Active Learning: a targeted, high-performance strategy for tagging data
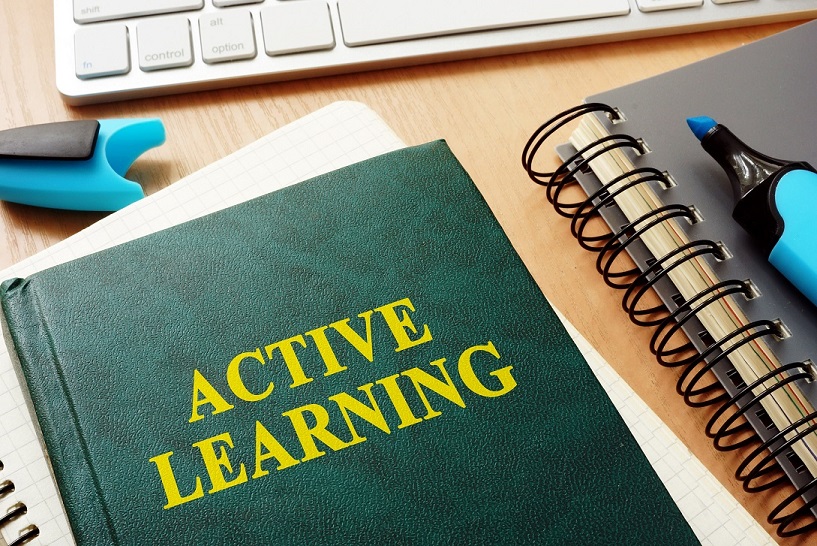
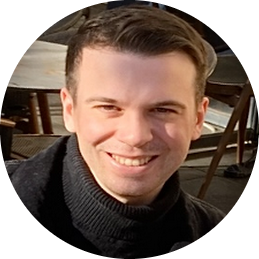
Active Learning: more than just a machine-learning approach.
Accelerate your AI projects by combining outsourcing to Madagascar with a targeted strategy to facilitate the work of annotators
Introduction
Artificial Intelligence (AI) has seen remarkable advances in recent years, particularly in the fields of Computer Vision and Natural Language Processing (NLP). However, the performance of these systems is highly dependent on the quality and quantity of the labeled data used to train them. The annotation of data, whether images or text, is a crucial step in providing this labeled data.
Active Learning, a targeted machine learning approach, can speed up the data annotation process, make annotators' work easier, and can be effectively combined with outsourcing data labelling work to Madagascar. In addition, many online image annotation tools incorporate Active Learning functionalities.
Active Learning: Optimizing data annotation
Active Learning is a machine learning strategy that aims to intelligently select the most informative and relevant samples for annotation as part of the data preparation required to train AI models. Instead of following a passive learning approach where data is labeled at random, Active Learning uses AI models under training to identify the most difficult or uncertain samples to classify. These samples are then submitted to human annotators for the addition of labels, gradually improving the model's performance, while providing annotators with hints or tips on how to label the data sets entrusted to them more effectively.
Data Labeling outsourcing in Madagascar
Madagascar has rapidly established itself as a preferred destination for data labeling outsourcing, for a number of reasons. Firstly, the country offers competitive labor costs, making outsourcing more economical. In addition, Madagascar has a multilingual population, making it a wise choice for text annotation projects in different languages. In addition, the availability of technical skills in the field of IT and related sciences makes it easy to find qualified annotators. Finally, the country's political stability creates a favorable environment for foreign investment, enhancing its attractiveness for outsourcing data labeling.
Image and text annotation
Image and text annotation is essential for training AI models for a variety of tasks.
Image annotation
In the field of Computer Vision, image annotation consists of adding labels or tags to objects and elements present in an image. For example, for object detection, bounding boxes can be drawn around objects of interest.
Text annotation
In NLP ("Natural Language Processing"), text annotation involves identifying and labeling specific parts of a text. This can include the detection of named entities, the categorization of sentiments or the classification of topics.
Annotators: key players in the effective use of Active Learning
Annotators, also known as "Data Labelers", play a central role in the Active Learning process. Their expertise in understanding specific instructions and applying strict labeling standards guarantees the quality of annotated data.
Close collaboration between data scientists, developers and annotators is essential to adapt the annotation process to the changing needs of the model being learned.
Online image and text annotation tools with embedded Active Learning functionalities
Several online image annotation platforms now offer Active Learning capabilities, simplifying the annotation process for data labelers.
For example, UBI AI is a platform offering online image annotation tools with Active Learning functionalities for NLP projects. These tools enable annotators to intelligently select the most informative and uncertain samples, improving the efficiency of the annotation process.
In addition to UBI AI, other examples of platforms that include Active Learning for online image annotation are XYZ Annotation Tool and Labelbox. Like most annotation platforms today, these tools provide a user-friendly interface for tagging images with various types of labels, such as keypoints, bounding boxes and object segments.
Conclusion
Active Learning is a powerful approach to accelerating the data annotation process in the field of Artificial Intelligence. By outsourcing data labeling to Madagascar, companies benefit from competitive costs, available technical skills, and a qualified multilingual population.
Thanks to online annotators and image annotation tools with embedded Active Learning functionalities, AI projects can reach new levels of accuracy and performance, taking the AI development process to new heights.