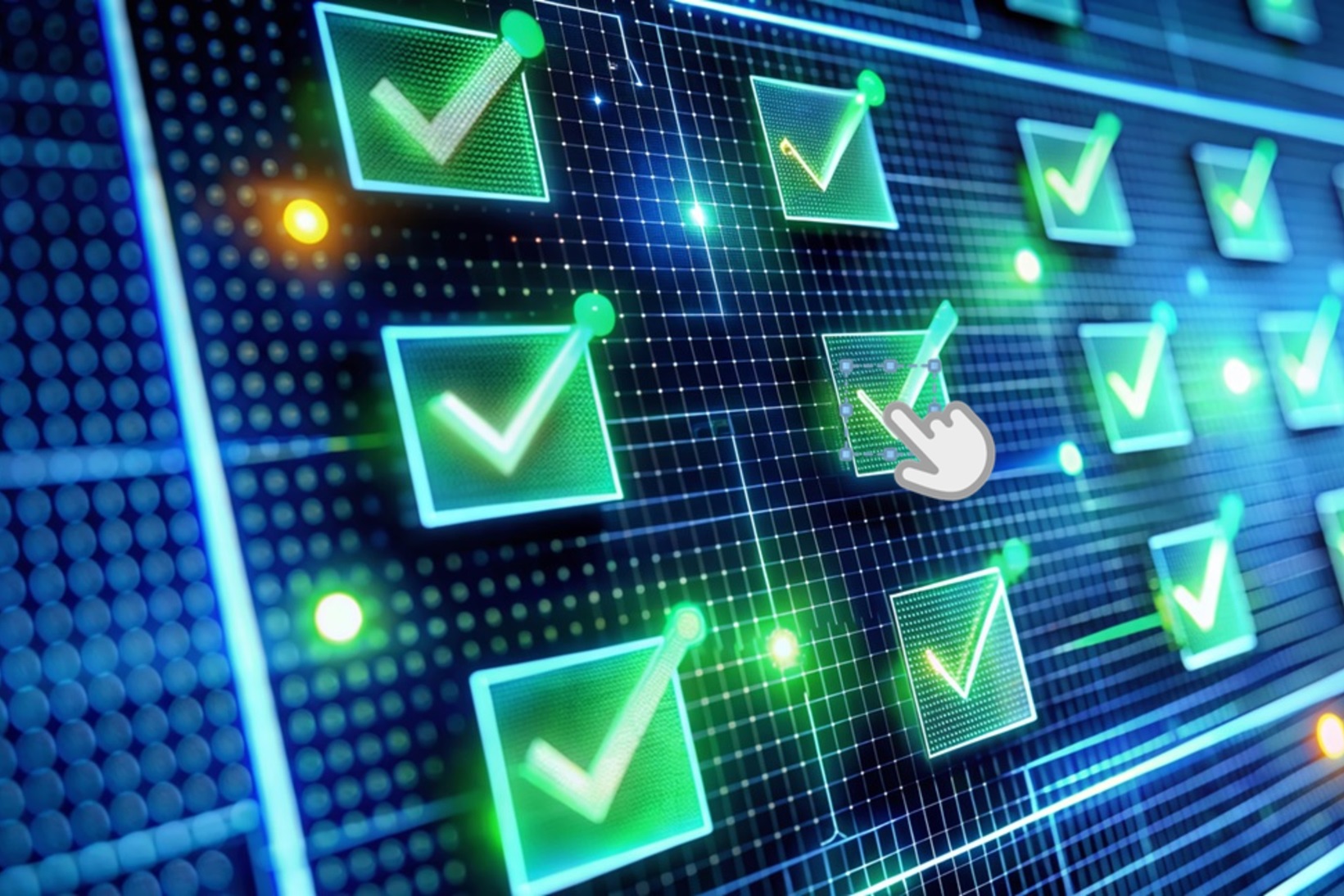
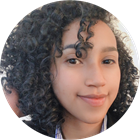
Assembling data sets is an essential element in the development of artificial intelligence systems. This involves assigning labels or tags to raw data to make it understandable and exploitable by Machine Learning models.
β
This is a very important step, as the quality of the annotations directly influences the performance and accuracy of the ai models. However, to guarantee data quality, it is essential to implement rigorous evaluations of data annotators, a critical process when training artificial intelligence models.
β
These evaluations ensure that annotations are carried out consistently and accurately, minimizing biases and errors that could compromise the effectiveness of artificial intelligence models.
β
β
What is the role of annotated data?
β
Fundamentals of data annotation
Datasets, and in particular annotated data, are the cornerstone of any artificial intelligence project. This involves the prior assignment of specific labels to various types of data, whether text, images or video, enabling machine learning models to recognize and interpret this information autonomously.
β
For example, in the context of image annotation, each visual element can be labeled to indicate its nature or function, such as identifying a person, object or action.
β
The types of annotation vary according to the needs of the project and the data to be processed. For text, this may include sentiment analysis, named entity labeling, or thematic categories. Image and video annotations may involve object segmentation, facial recognition or motion tracking.
β
Each of these annotations plays a key role in the training process of the models, enabling them to develop increasingly sophisticated classification, prediction and analysis capabilities.
β
Impact on the performance of artificial intelligence models
Accurate and consistent annotation is essential to guarantee the performance of Machine Learning models. Errors or inconsistencies in annotations can introduce bias or confusion into model learning, compromising their ability to generalize and provide reliable predictions.
β
High-quality annotations, on the other hand, improve not only the accuracy of models, but also their robustness and ability to adapt to new data. The impact of high-quality annotation on the performance of AI models cannot be underestimated, as data quality largely determines the model's ability to learn efficiently and produce accurate results.
β
β
β
β
β
β
β
β
β
Why is annotator evaluation important?
β
Avoid biases and errors
The evaluation of data annotators is essential to avoid biases and errors that can creep into the process of annotating data. Bias can take many forms, such as a tendency to interpret certain data subjectively, or to apply annotation criteria inconsistently.
β
These biases have a direct impact on artificial intelligence models, leading to biased or erroneous results that compromise data quality. Rigorous evaluation can detect these biases, adjust the instructions given to annotators, and ensure that annotations reflect an objective and uniform interpretation of the data.
β
Continuous improvement
In addition to preventing bias, annotator evaluation plays a key role in the continuous improvement of the annotation process. By identifying annotators' weak points, such as recurring errors or inconsistencies in the application of annotation criteria, it becomes possible to provide targeted training and make methodological adjustments.
β
This not only improves the quality of the annotations over time, but also enhances the overall consistency and accuracy of the dataset. As a result, artificial intelligence models based on these annotations become more robust, reliable, and capable of producing more accurate predictions.
β
β
What methodologies are used to evaluate annotators?
β
Inter-annotator agreement
One of the most commonly used methods for evaluating annotators is inter-annotator agreement. This method involves comparing the annotations produced by several annotators on the same dataset in order to measure their degree of agreement.
β
High agreement between annotators is an indicator of consistency, suggesting that annotation criteria are applied consistently. However, this method also has its drawbacks. High agreement can sometimes mask biases common to all annotators, while low agreement does not necessarily mean that annotations are incorrect, but may indicate legitimate differences of interpretation.
β
Despite these limitations, inter-annotator agreement remains a valuable tool for assessing consistency in the annotation process.
β
Testing the accuracy of annotated data
Accuracy tests are another key methodology in the evaluation of annotators. These tests are designed to measure an annotator's ability to correctly identify the elements to be annotated according to pre-established criteria.
β
Annotators are often evaluated on their ability to achieve a certain accuracy threshold, which is essential to ensure that annotations are both accurate and reliable. These tests are critical in identifying annotators whose performance may be inadequate, warranting additional training or revisions to the annotation process.
β
Other methods
In addition to inter-annotator agreement and accuracy testing, other evaluation methods are also employed. Sampling evaluation, for example, involves reviewing a subset of annotations to assess their quality, while continuous evaluation involves monitoring annotator performance over time for degradation or improvement.
β
These complementary approaches strengthen the overall assessment, ensuring constant supervision and enabling proactive adjustments to maintain a high level of quality in the annotations.
β
β
What role does human supervision play in automated assessment?
β
The efficiency of automated tools
With the advent of increasingly advanced annotation platforms and specialized systems, the evaluation of data annotators is becoming ever more sophisticated.
β
These platforms offer unrivalled speed and efficiency, making it possible to process large volumes of data and evaluate annotator performance in real time. These tools are capable, for example, of rapidly detecting inconsistencies, measuring agreement between annotators, and generating detailed evaluation reports.
β
Automation limits
However, these systems are often limited by their reliance on pre-programmed criteria and may lack nuance in interpreting complex or subjective annotations.
β
Automated tools, while powerful, are not always able to understand context or grasp the subtleties of data, which can lead to erroneous or incomplete assessments.
β
The added value of human supervision
This is where human control becomes critical. Human experts are able to understand specific contexts, recognize subtleties in the data, and make informed decisions about annotation quality.
β
Human intervention can compensate for the shortcomings of automated systems, providing a qualitative and contextual understanding that Machine Learning algorithms often miss.
β
Synergistic balance
The balance between automation and human supervision allows us to get the best out of both worlds. While automation ensures efficiency and consistency, human supervision ensures that evaluations take into account qualitative and contextual aspects.
β
This synergistic partnership is essential to maintain high standards in the evaluation of data annotators, while adapting to technological developments.
β
β
Case study: integrating an evaluation process into a data annotation workflow
β
Concrete illustration
In industry, several artificial intelligence projects have illustrated the impact of annotator evaluation on ultimate success. For example, a company specializing in the automatic detection of defects on production lines has adopted a rigorous evaluation process for its annotators.
β
This process included regular accuracy testing and the use of inter-annotator agreement to ensure that annotations were not only accurate, but also consistent across different annotators. This approach led to a significant improvement in annotation quality, which in turn improved the accuracy of the artificial intelligence models deployed.
β
Practical lessons
This case study highlights a number of practical lessons that can be applied to a variety of sectors. Firstly, the importance of a structured, ongoing evaluation process is proving to be a decisive factor in the success of artificial intelligence projects.
β
Companies need to integrate regular and rigorous evaluation mechanisms for their annotators, using methods such as inter-annotator agreement and accuracy testing.
β
In addition, ongoing training for annotators, based on the results of these assessments, ensures constant improvement in annotation quality.
β
These practices can be applied to a variety of fields, from image recognition to text analysis and video processing, to maximize the performance of AI models and guarantee reliable, accurate results.
β
β
What are the challenges in evaluating annotators?
β
Managing data complexity
One of the main challenges in evaluating annotators lies in the complexity of the data to be annotated. Complex data, such as images with multiple objects or ambiguous text, can make annotation more difficult and increase discrepancies between annotators.
β
This complexity poses a particular challenge during evaluation, as it becomes more difficult to determine whether differences in annotations are due to errors or legitimate interpretations. Evaluation in these contexts requires sophisticated methods to ensure that annotation quality is maintained, despite the complex nature of the data.
β
Maintain consistency and reduce bias
Maintaining consistency between different annotators while minimizing bias is another major challenge. Biases can arise due to cultural differences, personal experiences or simply subjective interpretation of annotation criteria.
β
These biases can affect the overall quality of annotations and, consequently, the performance of artificial intelligence models. It is essential to develop strategies to reduce these biases, such as standardization of annotation protocols and ongoing training of annotators, to ensure maximum consistency.
β
Technological innovations for assessment
To overcome these challenges, various technological solutions are emerging to facilitate the evaluation of data annotators. Artificial intelligence tools can now assist in evaluation by providing automated analyses of annotation quality, detecting inconsistencies and identifying potential biases.
β
These technologies not only streamline the evaluation process, but also make it more accurate and efficient. By integrating these tools, companies can improve the quality of their annotations while reducing the time and resources required to evaluate annotators.
β
β
What are the challenges of annotation quality?
β
Direct impact on AI model performance
The quality of data annotations is a key factor in the performance of artificial intelligence models. Poor-quality annotations can lead to misclassification errors, inaccurate predictions, and an overall reduction in model accuracy.
β
For example, in an image recognition model, incorrect or inconsistent annotations can lead the model to misidentify objects or people, compromising its reliability in critical applications such as security or medicine.
β
The direct consequence is a reduction in confidence in the results produced by the model, which can limit its use and adoption in real-life contexts.
β
Poor quality annotations can also affect the accuracy rate and the model's ability to maintain an accurate count of processed data, which is necessary for real-time applications.
β
Error propagation in AI models
Poor annotation quality is not limited to isolated errors; it can cause errors to propagate throughout the AI model. When the model learns from incorrectly annotated data, it incorporates these errors into its decision process, which can amplify biases and inaccuracies over time.
β
This propagation of errors can be particularly problematic in complex AI models that rely on successive layers of learning, where a small initial error can turn into a major problem, affecting the whole system (we could even speak of the butterfly effect in this specific example).
β
Consequences for interpretability and auditability
Poor-quality annotations can also complicate the interpretability and auditability of artificial intelligence models.
β
A model based on poorly annotated data can produce results that are difficult to explain or justify, posing challenges for regulators and auditors who need to ensure that the model complies with ethical and legal standards.
β
The opacity of decisions made by a poorly trained model can undermine corporate transparency and accountability, important elements for AI deployment in regulated sectors such as finance or healthcare.
β
Loss of trust and social acceptability
Beyond the technical implications, the quality of annotations directly influences public perception and the social acceptability of artificial intelligence technologies.
β
When AI models produce erroneous or biased results due to poor annotation, this can lead to widespread distrust of AI, slowing down its adoption and integration into society.
β
To maintain the confidence of users and the public, it is imperative that annotations are carried out with rigor and precision, thus guaranteeing AI models that are not only efficient, but also accurate and reliable.
β
β
What are the best strategies for minimizing errors?
β
Ongoing training for annotators
One of the most effective ways of reducing annotation errors is to invest in ongoing training for annotators.
β
By offering regular training sessions that update their skills and knowledge of annotation criteria, companies can ensure that annotators are well equipped to handle the challenges encountered during the annotation process.
β
This training can include workshops, simulations and case studies to help annotators better understand the nuances of the data they are processing.
β
Standardizing annotation protocols
Another way of minimizing errors is to standardize annotation protocols. By establishing clear, uniform guidelines for all annotators, we can reduce the inconsistencies and subjective interpretations that often lead to errors.
β
These protocols must be regularly reviewed and updated to adapt to changing data and project requirements.
β
Use of automated verification tools
Automated verification tools can also play an important role in reducing errors. These tools automatically detect inconsistencies or anomalies in annotations, offering a first line of defense against potential errors.
β
They can flag up suspicious annotations for human review, ensuring double-checking of critical data.
β
Setting up peer review processes
Peer review is another effective strategy for minimizing errors. By allowing annotators to review each other's work, we can not only detect errors more quickly, but also encourage a collaborative environment where annotators learn from each other.
β
This cross-review process enhances the consistency and accuracy of annotations, while offering professional development opportunities for annotators.
β
Regular feedback and adjustments
Finally, the implementation of a regular feedback system enables recurring errors to be identified and adjustments made in real time. Annotators must receive clear, constructive feedback on their work, accompanied by suggestions for improvement.
β
This continuous feedback helps prevent future errors and hone annotators' skills, leading to constant improvement in annotation quality.
β
β
What impact do annotations have on the ethics of AI?
β
The origin of ethical bias in annotations
Data annotation plays a central role in the development of artificial intelligence models, and its quality largely determines the precision and accuracy of these models' predictions. However, when annotations are biased, these biases propagate into AI models, affecting their ability to make ethical decisions.
β
Biases in annotations can arise from a variety of sources: subjective interpretations, unconscious biases on the part of annotators, or inadequate representation of data from a given population.
β
These biases can lead to models that reproduce and amplify existing inequalities, thus compromising the fairness and integrity of decisions made by AI.
β
Consequences of ethical biases on AI decisions
When an AI model is trained on biased data, it can make decisions that are morally or socially unacceptable. For example, a biased facial recognition model may be more likely to misidentify individuals of a certain ethnicity, leading to discrimination in critical contexts such as security or justice.
β
Similarly, in healthcare, a biased AI model could privilege certain groups of patients over others, exacerbating existing disparities in access to care. These examples illustrate how biases in annotations can have profound ethical implications, affecting the lives of individuals and society as a whole.
β
Measures to mitigate ethical bias
To minimize the impact of ethical biases, it is necessary to adopt rigorous practices during the data annotation process.
β
This includes training annotators to be aware of their own biases, implementing standardized protocols to reduce subjectivity, and using various automated tools to detect and correct potential biases in annotations.
β
What's more, human supervision is needed to regularly assess the fairness of the models, and to ensure that the decisions made by the AI meet strict ethical standards.
β
Collective responsibility for bias management
Ultimately, the responsibility for managing bias in annotations and ensuring the ethics of AI decisions lies with everyone involved in the development of these systems.
β
Companies, researchers and practitioners need to work together to identify and mitigate bias at every stage of the process, from data collection to model evaluation.
β
By adopting a proactive and transparent approach, it is possible to develop AI models that not only perform well, but also respect the principles of equity, justice and respect for human rights.
β
β
What does the future hold for the evaluation of data annotators?
β
Emerging trends in valuation
The assessment of data annotators is evolving rapidly, with emerging trends transforming the way this process is carried out. One of the most significant trends is automation, facilitated by the growing use of artificial intelligence.
β
Artificial intelligence systems can now monitor and evaluate annotator performance in real time, automatically detecting inconsistencies and errors. This automation not only reduces the time needed for evaluation, but also improves accuracy and consistency, eliminating much of the human bias.
β
Continuous innovation and improved practices
Innovation in assessment tools continues to progress, paving the way for ever more optimized practices in the industry. New technologies make it possible to refine assessment methods, enabling a more personalized and adaptable approach to specific project needs.
β
For example, machine learning systems can be used to identify annotators' individual weaknesses and offer targeted training. This continuous innovation promotes constant improvement in annotation quality.
β
What's more, it also encourages the adoption of industry-wide best practices, ensuring more reliable, high-performance artificial intelligence models.
β
β
Conclusion
β
The importance of evaluating data annotators cannot be underestimated, as has been demonstrated throughout this article. Methodologies such as inter-annotator agreement and accuracy testing are essential to ensure the quality and consistency of annotations, elements that are decisive for the performance of artificial intelligence models.
β
The challenges of data complexity and bias management also illustrate the need for rigorous, ongoing evaluation. As artificial intelligence continues to be integrated into new domains, the evaluation of annotators remains a central component in the development of reliable and effective models.
β
Evolving technologies, particularly automation and AI, offer opportunities to improve this process, but they don't replace the need for ongoing monitoring and constant improvement of annotation practices.
β
For companies and industry practitioners, adopting rigorous annotator evaluation practices is not only recommended, but essential to remain competitive in an environment where data quality determines the success of artificial intelligence projects.
β
By investing in structured assessment processes and taking advantage of emerging technologies, they can guarantee optimal results and contribute to the industry's ongoing progress.